Using Pre-Training Can Improve Model Robustness and Uncertainty
INTERNATIONAL CONFERENCE ON MACHINE LEARNING, VOL 97(2019)
摘要
He et al. (2018) have called into question the utility of pre-training by showing that training from scratch can often yield similar performance to pre-training We show that although pre-training may not improve performance on traditional classification metrics, it improves model robustness and uncertainty estimates. Through extensive experiments on label corruption, class imbalance, adversarial examples, out-of-distribution detection, and confidence calibration, we demonstrate large gains from pre-training and complementary effects with task-specific methods. We show approximately a 10% absolute improvement over the previous state-of-the-art in adversarial robustness. In some cases, using pre-training without task-specific methods also surpasses the state-of-the-art, highlighting the need for pre-training when evaluating future methods on robustness and uncertainty tasks.
更多查看译文
AI 理解论文
溯源树
样例
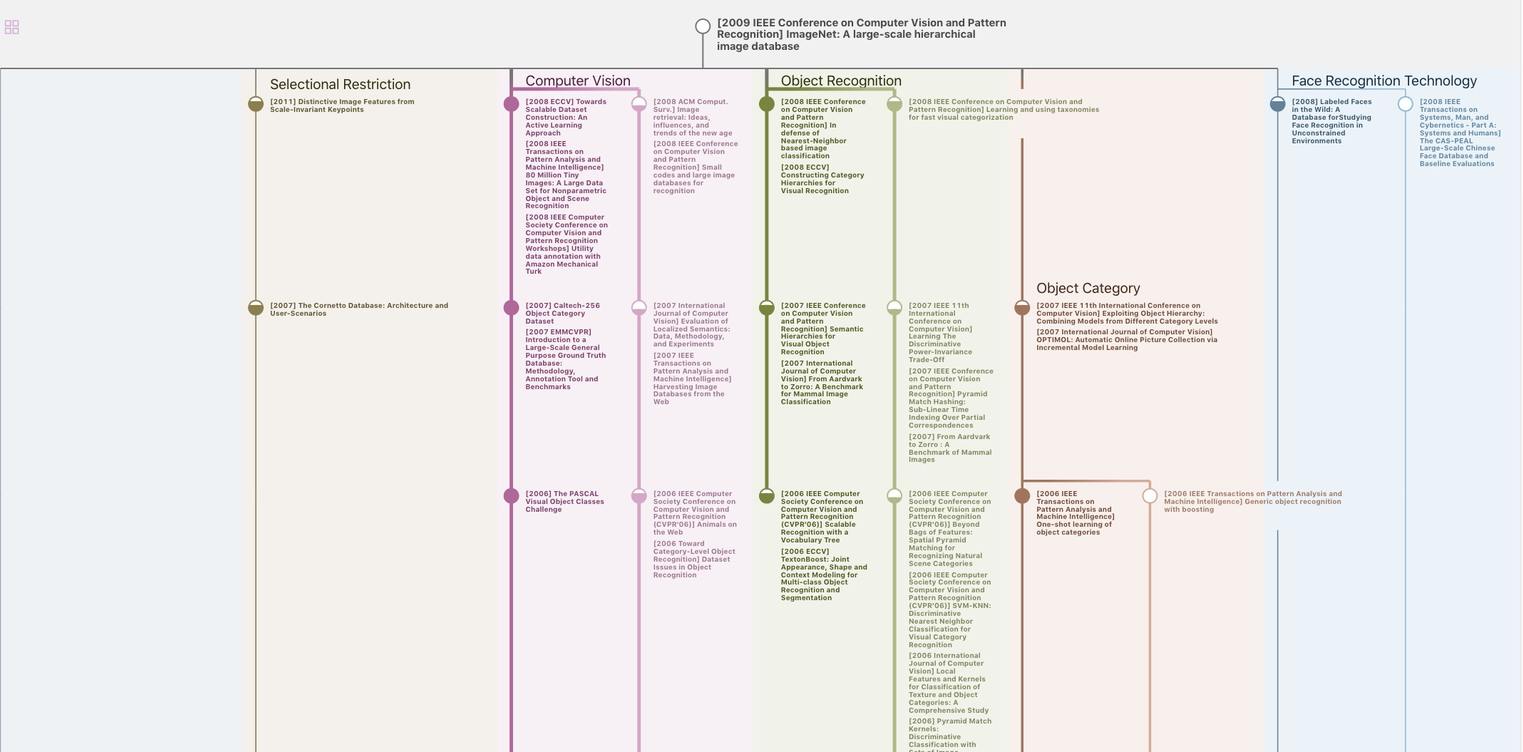
生成溯源树,研究论文发展脉络
Chat Paper
正在生成论文摘要