Semantic Fisher Scores for Task Transfer: Using Objects to Classify Scenes
IEEE Transactions on Pattern Analysis and Machine Intelligence(2020)
摘要
The transfer of a neural network (CNN) trained to recognize objects to the task of scene classification is considered. A Bag-of-Semantics (BoS) representation is first induced, by feeding scene image patches to the object CNN, and representing the scene image by the ensuing bag of posterior class probability vectors (semantic posteriors). The encoding of the BoS with a Fisher vector (FV) is then studied. A link is established between the FVof any probabilistic model and the 0-function of the expectation-maximization (EM) algorithm used to estimate its parameters by maximum likelihood. This enables 1) immediate derivation of FVs for any model for which an EM algorithm exists, and 2) leveraging efficient implementations from the EM literature for the computation of FVs. It is then shown that standard FVs, such as those derived from Gaussian or even Dirichlet mixtures, are unsuccessful for the transfer of semantic posteriors, due to the highly non-linear nature of the probability simplex. The analysis of these FVs shows that significant benefits can ensue by 1) designing FVs in the natural parameter space of the multinomial distribution, and 2) adopting sophisticated probabilistic models of semantic feature covariance. The combination of these two insights leads to the encoding of the BoS in the natural parameter space of the multinomial, using a vector of Fisher scores derived from a mixture of factor analyzers (MFA). A network implementation of the MFA Fisher Score (MFA-FS), denoted as the MFAFSNet, is finally proposed to enable end-to-end training. Experiments with various object CNNs and datasets show that the approach has state-of-the-art transfer performance. Somewhat surprisingly, the scene classification results are superior to those of a CNN explicitly trained for scene classification, using a large scene dataset (Places). This suggests that holistic analysis is insufficient for scene classification. The modeling of local object semantics appears to be at least equally important. The two approaches are also shown to be strongly complementary, leading to very large scene classification gains when combined, and outperforming all previous scene classification approaches by a sizable margin.
更多查看译文
关键词
Deep neural network,scene classification,fisher vector,MFA
AI 理解论文
溯源树
样例
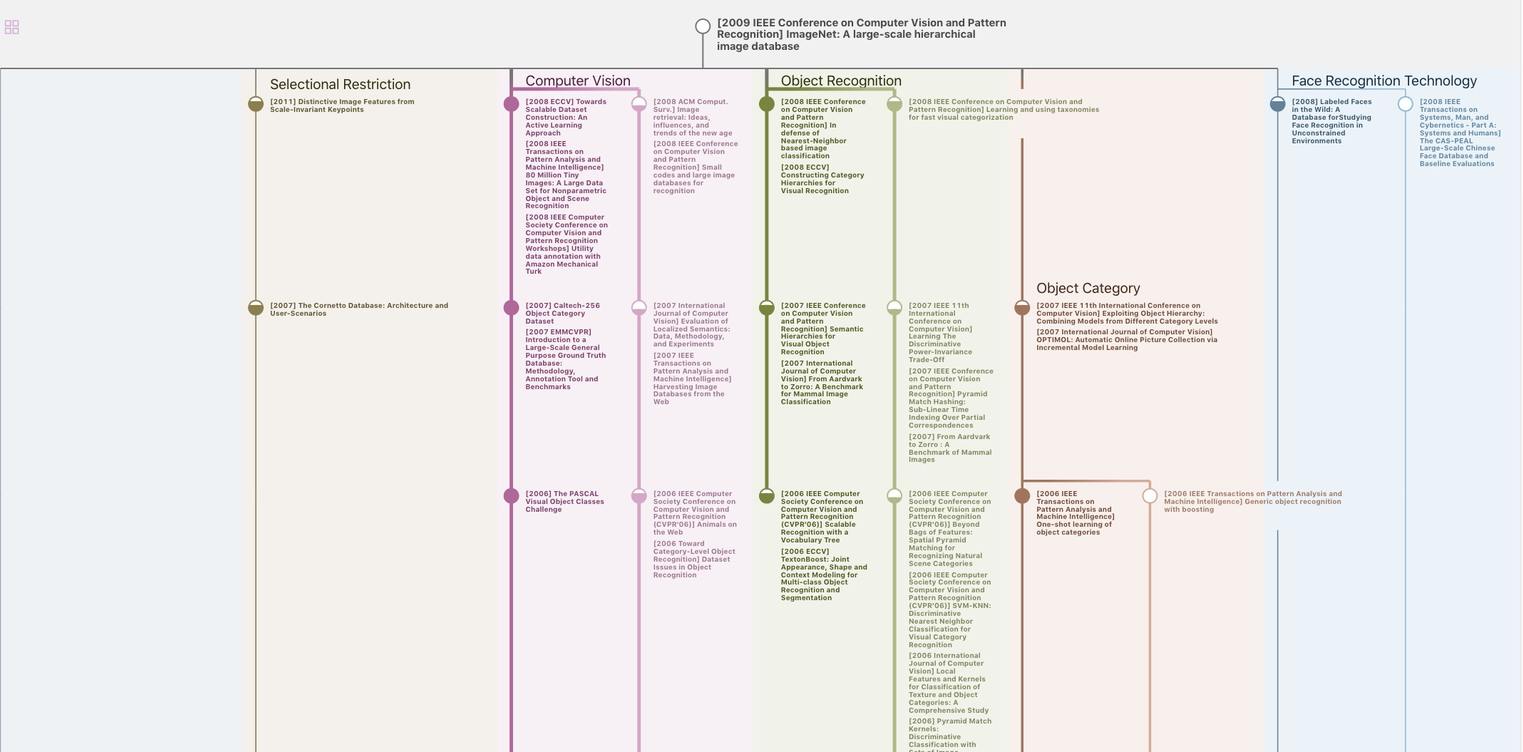
生成溯源树,研究论文发展脉络
Chat Paper
正在生成论文摘要