MVF-CNN: Fusion of Multilevel Features for Large-Scale Point Cloud Classification.
IEEE ACCESS(2019)
摘要
Due to the disorder, sparsity, and irregularity of point clouds, the accurate classification of large scale point clouds is a challenging problem. Voxelization-based deep learning methods have been applied to point cloud classification and have achieved good performances. However, there are some problems in the methods, such as voxels lacking color information, single receptive fields being considered only at the same voxel scale, and only the global features of voxels being considered in the classification. This paper proposes a deep learning-based algorithm for large-scale point cloud classification through the fusion of multiscale voxels and features (MVF-CNN). First, the point cloud is transformed into voxels of two different sizes. Then, the voxels are input into the 3D convolutional neural network (3D CNN) with three different scale receptive fields for the feature extraction. Next, the output features of the 3D CNN are input into the proposed global and local feature fusion classification network (GLNet), which fuses the global features of the voxels at the main branch and the local features of each voxel at the auxiliary branch. Finally, the multiscale features of the main branch are fused, and its classification results are obtained. We have conducted the experiments on six-point cloud scenes. The experimental results show that the proposed algorithm accurately classifies large-scale point clouds. Compared with several semisupervised/supervised learning methods, the proposed algorithm obtains better classification results. In addition, the experimental results also demonstrate that the proposed algorithm has a strong generalization ability and obviously has a better classification performance than the compared algorithms.
更多查看译文
关键词
Point cloud,semantic segmentation,multiscale voxels,3D convolutional neural network (3D CNN)
AI 理解论文
溯源树
样例
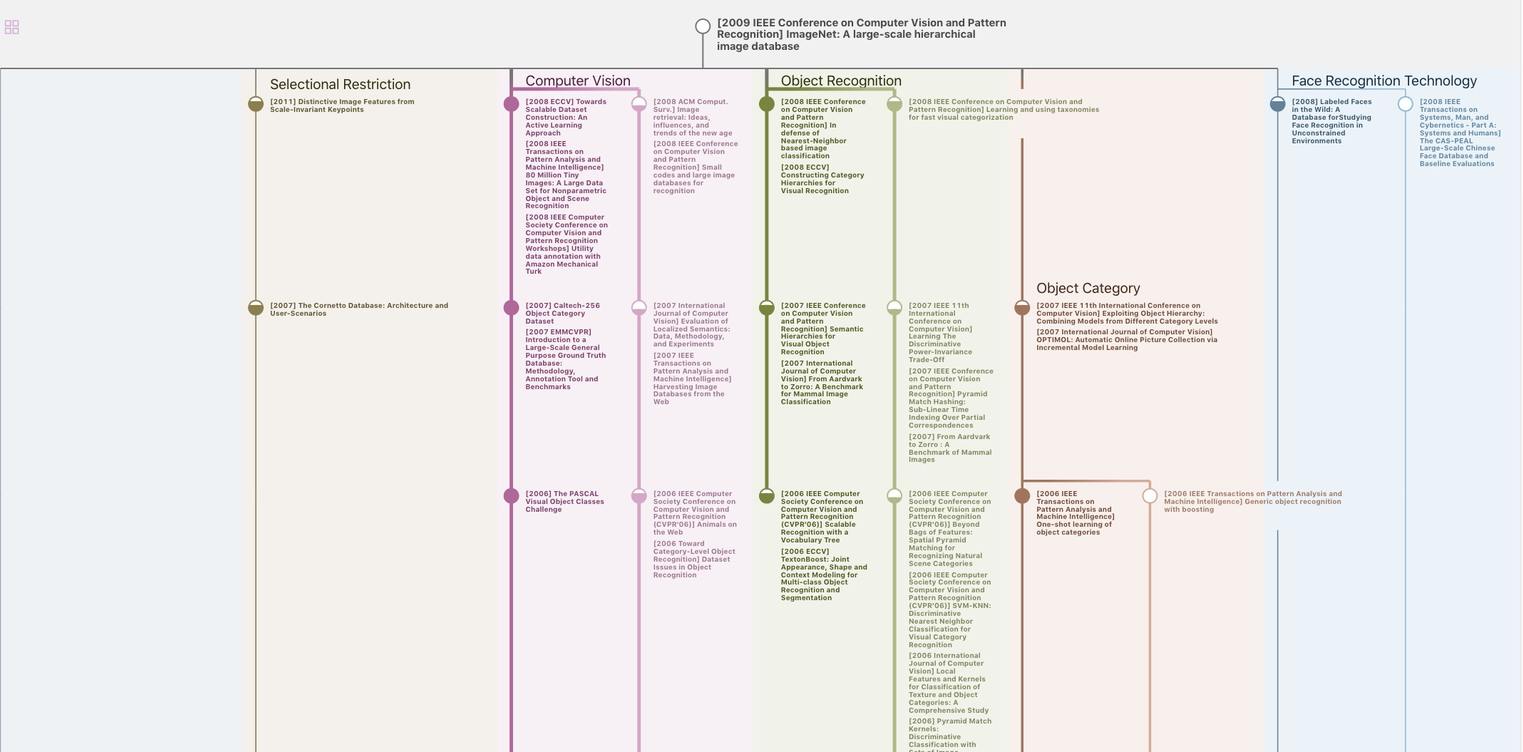
生成溯源树,研究论文发展脉络
Chat Paper
正在生成论文摘要