Neural Temporal Difference and Q Learning Provably Converge to Global Optima
MATHEMATICS OF OPERATIONS RESEARCH(2024)
摘要
Temporal difference learning (TD), coupled with neural networks, is among the most fundamental building blocks of deep reinforcement learning. However, because of the nonlinearity in value function approximation, such a coupling leads to nonconvexity and even divergence in optimization. As a result, the global convergence of neural TD remains unclear. In this paper, we prove for the first time that neural TD converges at a sublinear rate to the global optimum of the mean-squared projected Bellman error for policy evaluation. In particular, we show how such global convergence is enabled by the over parameterization of neural networks, which also plays a vital role in the empirical success of neural TD. We establish the theory for two-layer neural networks in the main paper and extend them to multilayer neural networks in the appendix. Beyond policy evaluation, we establish the global convergence of neural (soft) Q learning.
更多查看译文
关键词
Primary: 90C40,secondary: 68T05,reinforcement learning,temporal difference learning,overparameterized neural network
AI 理解论文
溯源树
样例
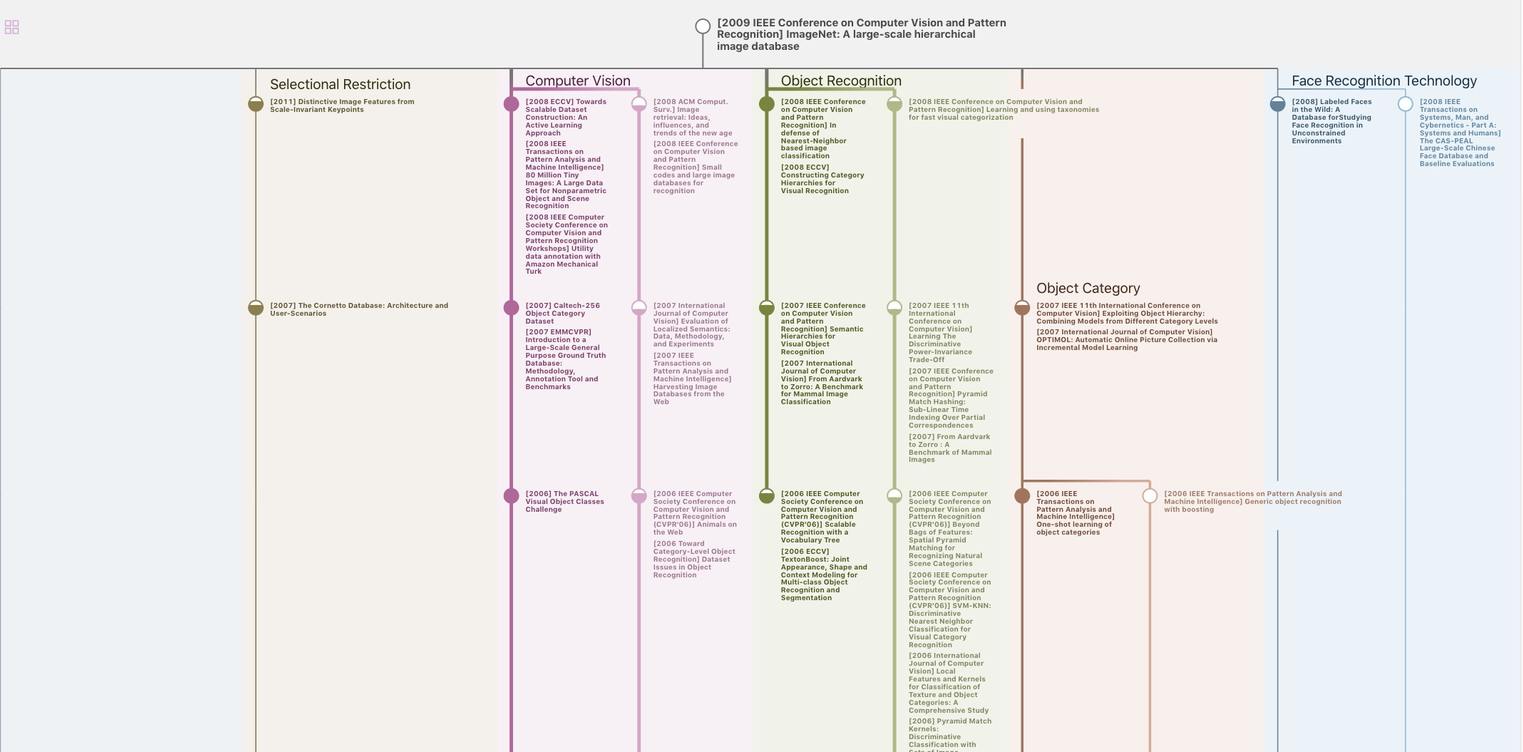
生成溯源树,研究论文发展脉络
Chat Paper
正在生成论文摘要