Exact Markov chain-based runtime analysis of a discrete particle swarm optimization algorithm on sorting and OneMax
Natural Computing(2021)
摘要
Meta-heuristics are powerful tools for solving optimization problems whose structural properties are unknown or cannot be exploited algorithmically. We propose such a meta-heuristic for a large class of optimization problems over discrete domains based on the particle swarm optimization (PSO) paradigm. We provide a comprehensive formal analysis of the performance of this algorithm on certain “easy” reference problems in a black-box setting, namely the sorting problem and the problem O ne M ax . In our analysis we use a Markov model of the proposed algorithm to obtain upper and lower bounds on its expected optimization time. Our bounds are essentially tight with respect to the Markov model. We show that for a suitable choice of algorithm parameters the expected optimization time is comparable to that of known algorithms and, furthermore, for other parameter regimes, the algorithm behaves less greedy and more explorative, which can be desirable in practice in order to escape local optima. Our analysis provides a precise insight on the tradeoff between optimization time and exploration. To obtain our results we introduce the notion of indistinguishability of states of a Markov chain and provide bounds on the solution of a recurrence equation with non-constant coefficients by integration.
更多查看译文
关键词
Particle swarm optimization,Discrete optimization,Runtime analysis,Markov chains,Heuristics
AI 理解论文
溯源树
样例
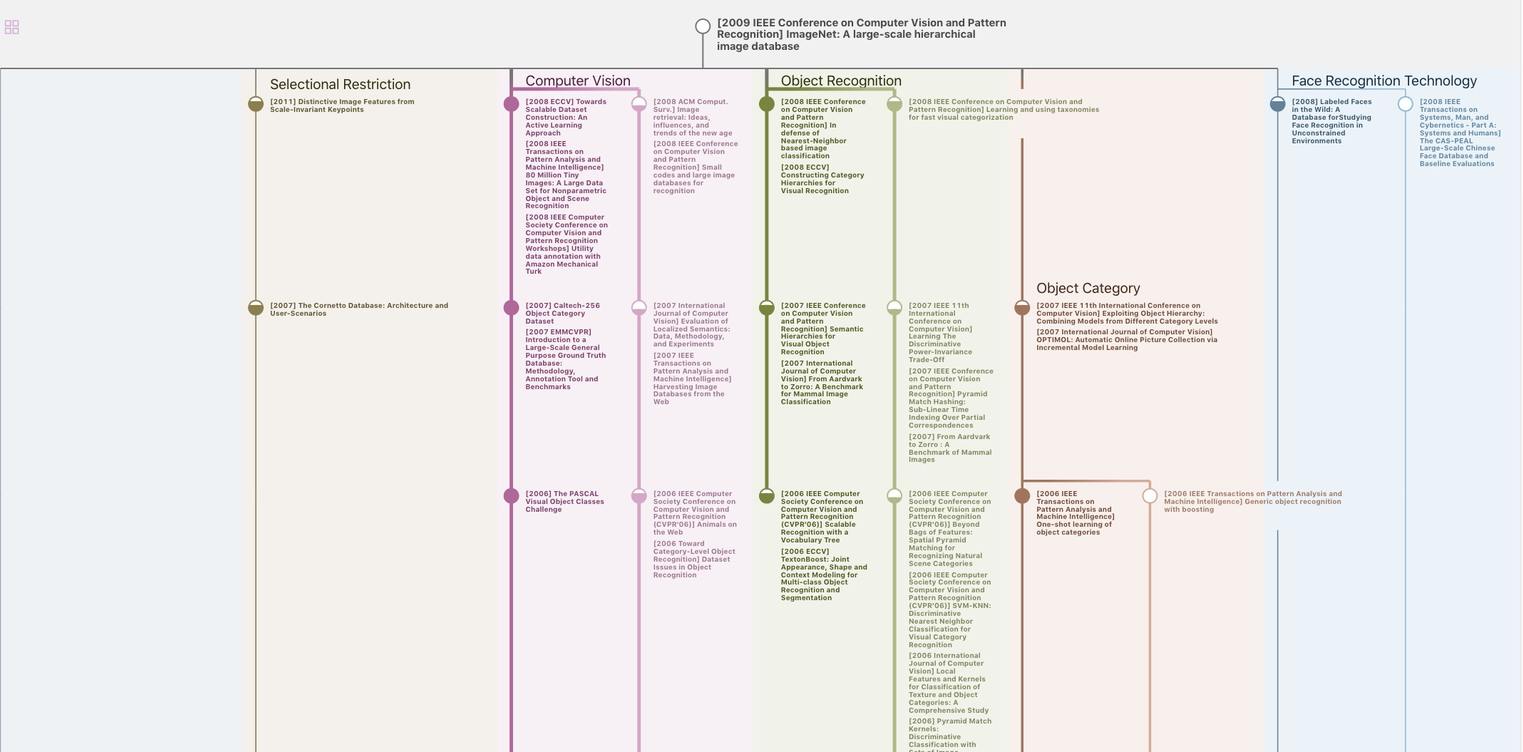
生成溯源树,研究论文发展脉络
Chat Paper
正在生成论文摘要