Multi-Instance Learning for End-to-End Knowledge Base Question Answering.
arXiv: Computation and Language(2019)
摘要
End-to-end training has been a popular approach for knowledge base question answering (KBQA). However, real world applications often contain answers of varied quality for usersu0027 questions. It is not appropriate to treat all available answers of a user question equally. This paper proposes a novel approach based on multiple instance learning to address the problem of noisy answers by exploring consensus among answers to the same question in training end-to-end KBQA models. In particular, the QA pairs are organized into bags with dynamic instance selection and different options of instance weighting. Curriculum learning is utilized to select instance bags during training. On the public CQA dataset, the new method significantly improves both entity accuracy and the Rouge-L score over a state-of-the-art end-to-end KBQA baseline.
更多查看译文
AI 理解论文
溯源树
样例
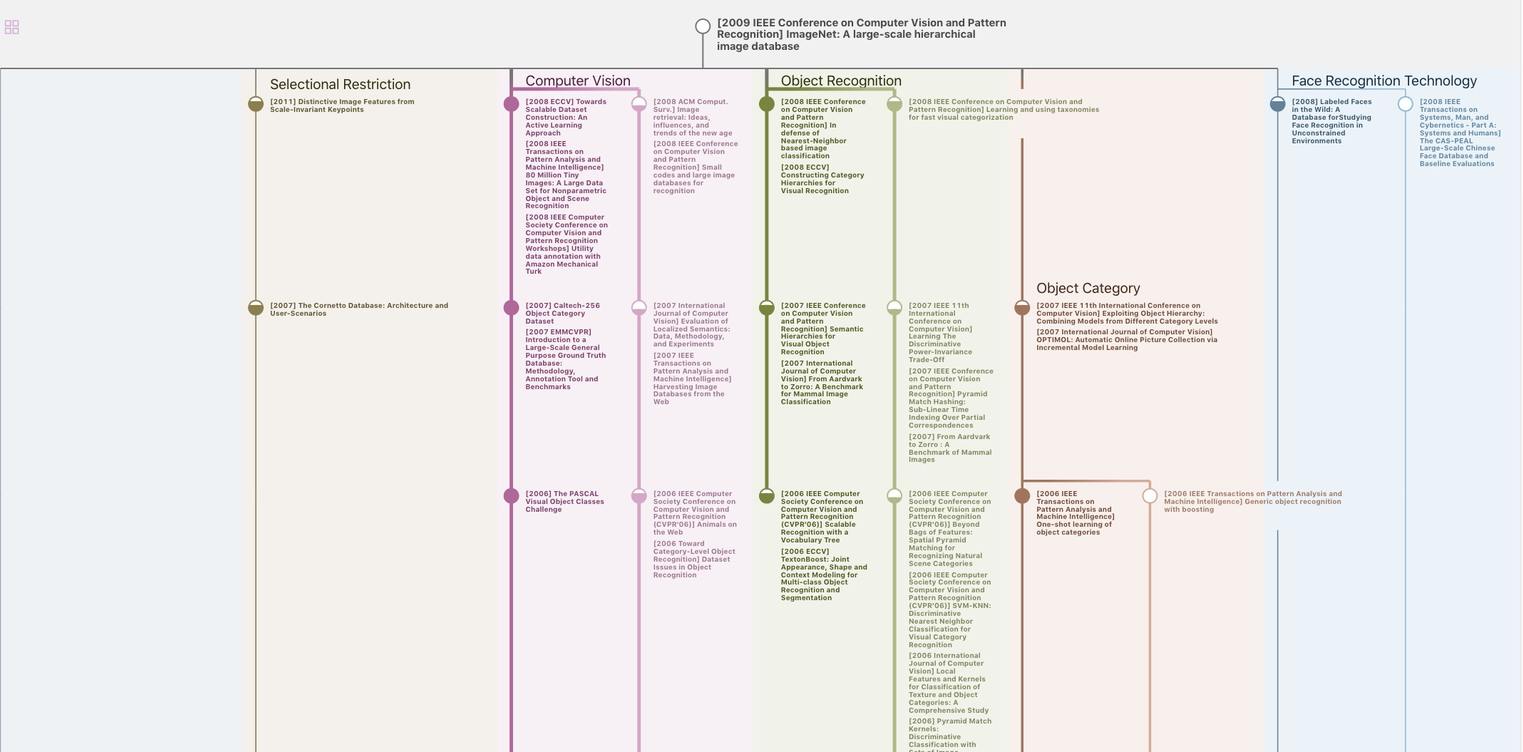
生成溯源树,研究论文发展脉络
Chat Paper
正在生成论文摘要