An In-Dram Neural Network Processing Engine
2019 IEEE INTERNATIONAL SYMPOSIUM ON CIRCUITS AND SYSTEMS (ISCAS)(2019)
摘要
Many advanced neural network inference engines are bounded by the available memory bandwidth. The conventional approach to address this issue is to employ high bandwidth memory devices or to adapt data compression techniques (reduced precision, sparse weight matrices). Alternatively, an emerging approach to bridge the memory-computation gap and to exploit extreme data parallelism is Processing in Memory (PIM). The close proximity of the computation units to the memory cells reduces the amount of external data transactions and it increases the overall energy efficiency of the memory system. In this work, we present a novel PIM based Binary Weighted Network (BWN) inference accelerator design that is inline with the commodity Dynamic Random Access Memory (DRAM) design and process. In order to exploit data parallelism and minimize energy, the proposed architecture integrates the basic BWN computation units at the output of the Primary Sense Amplifiers (PSAs) and the rest of the substantial logic near the Secondary Sense Amplifiers (SSAs). The power and area values are obtained at sub-array (SA) level using exhaustive circuit level simulations and full-custom layout. The proposed architecture results in an area overhead of 25% compared to a commodity 8 Gb DRAM and delivers a throughput of 63.59 FPS (Frames per Second) for AlexNet. We also demonstrate that our architecture is extremely energy efficient, 7.25 x higher FPS/W, as compared to previous works.
更多查看译文
关键词
Processing-in-Memory (PIM), DRAM, Binary Weighted Network (BWN), Convolution Neural Network (CNN)
AI 理解论文
溯源树
样例
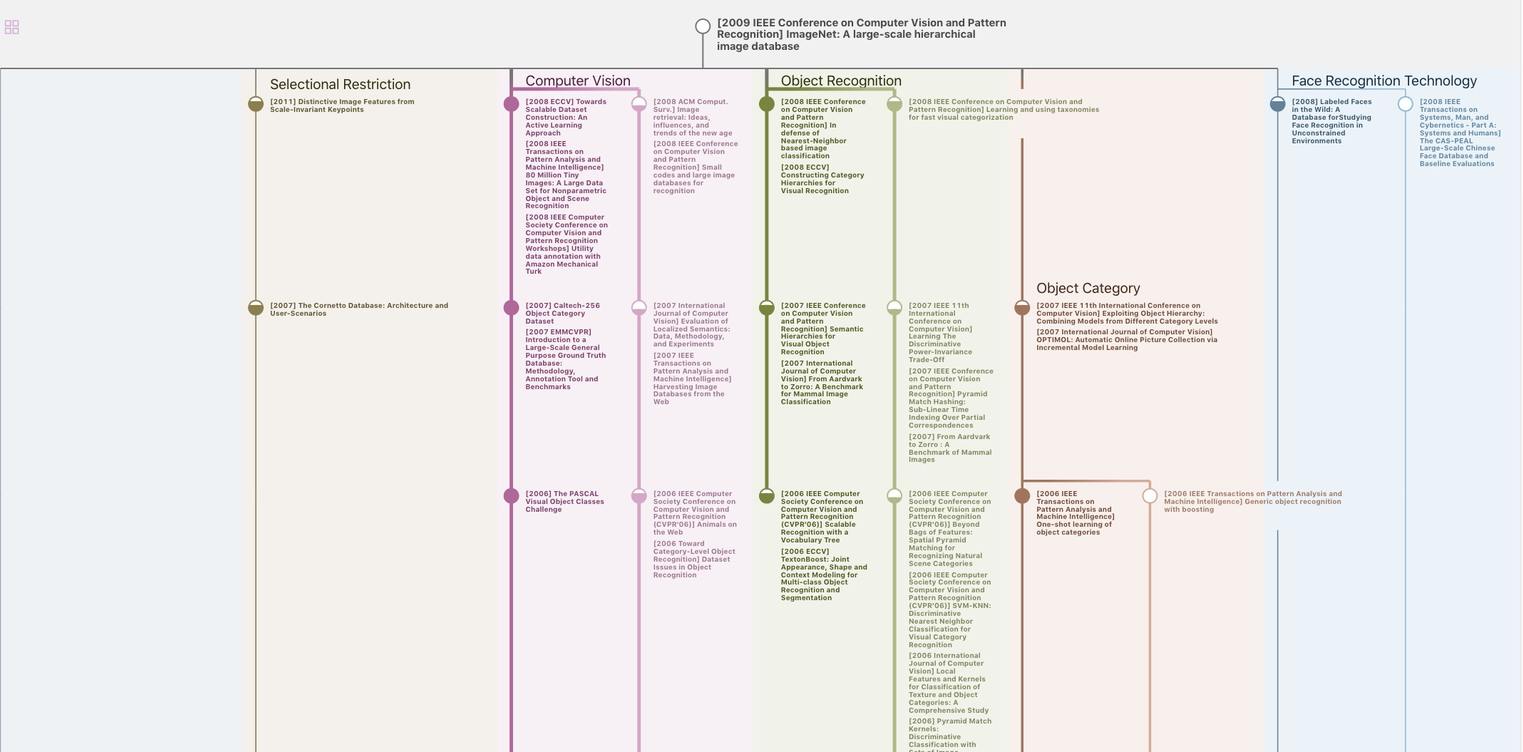
生成溯源树,研究论文发展脉络
Chat Paper
正在生成论文摘要