TensorFlow Doing HPC
2019 IEEE International Parallel and Distributed Processing Symposium Workshops (IPDPSW)(2019)
摘要
TensorFlow is a popular emerging open-source programming framework supporting the execution of distributed applications on heterogeneous hardware. While TensorFlow has been initially designed for developing Machine Learning (ML) applications, in fact TensorFlow aims at supporting the development of a much broader range of application kinds that are outside the ML domain and can possibly include HPC applications. However, very few experiments have been conducted to evaluate TensorFlow performance when running HPC workloads on supercomputers. This work addresses this lack by designing four traditional HPC benchmark applications: STREAM, matrix-matrix multiply, Conjugate Gradient (CG) solver and Fast Fourier Transform (FFT). We analyze their performance on two supercomputers with accelerators and evaluate the potential of TensorFlow for developing HPC applications. Our tests show that TensorFlow can fully take advantage of high performance networks and accelerators on supercomputers. Running our TensorFlow STREAM benchmark, we obtain over 50% of theoretical communication bandwidth on our testing platform. We find an approximately 2×, 1.7× and 1.8× performance improvement when increasing the number of GPUs from two to four in the matrix-matrix multiply, CG and FFT applications respectively. All our performance results demonstrate that TensorFlow has high potential of emerging also as HPC programming framework for heterogeneous supercomputers.
更多查看译文
关键词
TensorFlow,Emerging Programming Environment,Parallel Computing,Heterogeneous Supercomputers,HPC Applications
AI 理解论文
溯源树
样例
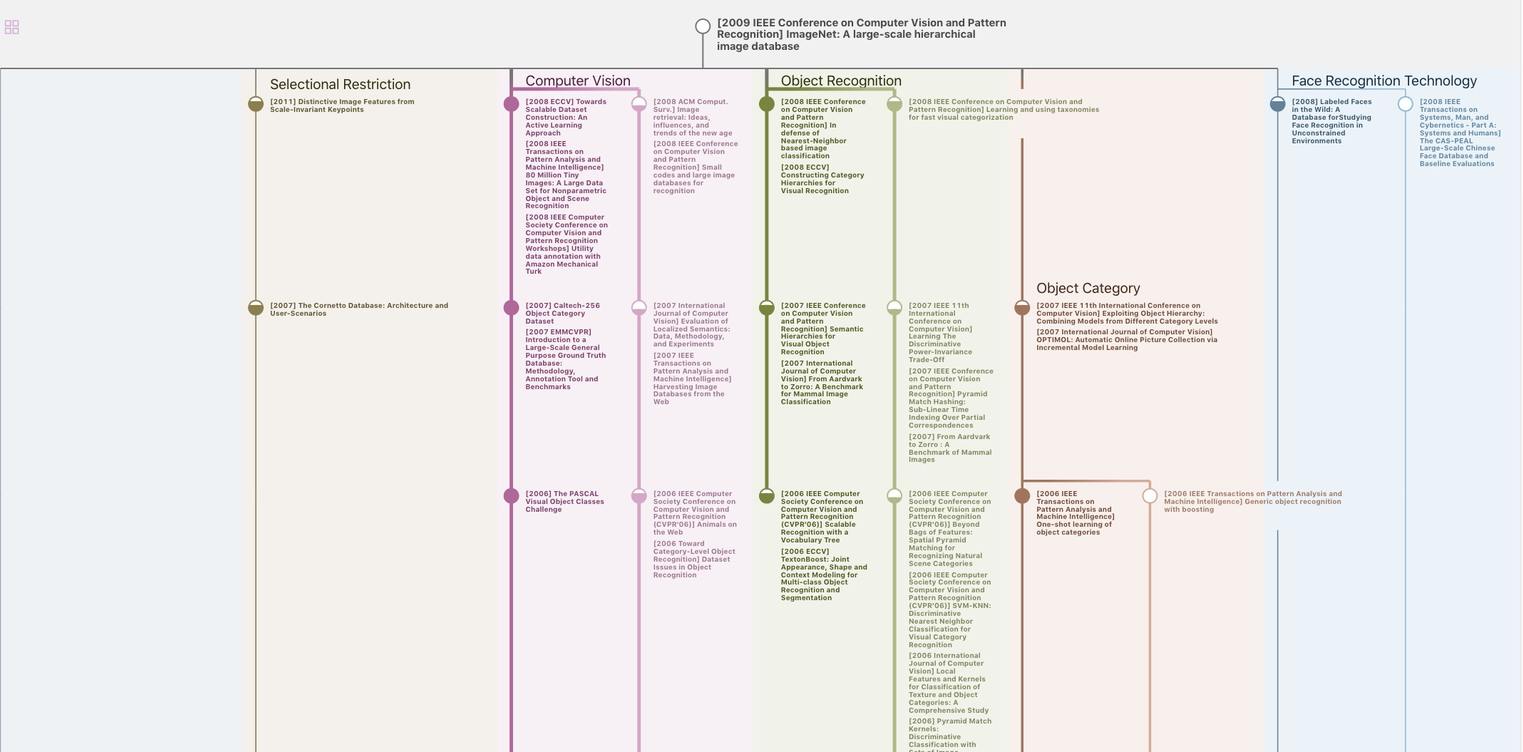
生成溯源树,研究论文发展脉络
Chat Paper
正在生成论文摘要