$Deep-Full-Range$ : A Deep Learning Based Network Encrypted Traffic Classification and Intrusion Detection Framework.
IEEE ACCESS(2019)
摘要
With the rapid evolution of network traffic diversity, the understanding of network traffic has become more pivotal and more formidable. Previously, traffic classification and intrusion detection require a burdensome analyzing of various traffic features and attack-related characteristics by experts, and even, private information might be required. However, due to the outdated features labeling and privacy protocols, the existing approaches may not fit with the characteristics of the changing network environment anymore. In this paper, we present a light-weight framework with the aid of deep learning for encrypted traffic classification and intrusion detection, termed as deep-full-range (DFR). Thanks to deep learning, DFR is able to learn from raw traffic without manual intervention and private information. In such a framework, our proposed algorithms are compared with other state-of-the-art methods using two public datasets. The experimental results show that our framework not only can outperform the state-of-the-art methods by averaging 13.49% on encrypted traffic classification's F1 score and by averaging 12.15% on intrusion detection's F1 score but also require much lesser storage resource requirement.
更多查看译文
关键词
Encrypted traffic classification,network intrusion detection,deep learning,end-to-end
AI 理解论文
溯源树
样例
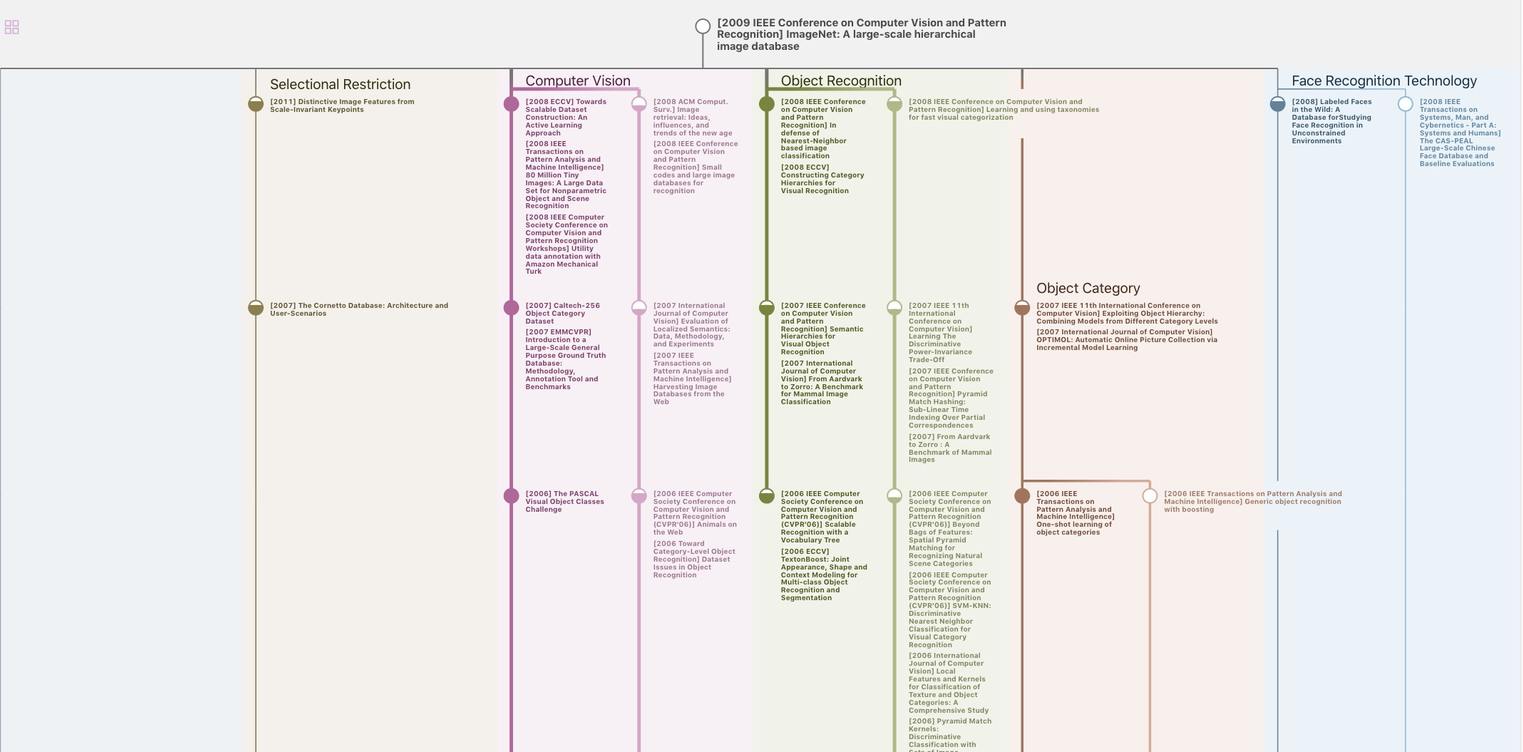
生成溯源树,研究论文发展脉络
Chat Paper
正在生成论文摘要