Error bounds for approximations with deep ReLU neural networks in $W^{s, p}$ norms.
Analysis and Applications(2020)
摘要
We analyze approximation rates of deep ReLU neural networks for Sobolev-regular functions with respect to weaker Sobolev norms. First, we construct, based on a calculus of ReLU networks, artificial neural networks with ReLU activation functions that achieve certain approximation rates. Second, we establish lower bounds for the approximation by ReLU neural networks for classes of Sobolev-regular functions. Our results extend recent advances in the approximation theory of ReLU networks to the regime that is most relevant for applications in the numerical analysis of partial differential equations.
更多查看译文
AI 理解论文
溯源树
样例
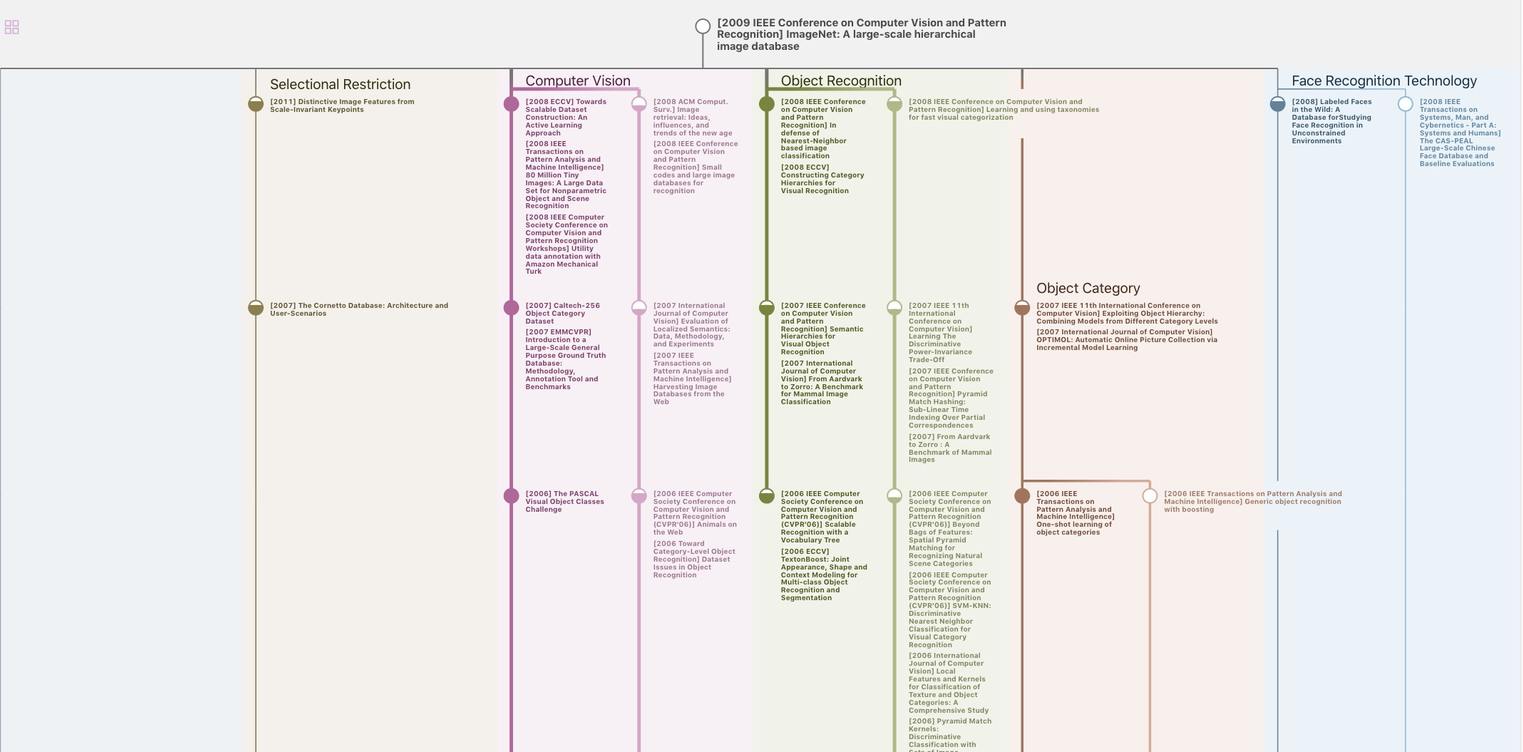
生成溯源树,研究论文发展脉络
Chat Paper
正在生成论文摘要