Empirically accelerating scaled gradient projection using deep neural network for inverse problems in image processing
ICASSP 2021 - 2021 IEEE International Conference on Acoustics, Speech and Signal Processing (ICASSP)(2021)
摘要
Recently, deep neural networks (DNNs) have shown advantages in accelerating optimization algorithms. One approach is to unfold finite number of iterations of conventional optimization algorithms and to learn parameters in the algorithms. However, these are forward methods and are indeed neither iterative nor convergent. Here, we present a novel DNN-based convergent iterative algorithm that accelerates conventional optimization algorithms. We train a DNN to yield parameters in scaled gradient projection method. So far, these parameters have been chosen heuristically, but have shown to be crucial for good empirical performance. In simulation results, the proposed method significantly improves the empirical convergence rate over conventional optimization methods for various large-scale inverse problems in image processing.
更多查看译文
关键词
scaled gradient projection,deep neural network,iterative algorithm,convergence
AI 理解论文
溯源树
样例
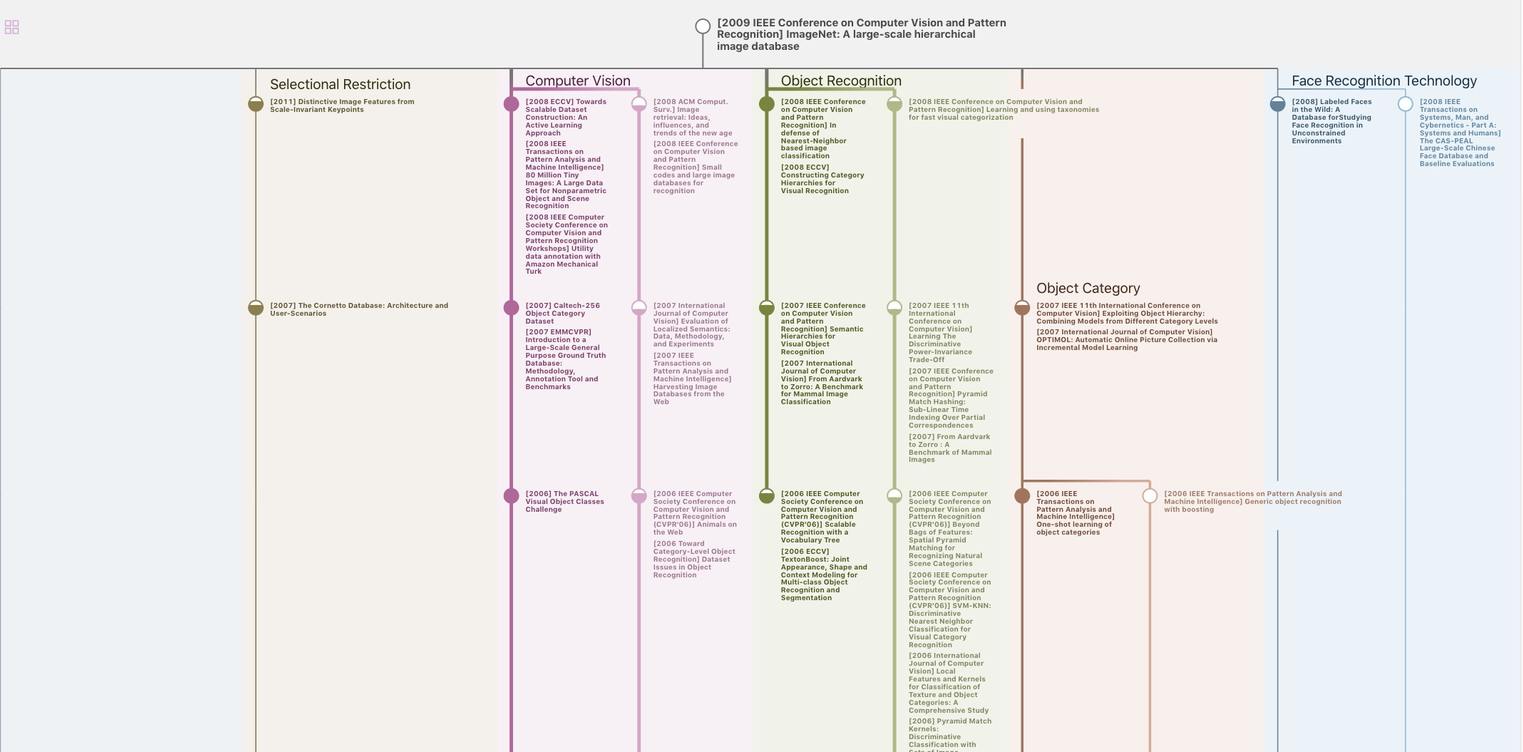
生成溯源树,研究论文发展脉络
Chat Paper
正在生成论文摘要