Peregrine: A Flexible Hardware Accelerator for LSTM with Limited Synaptic Connection Patterns
Proceedings of the 56th Annual Design Automation Conference 2019(2019)
摘要
In this paper, we present an integrated solution to design a high-performance LSTM accelerator. We propose a fast and flexible hardware architecture, named Peregrine, supported by a stack of innovations from algorithm to hardware design. Peregrine first minimizes the memory footprint by limiting the synaptic connection patterns within the LSTM network. Also, Peregrine provides parallel Huffman decoders with adaptive clocking to provide flexibility in dealing with a wide range of sparsity levels in the weight matrices. All these features are incorporated in a novel hardware architecture to maximize energy-efficiency. As a result, Peregrine improves performance by ~38% and energy-efficiency by ~33% in speech recognition compared to the state-of-the-art LSTM accelerator.
更多查看译文
关键词
Deep learning, energy-efficiency, hardware accelerator, recurrent neural network, sparse matrix format
AI 理解论文
溯源树
样例
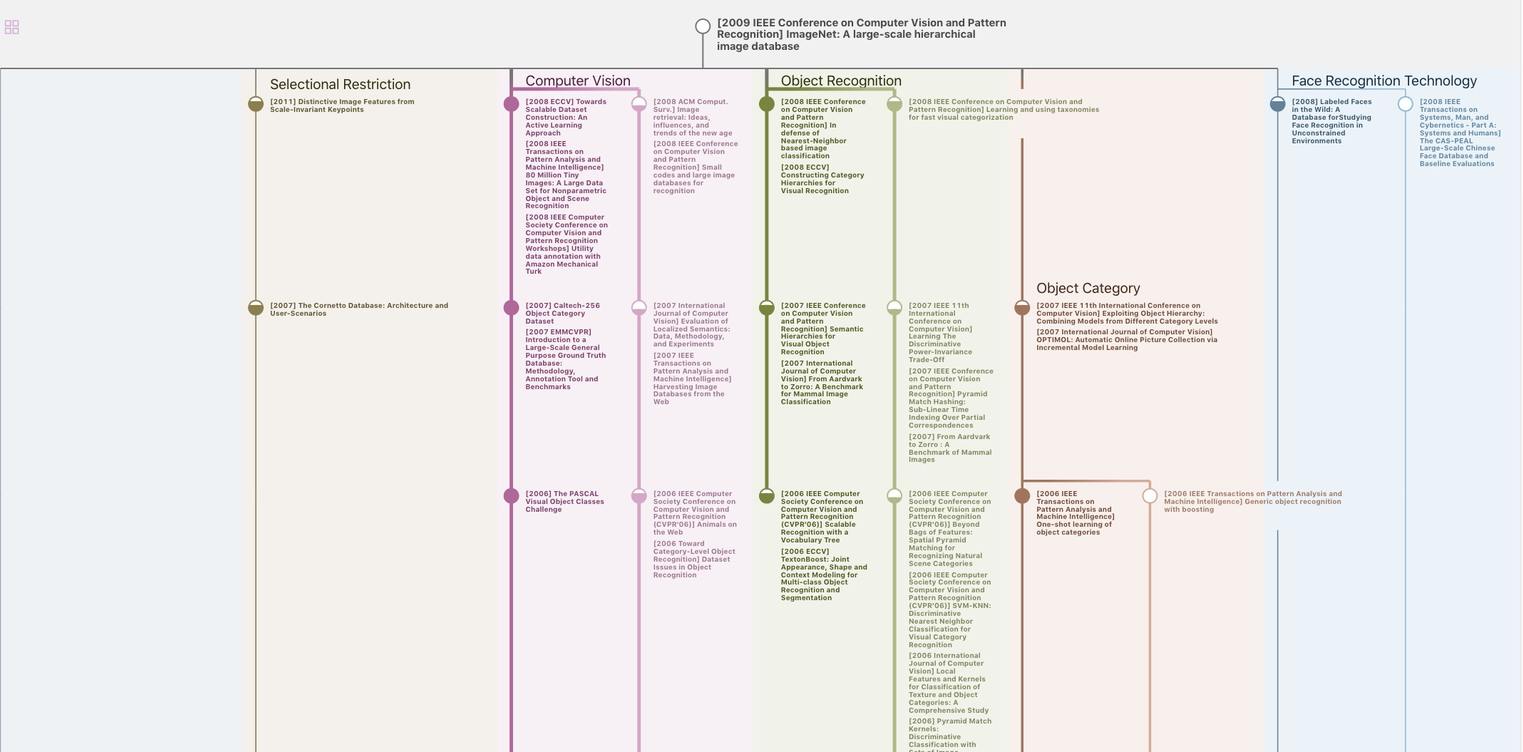
生成溯源树,研究论文发展脉络
Chat Paper
正在生成论文摘要