Learning to Reconstruct 3D Manhattan Wireframes from a Single Image
2019 IEEE/CVF INTERNATIONAL CONFERENCE ON COMPUTER VISION (ICCV 2019)(2019)
摘要
In this paper, we propose a method to obtain a compact and accurate 3D wireframe representation from a single image by effectively exploiting global structural regularities. Our method trains a convolutional neural network to simultaneously detect salient junctions and straight lines, as well as predict their 3D depths and vanishing points. Compared with the state-of-the-art learning-based wireframe detection methods, our network is much simpler and more unified, leading to better 2D wireframe detection. With global structural priors such as Manhattan assumption, our method further reconstructs a full 3D wireframe model, a compact vector representation suitable for a variety of high-level vision tasks such as AR and CAD. We conduct extensive evaluations on a large synthetic dataset of urban scenes as well as real images.
更多查看译文
关键词
single image,urban environment,global structural regularities,intuitive 3D wireframe representation,single convolutional neural network,2D wireframe detection,Manhattan assumption,compact vector representation,urban scenes,learning-based wireframe detection methods,3D Manhattan wireframe reconstruction,salient junction detection,3D depth,vanishing points,full 3D wireframe model,high-level vision tasks,synthetic dataset
AI 理解论文
溯源树
样例
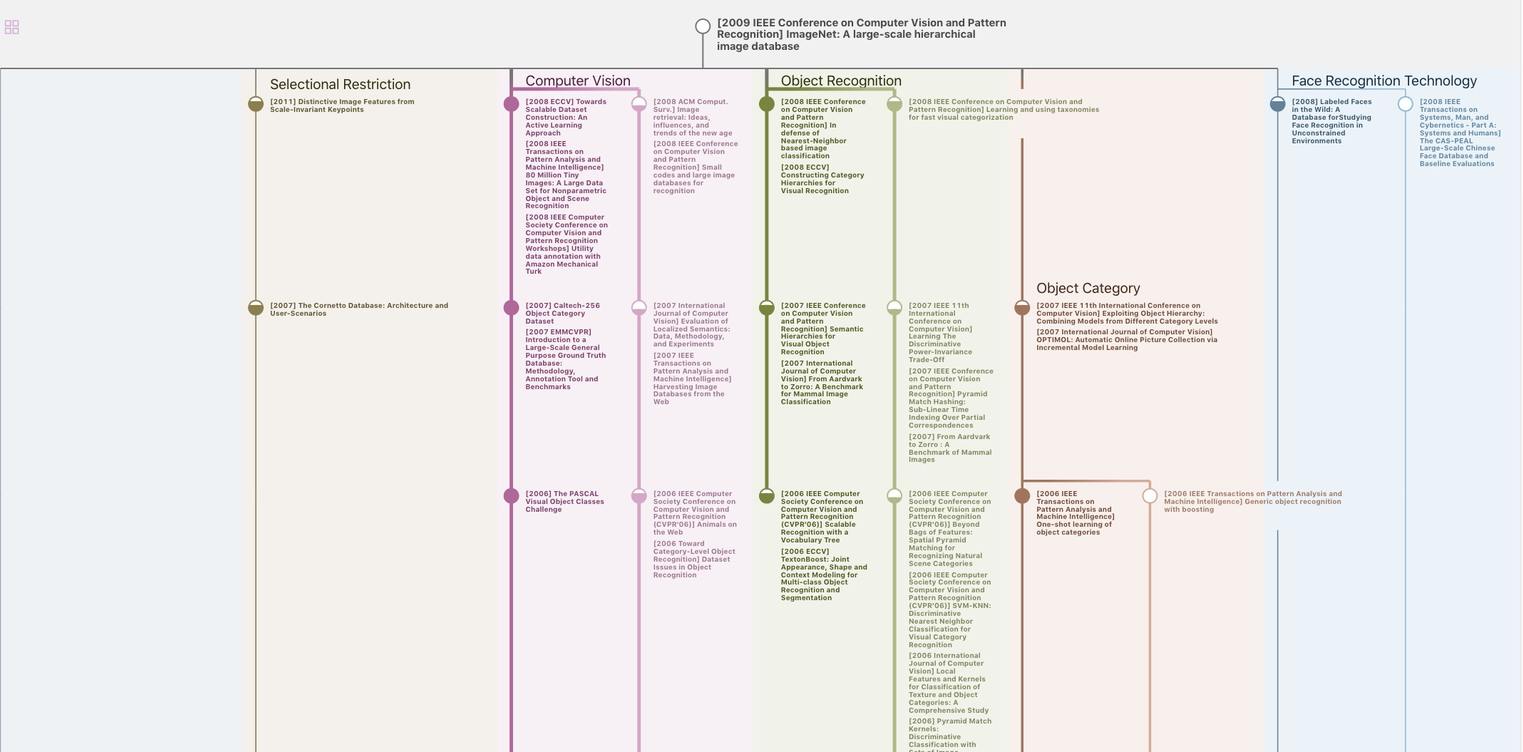
生成溯源树,研究论文发展脉络
Chat Paper
正在生成论文摘要