Attacking Split Manufacturing from a Deep Learning Perspective
Proceedings of the 56th Annual Design Automation Conference 2019(2019)
摘要
The notion of integrated circuit split manufacturing which delegates the front-end-of-line (FEOL) and back-end-of-line (BEOL) parts to different foundries, is to prevent overproduction, piracy of the intellectual property (IP), or targeted insertion of hardware Trojans by adversaries in the FEOL facility. In this work, we challenge the security promise of split manufacturing by formulating various layout-level placement and routing hints as vector- and image-based features. We construct a sophisticated deep neural network which can infer the missing BEOL connections with high accuracy. Compared with the publicly available network-flow attack [1], for the same set of ISCAS-85 benchmarks, we achieve 1.21× accuracy when splitting on M1 and 1.12× accuracy when splitting on M3 with less than 1% running time.
更多查看译文
关键词
sophisticated deep neural network,front-end-of-line,back-end-of-line parts,different foundries,FEOL facility,security promise,layout-level placement,image-based features,deep learning,IP piracy,integrated circuit split manufacturing,network-flow attack,BEOL connections,vector-based features,ISCAS-85benchmarks
AI 理解论文
溯源树
样例
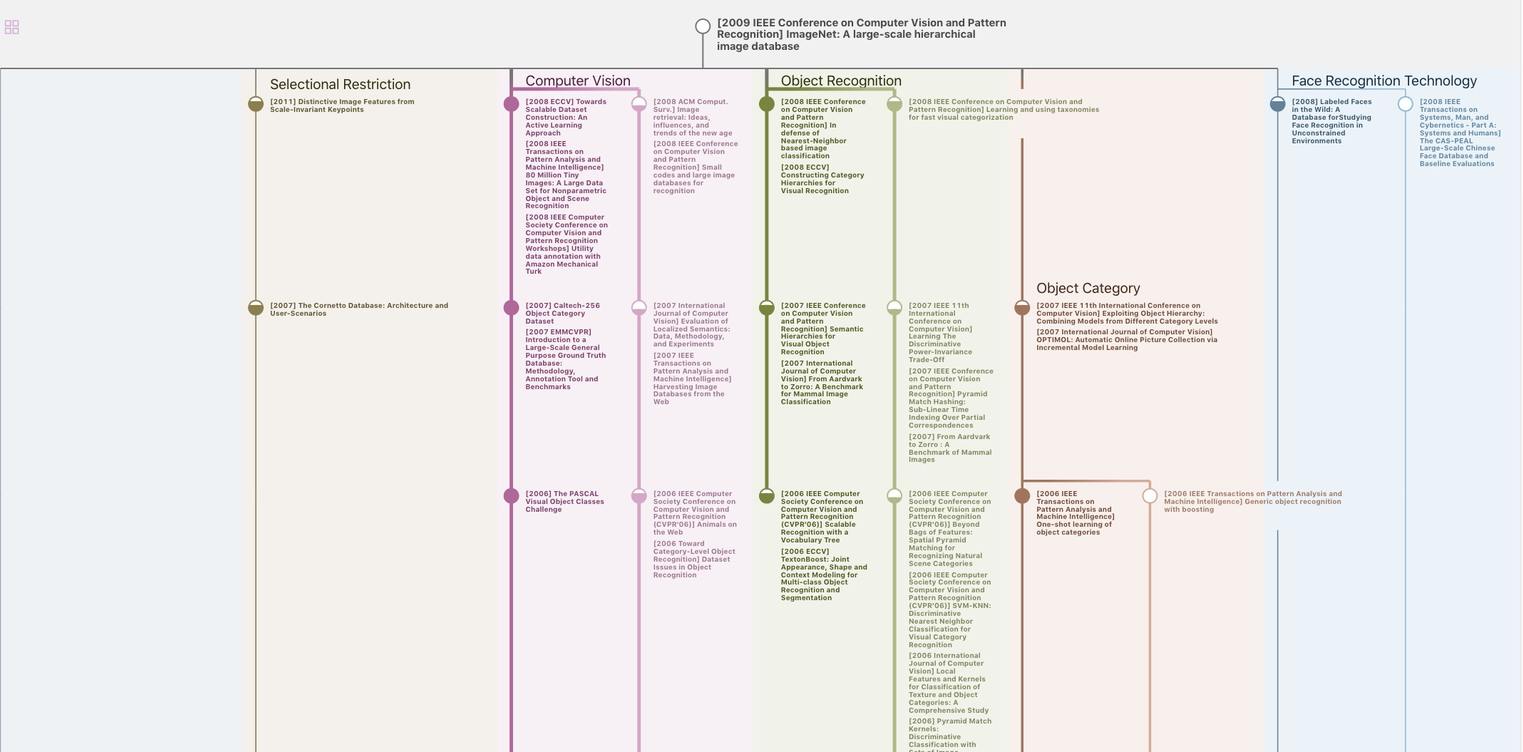
生成溯源树,研究论文发展脉络
Chat Paper
正在生成论文摘要