E-LSTM-D: A Deep Learning Framework for Dynamic Network Link Prediction
CoRR(2021)
摘要
Predicting the potential relations between nodes in networks, known as link prediction, has long been a challenge in network science. However, most studies just focused on link prediction of static network, while real-world networks always evolve over time with the occurrence and vanishing of nodes and links. Dynamic network link prediction (DNLP) thus has been attracting more and more attention since it can better capture the evolution nature of networks, but still most algorithms fail to achieve satisfied prediction accuracy. Motivated by the excellent performance of long short-term memory (LSTM) in processing time series, in this article, we propose a novel encoder-LSTM-decoder (E-LSTM-D) deep learning model to predict dynamic links end to end. It could handle long-term prediction problems, and suits the networks of different scales with fine-tuned structure. To the best of our knowledge, it is the first time that LSTM, together with an encoder-decoder architecture, is applied to link prediction in dynamic networks. This new model is able to automatically learn structural and temporal features in a unified framework, which can predict the links that never appear in the network before. The extensive experiments show that our E-LSTM-D model significantly outperforms newly proposed DNLP methods and obtain the state-of-the-art results.
更多查看译文
关键词
Dynamic network,encoder–decoder,link prediction,long short-term memory (LSTM),network embedding
AI 理解论文
溯源树
样例
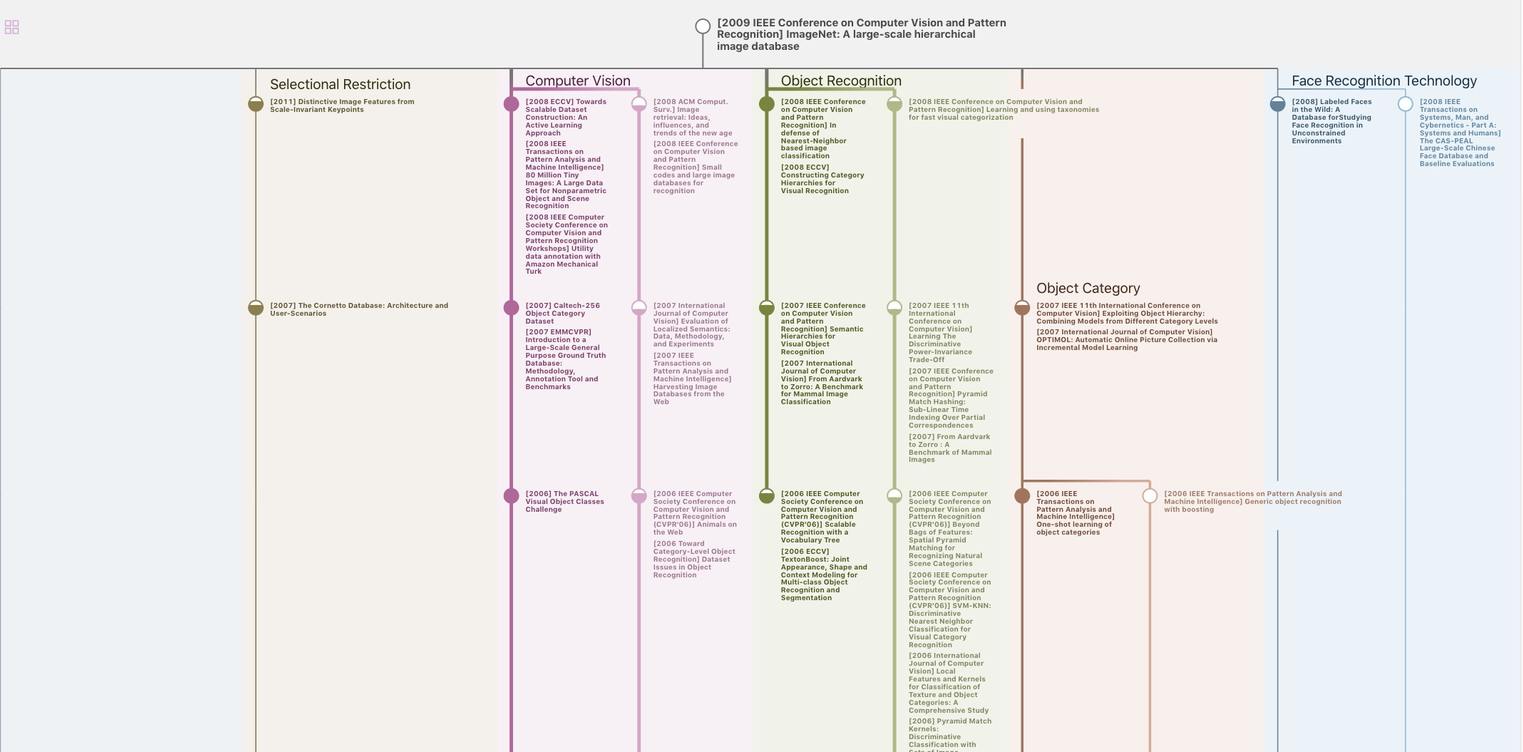
生成溯源树,研究论文发展脉络
Chat Paper
正在生成论文摘要