Learning Neurosymbolic Generative Models via Program Synthesis
INTERNATIONAL CONFERENCE ON MACHINE LEARNING, VOL 97(2019)
摘要
Generative models have become significantly more powerful in recent years. However, these models continue to have difficulty capturing global structure in data. For example, images of buildings typically contain spatial patterns such as windows repeating at regular intervals, but state-of-the-art models have difficulty generating these patterns. We propose to address this problem by incorporating programs representing global structure into generative models-e.g., a 2D for-loop may represent a repeating pattern of windows- along with a framework for learning these models by leveraging program synthesis to obtain training data. On both synthetic and real-world data, we demonstrate that our approach substantially outperforms state-of-the-art at both generating and completing images with global structure.
更多查看译文
关键词
neurosymbolic generative models,program synthesis,learning
AI 理解论文
溯源树
样例
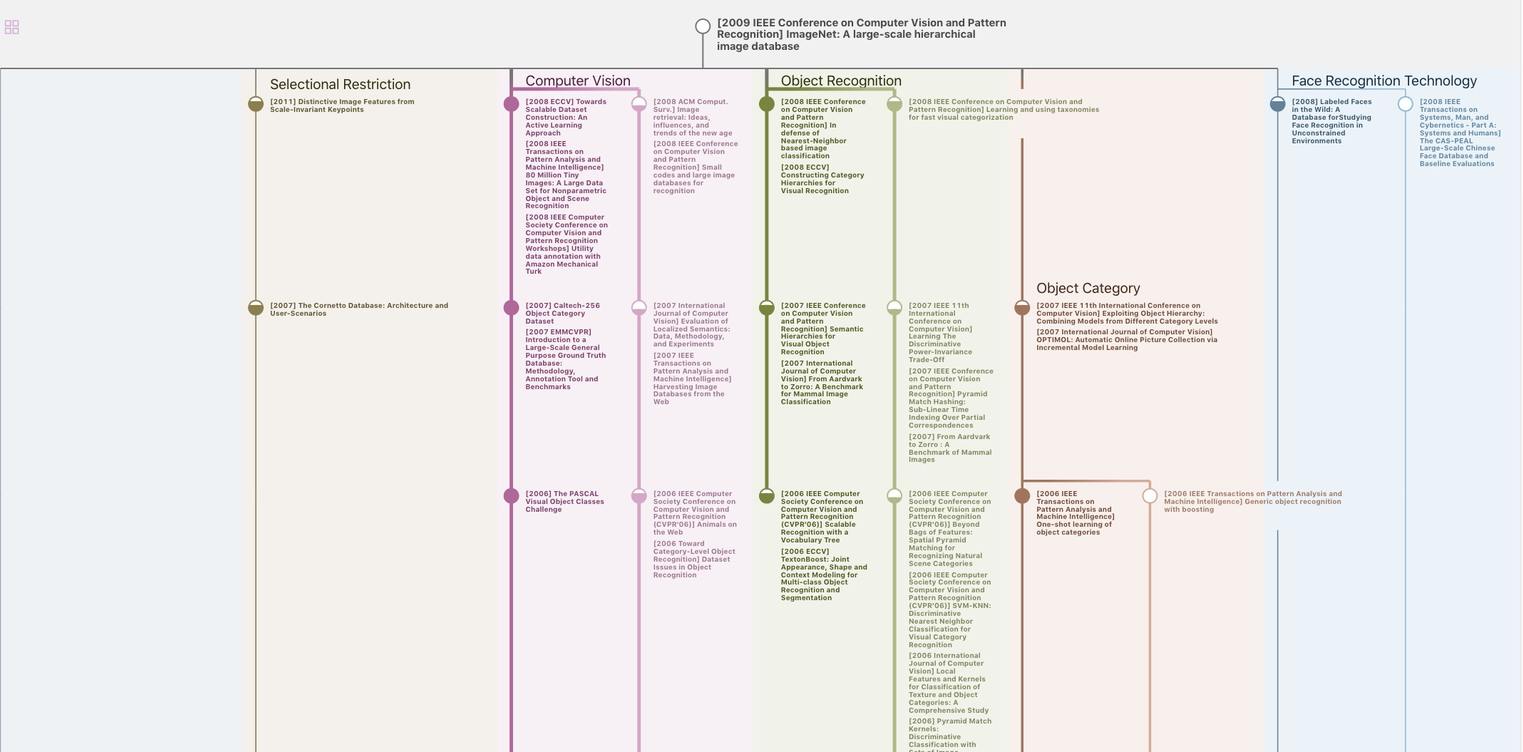
生成溯源树,研究论文发展脉络
Chat Paper
正在生成论文摘要