A Geometric Perspective on Optimal Representations for Reinforcement Learning.
ADVANCES IN NEURAL INFORMATION PROCESSING SYSTEMS 32 (NIPS 2019)(2019)
摘要
We propose a new perspective on representation learning in reinforcement learning based on geometric properties of the space of value functions. We leverage this perspective to provide formal evidence regarding the usefulness of value functions as auxiliary tasks. Our formulation considers adapting the representation to minimize the (linear) approximation of the value function of all stationary policies for a given environment. We show that this optimization reduces to making accurate predictions regarding a special class of value functions which we call adversarial value functions (AVFs). We demonstrate that using value functions as auxiliary tasks corresponds to an expected-error relaxation of our formulation, with AVFs a natural candidate, and identify a close relationship with proto-value functions (Mahadevan, 2005). We highlight characteristics of AVFs and their usefulness as auxiliary tasks in a series of experiments on the four-room domain.
更多查看译文
关键词
reinforcement learning,geometric perspective,close relationship
AI 理解论文
溯源树
样例
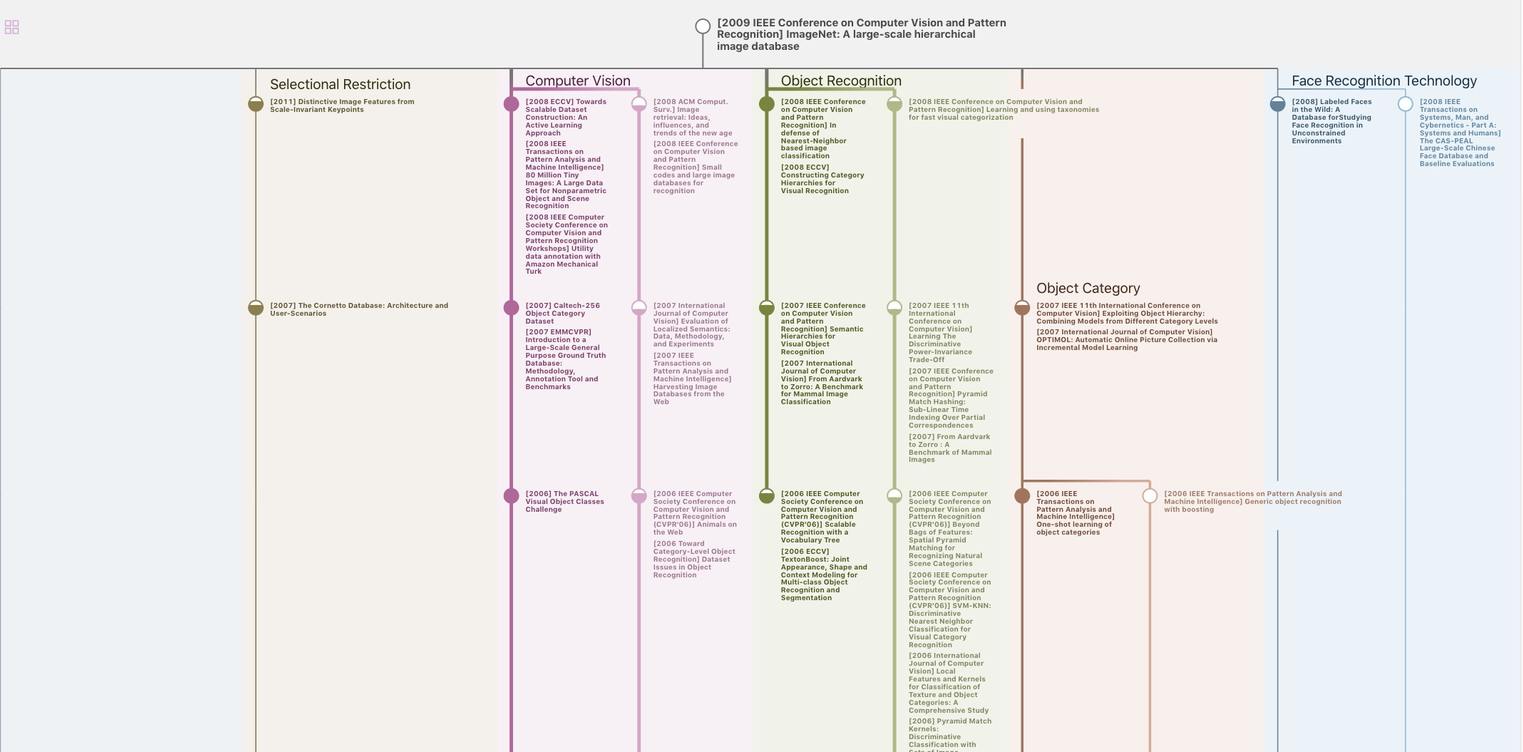
生成溯源树,研究论文发展脉络
Chat Paper
正在生成论文摘要