Sircs: Slope-Intercept-Residual Compression By Correlation Sequencing For Multi-Stream High Variation Data
DATABASE SYSTEMS FOR ADVANCED APPLICATIONS (DASFAA 2019), PT I(2019)
摘要
Multi-stream data with high variation is ubiquitous in the modern network systems. With the development of telecommunication technologies, robust data compression techniques are urged to be developed. In this paper, we humbly introduce a novel technique specifically for high variation signal data: SIRCS, which applies linear regression model for slope, intercept and residual decomposition of the multi data stream and combines the advanced tree mapping techniques. SIRCS inherits the advantages from the existing grouping compression algorithms, like GAMPS. With the newly invented correlation sorting techniques: the correlation tree mapping, SIRCS can practically improve the compression ratio by 13% from the traditional clustering mapping scheme. The application of the linear model decomposition can further facilitate the improvement of the algorithm performance from the stateof- art algorithms, with the RMSE decrease 4% and the compression time dramatically drop compared to the GAMPS. With the wide range of the error tolerance from 1% to 27%, SIRCS performs consistently better than all evaluated state-of-art algorithms regarding compression efficiency and accuracy.
更多查看译文
关键词
High variation data, Multi-signal compression, Correlation mapping, Linear regression model, Error detection
AI 理解论文
溯源树
样例
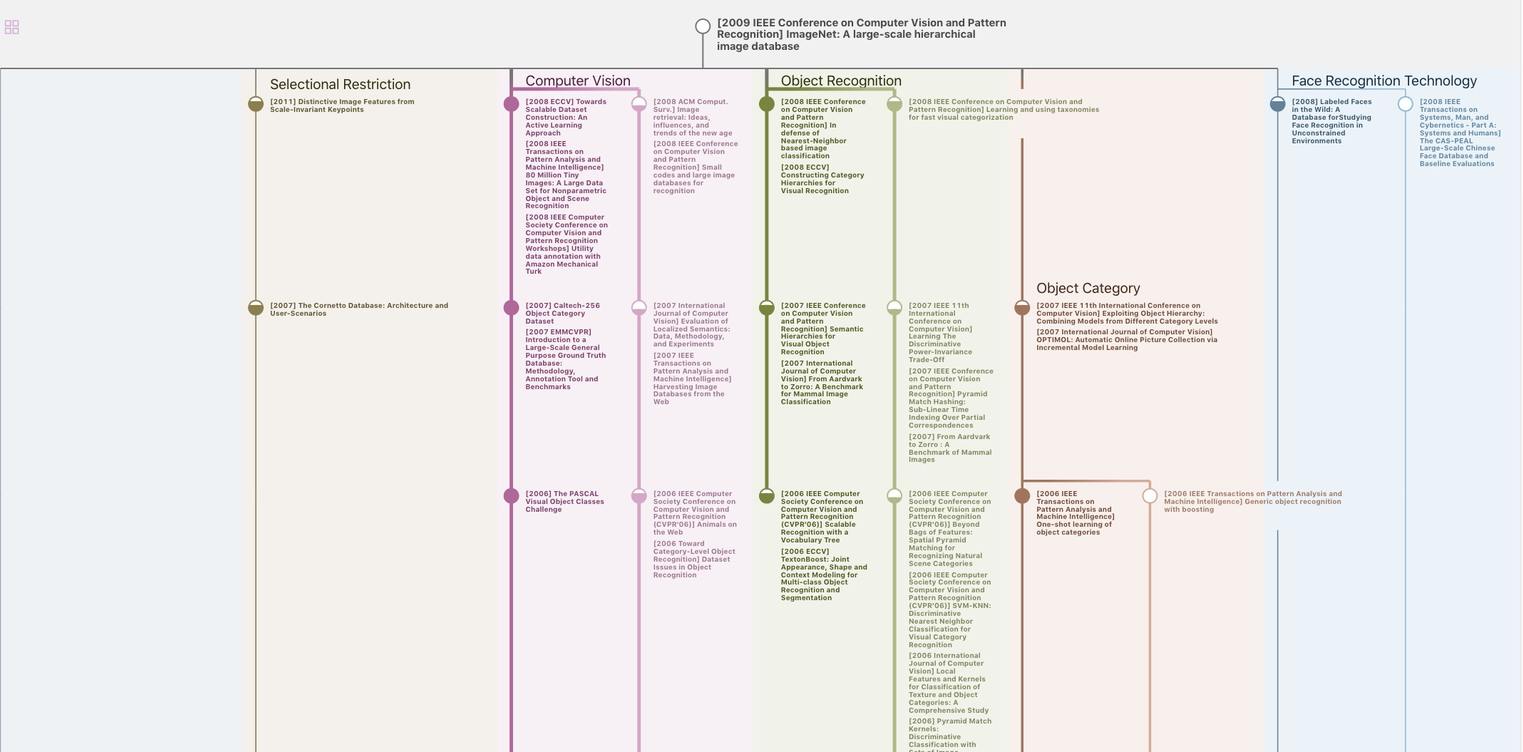
生成溯源树,研究论文发展脉络
Chat Paper
正在生成论文摘要