Improved Faster R-CNN Traffic Sign Detection Based on a Second Region of Interest and Highly Possible Regions Proposal Network.
SENSORS(2019)
摘要
Traffic sign detection systems provide important road control information for unmanned driving systems or auxiliary driving. In this paper, the Faster region with a convolutional neural network (R-CNN) for traffic sign detection in real traffic situations has been systematically improved. First, a first step region proposal algorithm based on simplified Gabor wavelets (SGWs) and maximally stable extremal regions (MSERs) is proposed. In this way, the region proposal a priori information is obtained and will be used for improving the Faster R-CNN. This part of our method is named as the highly possible regions proposal network (HP-RPN). Second, in order to solve the problem that the Faster R-CNN cannot effectively detect small targets, a method that combines the features of the third, fourth, and fifth layers of VGG16 to enrich the features of small targets is proposed. Third, the secondary region of interest method to enhance the feature of detection objects and improve the classification capability of the Faster R-CNN is proposed. Finally, a method of merging the German traffic sign detection benchmark (GTSDB) and Chinese traffic sign dataset (CTSD) databases into one larger database to increase the number of database samples is proposed. Experimental results show that our method improves the detection performance, especially for small targets.
更多查看译文
关键词
simplified Gabor filters,Faster R-CNN,secondary regions of interest,highly possible regions proposal
AI 理解论文
溯源树
样例
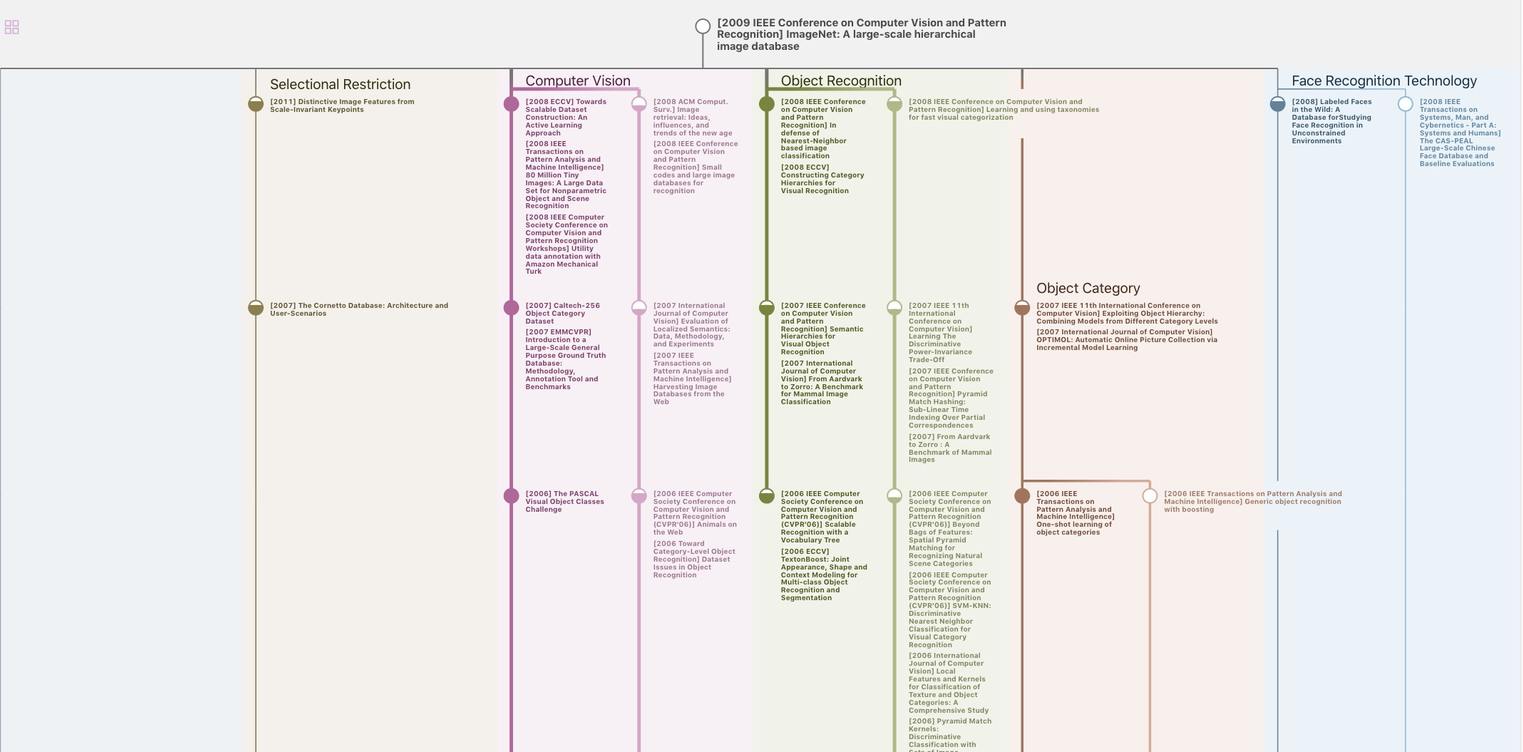
生成溯源树,研究论文发展脉络
Chat Paper
正在生成论文摘要