On the Global Minimizers of Real Robust Phase Retrieval With Sparse Noise
IEEE Transactions on Information Theory(2021)
摘要
AbstractWe study a class of real robust phase retrieval problems under a Gaussian assumption on the coding matrix when the received signal is sparsely corrupted by noise. The goal is to establish conditions on the sparsity under which the input vector can be exactly recovered. The recovery problem is formulated as residual minimization in the $\ell _{1}$ -norm. The main contribution is a robust phase retrieval counterpart to the seminal paper by Candes and Tao on compressed sensing ( $\ell _{1}$ regression) [Decoding by linear programming. IEEE Transactions on Information Theory, 51(12):4203–4215, 2005]. The analysis depends on a key new property of the coding matrix called the Absolute Range Property (ARP) which is the analogue to the Null Space Property (NSP) in compressed sensing. When the residuals are computed using squared magnitudes, we show that ARP follows from a standard Restricted Isometry Property (RIP). However, when the residuals are computed using absolute magnitudes, a different kind of RIP or growth property is required. We conclude by showing that the robust phase retrieval objectives are sharp with respect to their minimizers with high probability.
更多查看译文
关键词
Decoding, sparse matrices, mathematics, phase estimation, information theory
AI 理解论文
溯源树
样例
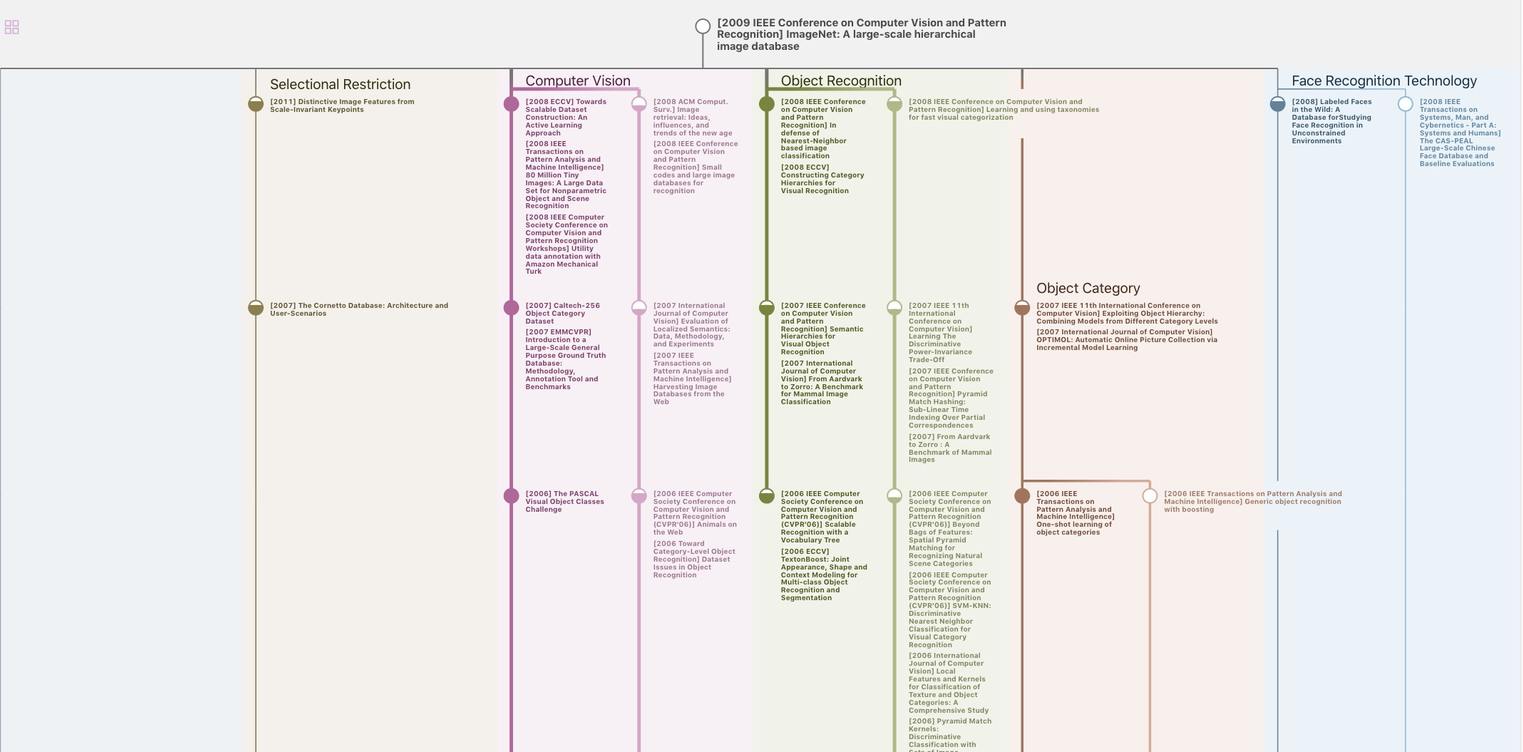
生成溯源树,研究论文发展脉络
Chat Paper
正在生成论文摘要