A versatile computational framework for group pattern mining of pedestrian trajectories
GeoInformatica(2019)
摘要
Mining patterns of large-scale trajectory data streams has been of increase research interest. In this paper, we are interested in mining group patterns of moving objects. Group pattern mining describes a special type of trajectory mining task that requires to efficiently discover trajectories of objects that are found in close proximity to each other for a period of time. In particular, we focus on trajectories of pedestrians coming from motion video analysis and we are interested in interactive analysis and exploration of group dynamics, including various definitions of group gathering and dispersion . Traditional approaches to solve the problem adhere to strict definition of group semantics. That restricts their application to specific problems and renders them inadequate for many real-world scenarios. To address this limitation, we propose a novel versatile method, timeWgroups , for efficient discovery of pedestrian groups that can adhere to different pattern semantics. First, the method efficiently discovers pairs of pedestrians that move together over time , under varying conditions of space and time. Subsequently, pairs of pedestrians are used as a building block for effectively discovering groups of pedestrians that can satisfy versatile group pattern semantics. As such, the proposed method can accommodate many different scenarios and application requirements. In addition, we introduce a new group pattern, individual perspective grouping that focuses on how individuals perceive groups. Based on the new group pattern we define the concept of dominant groups , a global metric for defining important groups that respects the individual perspective group pattern. Through experiments on real data, we demonstrate the effectiveness of our methods on discovering group patterns of pedestrian trajectories against sensible baselines, for a varying range of conditions. Furthermore, a query-based search method is provided that allows for interactive exploration and analysis of group dynamics over time and space. In addition, a visual testing is performed on real motion video to assert the group dynamics discovered by our methods.
更多查看译文
关键词
Trajectory mining, Group pattern mining, Pedestrian behavior
AI 理解论文
溯源树
样例
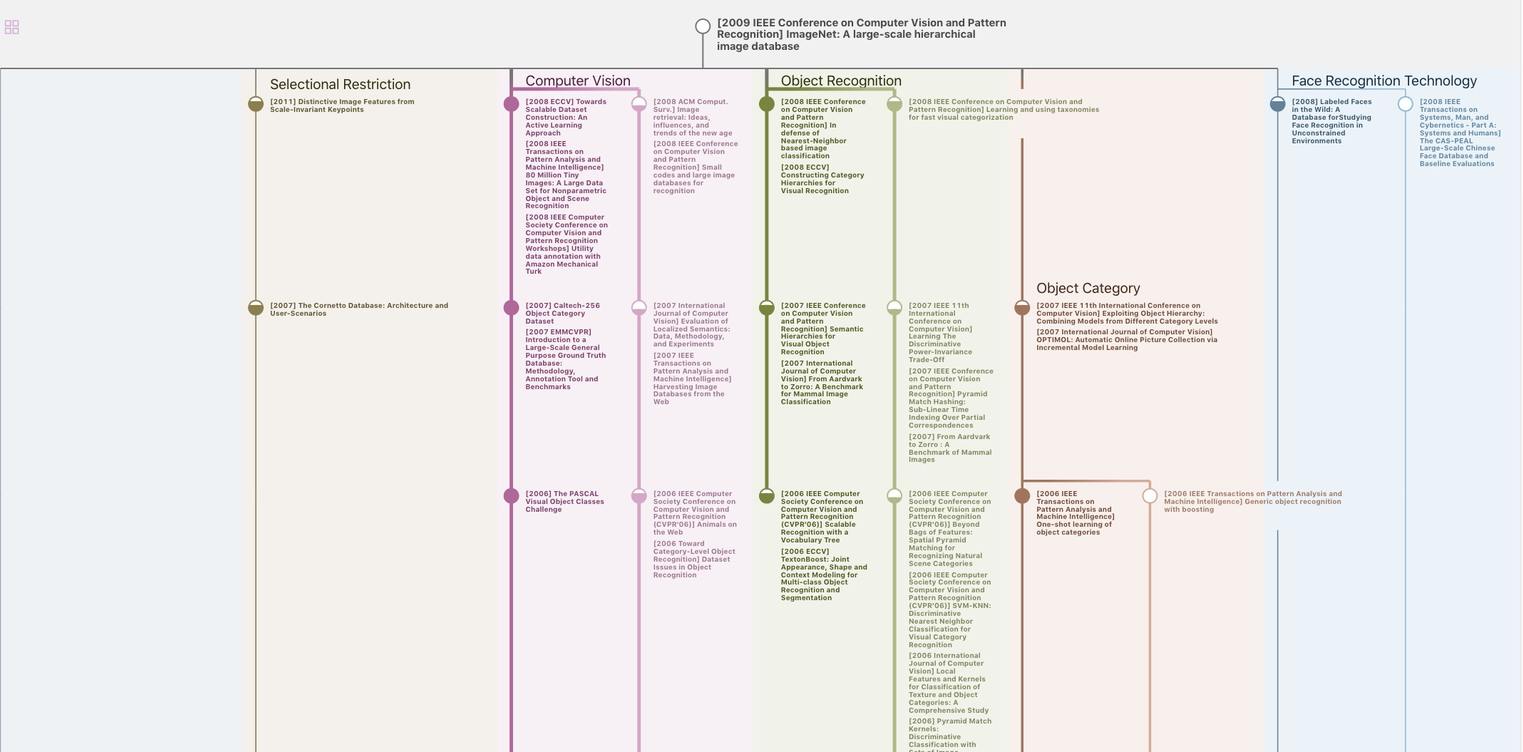
生成溯源树,研究论文发展脉络
Chat Paper
正在生成论文摘要