WGAN-Based Synthetic Minority Over-Sampling Technique: Improving Semantic Fine-Grained Classification for Lung Nodules in CT Images.
IEEE ACCESS(2019)
摘要
Data imbalance issue generally exists in most medical image analysis problems and maybe getting important with the popularization of data-hungry deep learning paradigms. We explore the cutting-edge Wasserstein generative adversarial networks (WGANs) to address the data imbalance problem with oversampling on the minority classes. The WGAN can estimate the underlying distribution of a minority class to synthesize more plausible and helpful samples for the classification model. In this paper, the WGAN-based over-sampling technique is applied to augment the data to balance for the fine-grained classification of seven semantic attributes of lung nodules in computed tomography images. The fine-grained classification is carried out with a normal convolutional neural network (CNN). To further illustrate the efficacy of the WGAN-based over-sampling technique, the conventional data augmentation method commonly used in many deep learning works, the generative adversarial networks (GANs), and the deep convolutional generative adversarial networks (DCGANs) are implemented for comparison. The whole schemes of the minority oversampling and fine-grained classification are tested with the public lung imaging database consortium dataset. The experimental results suggest that the WGAN-based oversampling technique can synthesize helpful samples for the minority classes to assist the training of the CNN model and to boost the fine-grained classification performance better than the conventional data augmentation method and the two schemes of the GAN and DCGAN techniques do. It may thus suggest that the WGAN technique offers an alternative methodological option for the further deep learning on imbalanced classification studies.
更多查看译文
关键词
Computer-aided diagnosis (CAD),lung nodule,computed tomography (CT),synthetic minority over-sampling,deep learning,data imbalance,adversarial neural networks
AI 理解论文
溯源树
样例
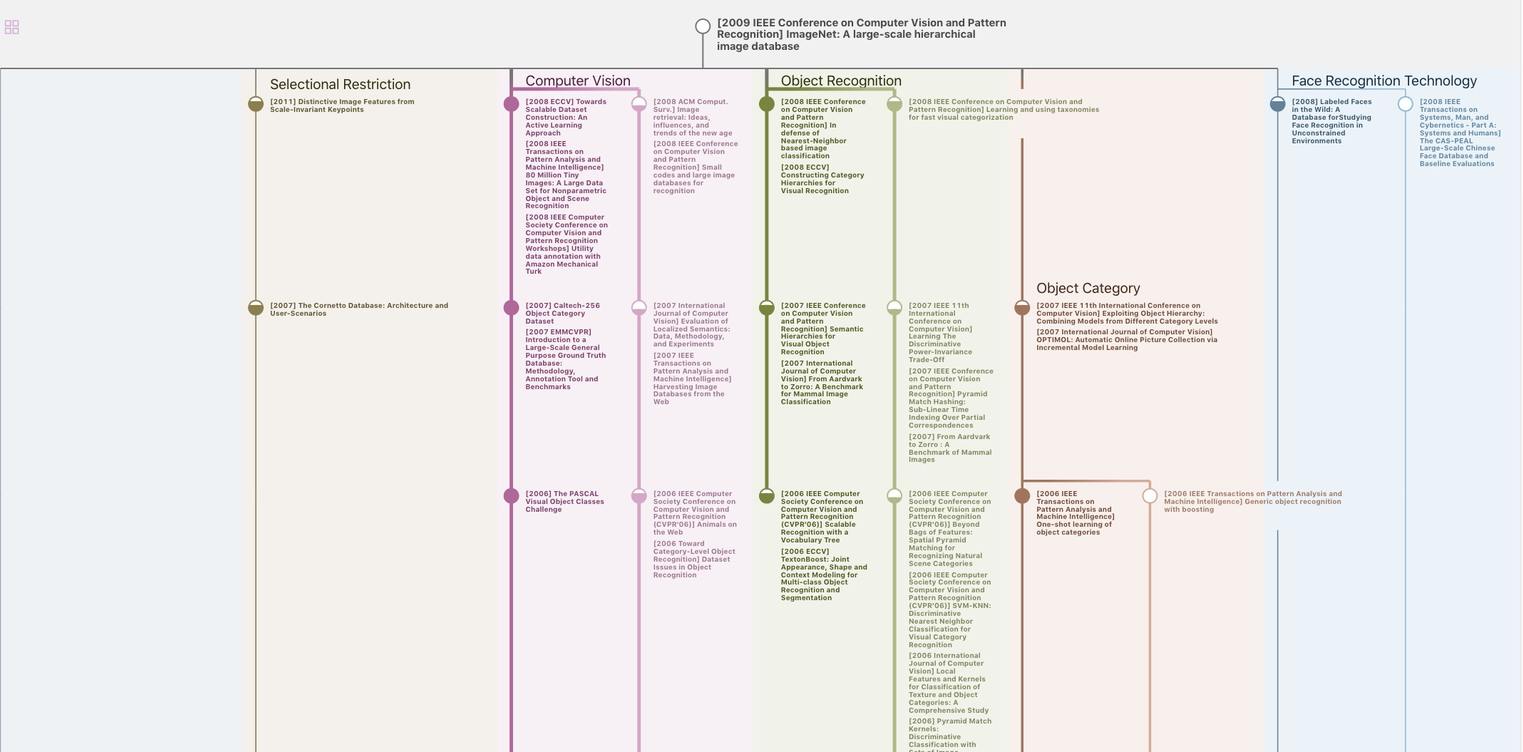
生成溯源树,研究论文发展脉络
Chat Paper
正在生成论文摘要