Robust Estimators in High-Dimensions Without the Computational Intractability
SIAM JOURNAL ON COMPUTING(2019)
摘要
We study high-dimensional distribution learning in an agnostic setting where an adversary is allowed to arbitrarily corrupt an epsilon-fraction of the samples. Such questions have a rich history spanning statistics, machine learning, and theoretical computer science. Even in the most basic settings, the only known approaches are either computationally inefficient or lose dimension-dependent factors in their error guarantees. This raises the following question: Is high-dimensional agnostic distribution learning even possible, algorithmically? In this work, we obtain the first computationally efficient algorithms with dimension-independent error guarantees for agnostically learning several fundamental classes of high-dimensional distributions: (1) a single Gaussian, (2) a product distribution on the hypercube, (3) mixtures of two product distributions (under a natural balancedness condition), and (4) mixtures of spherical Gaussians. Our algorithms achieve error that is independent of the dimension, and in many cases scales nearly linearly with the fraction of adversarially corrupted samples. Moreover, we develop a general recipe for detecting and correcting corruptions in high-dimensions that may be applicable to many other problems.
更多查看译文
关键词
robust learning,high-dimensions,Gaussian distribution,mixture models,product distributions
AI 理解论文
溯源树
样例
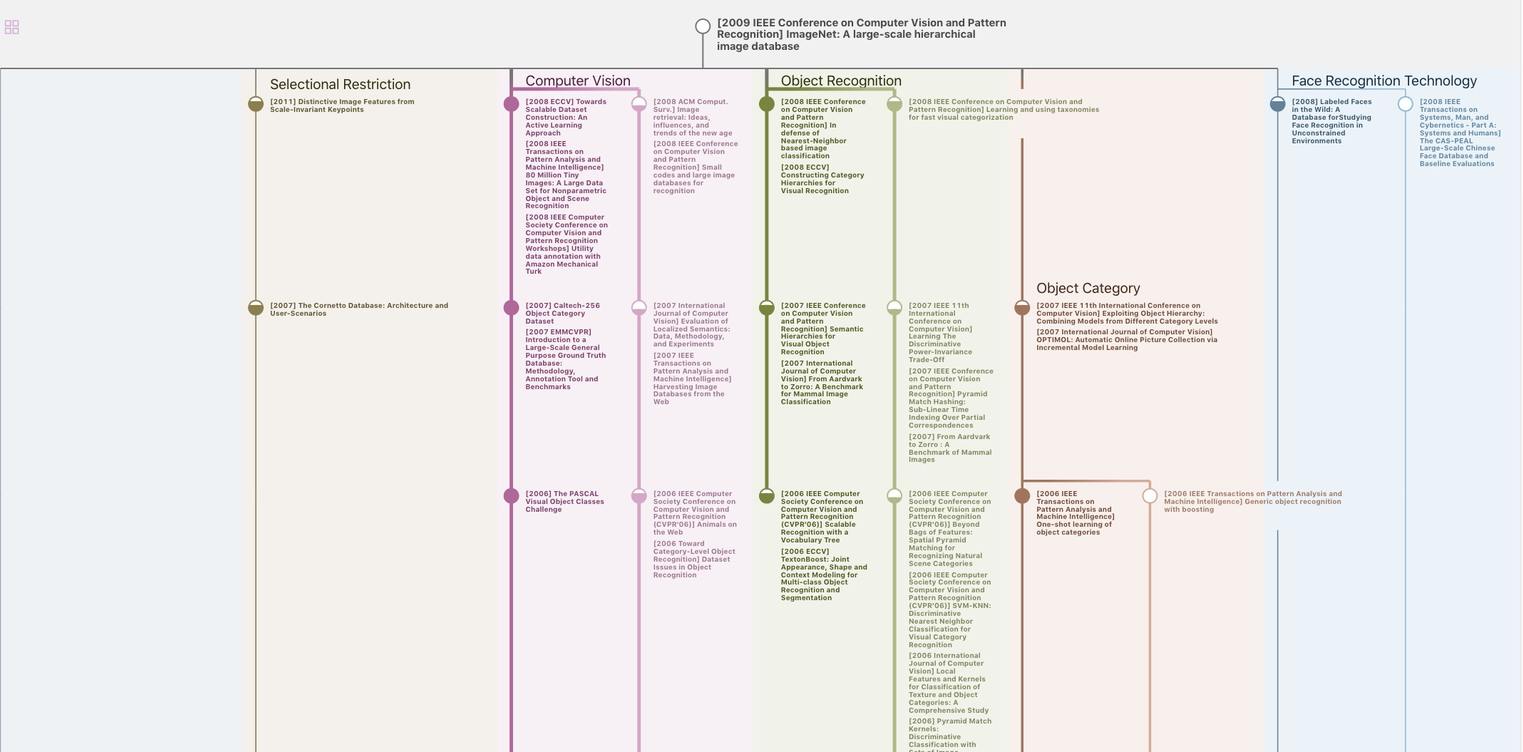
生成溯源树,研究论文发展脉络
Chat Paper
正在生成论文摘要