One-class support vector machines with a bias constraint and its application in system reliability prediction
AI EDAM-ARTIFICIAL INTELLIGENCE FOR ENGINEERING DESIGN ANALYSIS AND MANUFACTURING(2019)
摘要
Support vector machine (SVM) methods are widely used for classification and regression analysis. In many engineering applications, only one class of data is available, and then one-class SVM methods are employed. In reliability applications, the one-class data may be failure data since the data are recorded during reliability experiments when only failures occur. Different from the problems handled by existing one-class SVM methods, there is a bias constraint in the SVM model in this work and the constraint comes from the probability of failure estimated from the failure data. In this study, a new one-class SVM regression method is proposed to accommodate the bias constraint. The one class of failure data is maximally separated from a hypersphere whose radius is determined by the known probability of failure. The proposed SVM method generates regression models that directly link the states of failure modes with design variables, and this makes it possible to obtain the joint probability density of all the component states of an engineering system, resulting in a more accurate prediction of system reliability during the design stage. Three examples are given to demonstrate the effectiveness of the new one-class SVM method.
更多查看译文
关键词
First-order reliability method,optimization,support vector machines,system reliability,statistical dependence
AI 理解论文
溯源树
样例
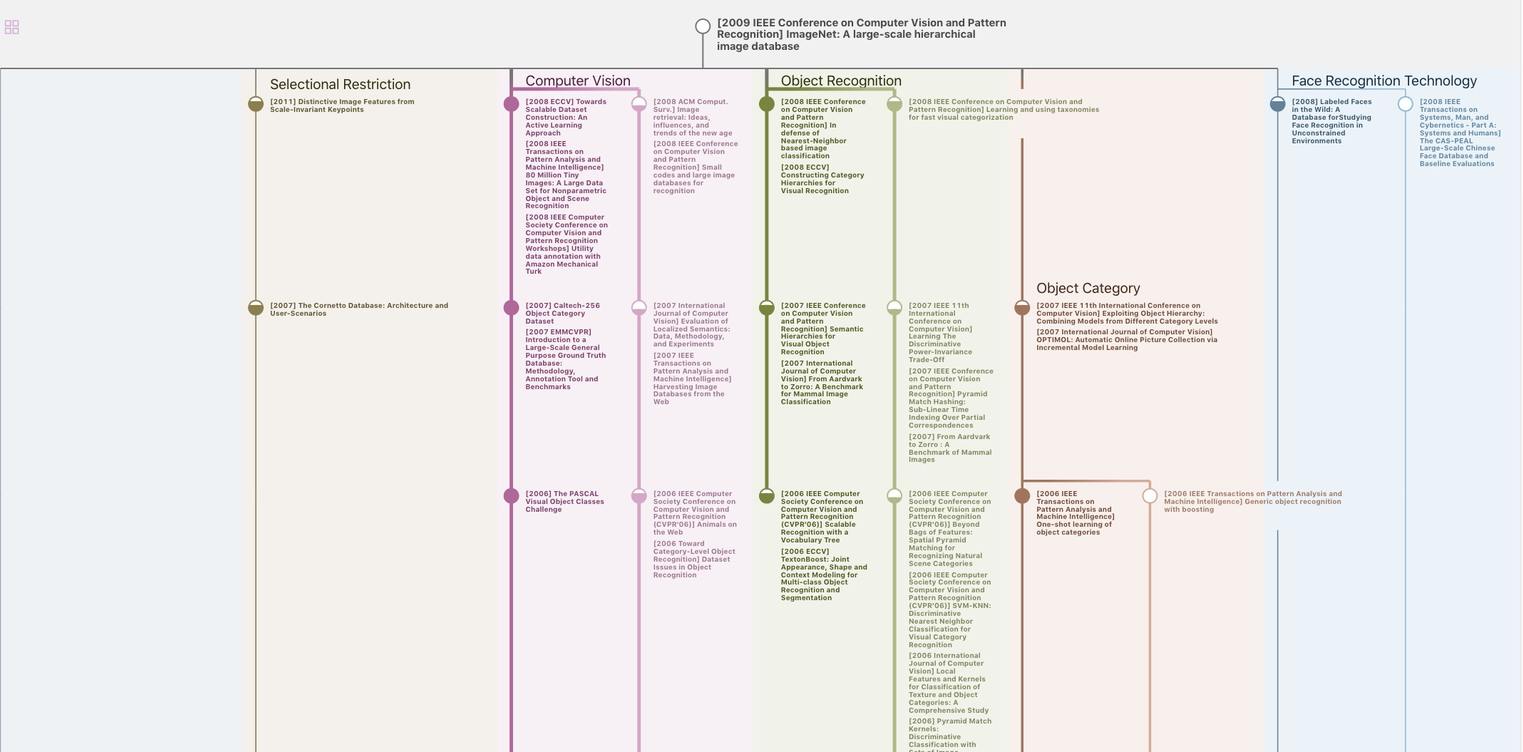
生成溯源树,研究论文发展脉络
Chat Paper
正在生成论文摘要