Finite-sample analysis of nonlinear stochastic approximation with applications in reinforcement learning
Automatica(2022)
摘要
Motivated by applications in reinforcement learning (RL), we study a nonlinear stochastic approximation (SA) algorithm under Markovian noise, and establish its finite-sample convergence bounds under various stepsizes. Specifically, we show that when using constant stepsize (i.e., αk≡α), the algorithm achieves exponential fast convergence to a neighborhood (with radius O(αlog(1/α))) around the desired limit point. When using diminishing stepsizes with appropriate decay rate, the algorithm converges with rate O(log(k)/k). Our proof is based on Lyapunov drift arguments, and to handle the Markovian noise, we exploit the fast mixing of the underlying Markov chain. To demonstrate the generality of our theoretical results on Markovian SA, we use it to derive the finite-sample bounds of the popular Q-learning algorithm with linear function approximation, under a condition on the behavior policy. Importantly, we do not need to make the assumption that the samples are i.i.d., and do not require an artificial projection step in the algorithm. Numerical simulations corroborate our theoretical results.
更多查看译文
关键词
Markovian stochastic approximation,Finite-sample analysis,Reinforcement learning,Q-learning,Linear function approximation
AI 理解论文
溯源树
样例
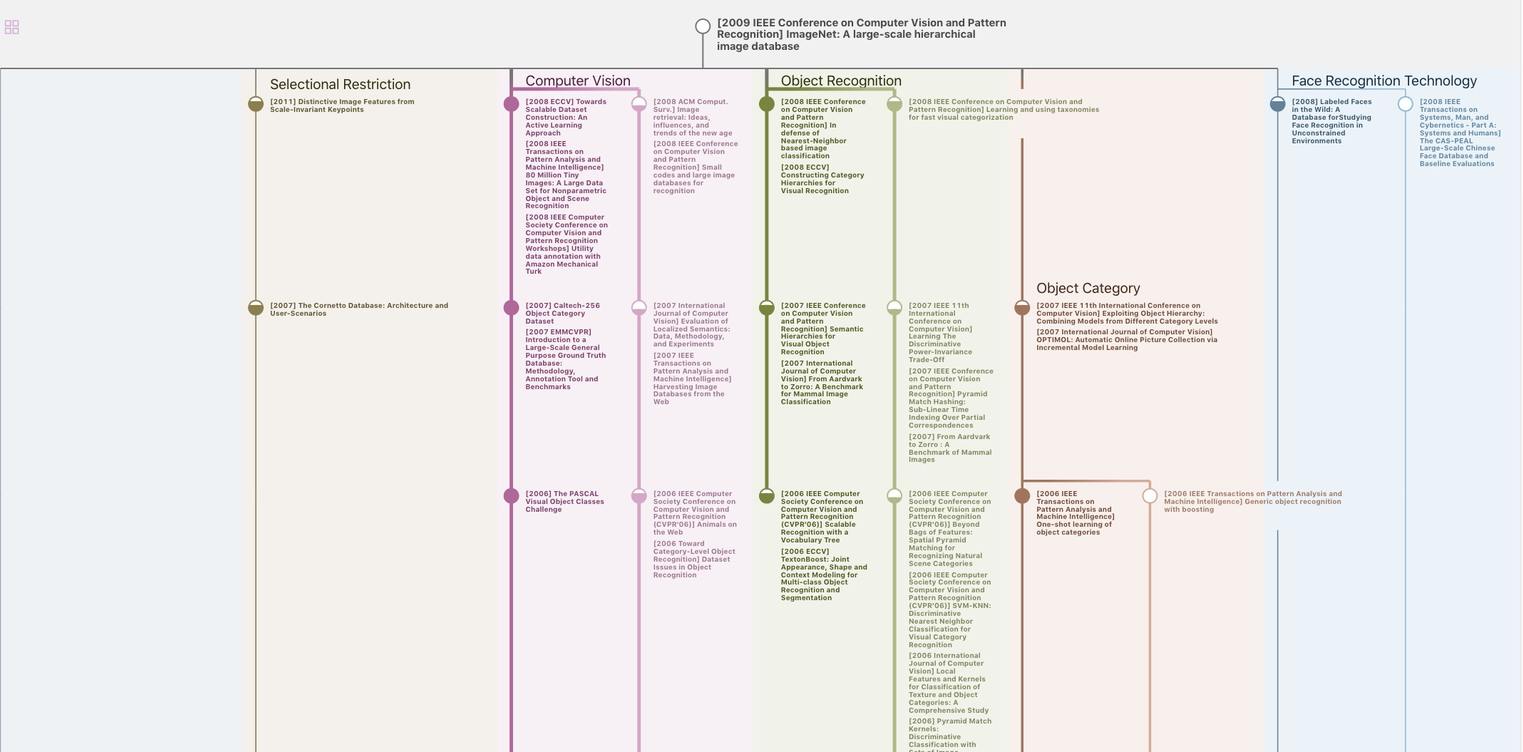
生成溯源树,研究论文发展脉络
Chat Paper
正在生成论文摘要