Marker subset selection and decision support range identification for acute myeloid leukemia classification model development with multiparameter flow cytometry
bioRxiv(2019)
摘要
In this study, we developed acute myeloid leukemia (AML) classification model through Wilks9 lambda based important marker identification method and stepwise forward selection approach, and spotted important decision support range of flow cytometry parameter using insights provided by machine learning algorithm. AML flow cytometry data released from FlowCAP II challenge in 2011 was used. In FlowCAP II challenge, several sample classification algorithms were able to effectively classify AML and nonAML. Most algorithms extracted features from high dimensional flow cytometry readout comprised of multiple fluorescent parameters for a large number of antibodies. Multiple parameters with forward scatter and side scatter increase computational complexity in the feature extraction procedure as well as in the model development. Parameter subset selection can decrease model complexity, improve model performance, and contribute to a panel design specific for target disease. With this motivation, we estimated importance of each parameter via Wilks9 lambda and then identified the best subset of parameters using stepwise forward selection. In the importance-estimation process, histogram matrix of each parameter was used. As a result, parameters, which are associated with blasts gating and identification of immature myeloid cells, were identified as important descriptors in AML classification, and combination of these markers is more effective than an individual marker. A random forest, supervised classification machine learning algorithm was used for the model development. We highlighted decision support range of the fluorescent signal for the identified important parameters, which significantly contribute to AML classification, through a mean decrease in Gini supported in random forest. These specific ranges could help with establishing diagnosis criteria and elaborate the AML classification model. Because methodology proposed in this study can not only estimate the importance of each parameter but also identify the best subset and the specific ranges, we expect that it would contribute to in silico modeling using flow and mass cytometry readout as well as panel design for sample classification.
更多查看译文
关键词
AML,Classification,Flow Cytometry,Wilks’, Lambda,Stepwise Forward Selection,Machine Learning
AI 理解论文
溯源树
样例
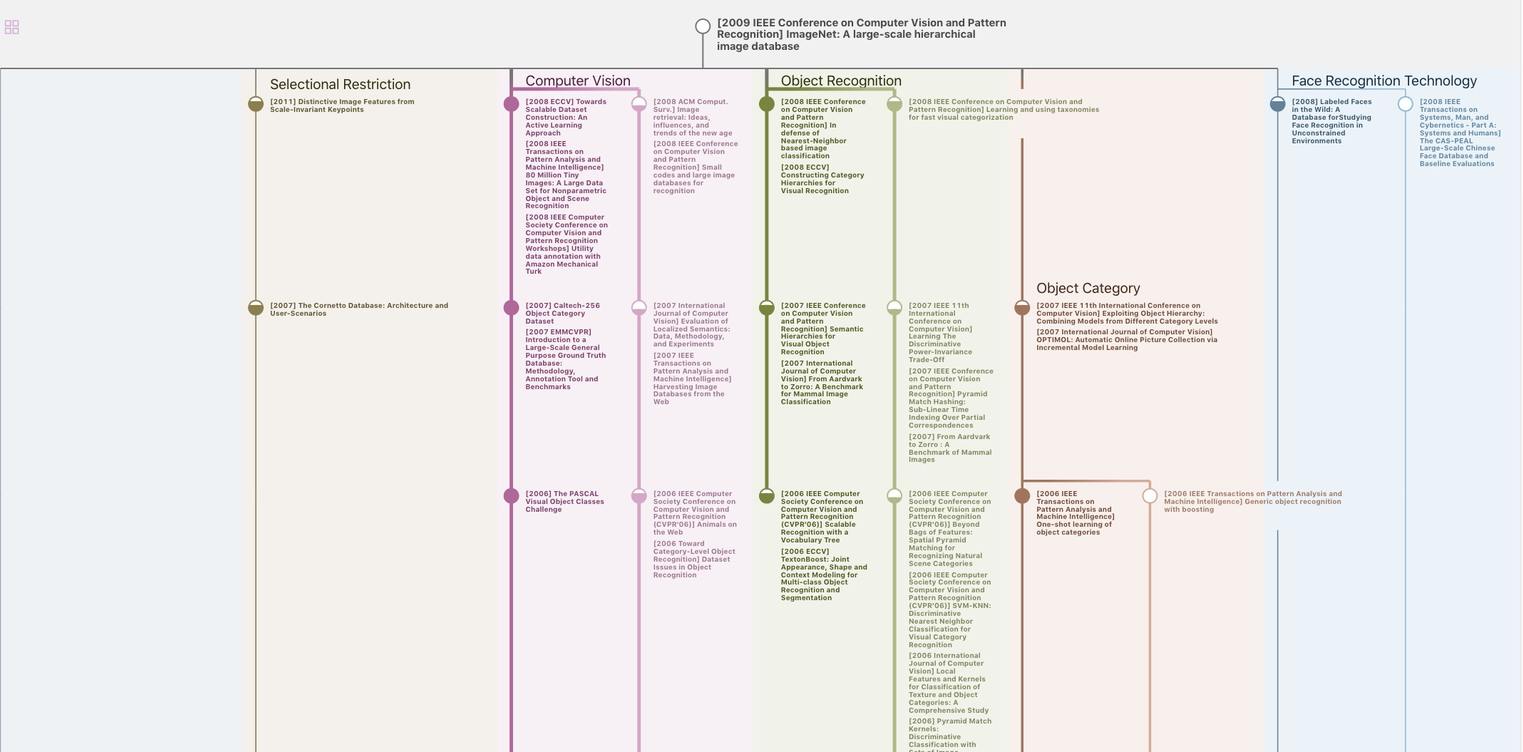
生成溯源树,研究论文发展脉络
Chat Paper
正在生成论文摘要