Effect of Time Intervals on K-nearest Neighbors Model for Short-term Traffic Flow Prediction
PROMET-TRAFFIC & TRANSPORTATION(2019)
摘要
The accuracy and reliability in predicting short-term traffic flow is important. The K-nearest neighbors (K-NN) approach has been widely used as a nonparametric model for traffic flow prediction. However, the reliability of the K-NN model results is unknown and the uncertainty of traffic flow point prediction needs to be quantified. To this end, we extended the K-NN approach by constructing the prediction interval associated with the point prediction. Recognizing the stochastic nature of traffic, time interval used to measure traffic flow rate is remarkably influential. In this paper, extensive tests have also been conducted after aggregating real traffic flow data into time intervals, ranging from 3 minutes to 30 minutes. The results show that the performance of traffic flow prediction can be improved when the time interval increases. More importantly, when the time interval is shorter than 10 minutes, K-NN can generate higher accuracy of the point prediction than the selected benchmark model. This finding suggests the K-NN model may be more appropriate for traffic flow point and interval prediction at a shorter time interval.
更多查看译文
关键词
short-term traffic flow forecasting,point prediction,prediction interval,K-nearest neighbors,seasonal autoregressive integrated moving average (SARIMA),generalized autoregressive conditional heteroscedasticity (GARCH)
AI 理解论文
溯源树
样例
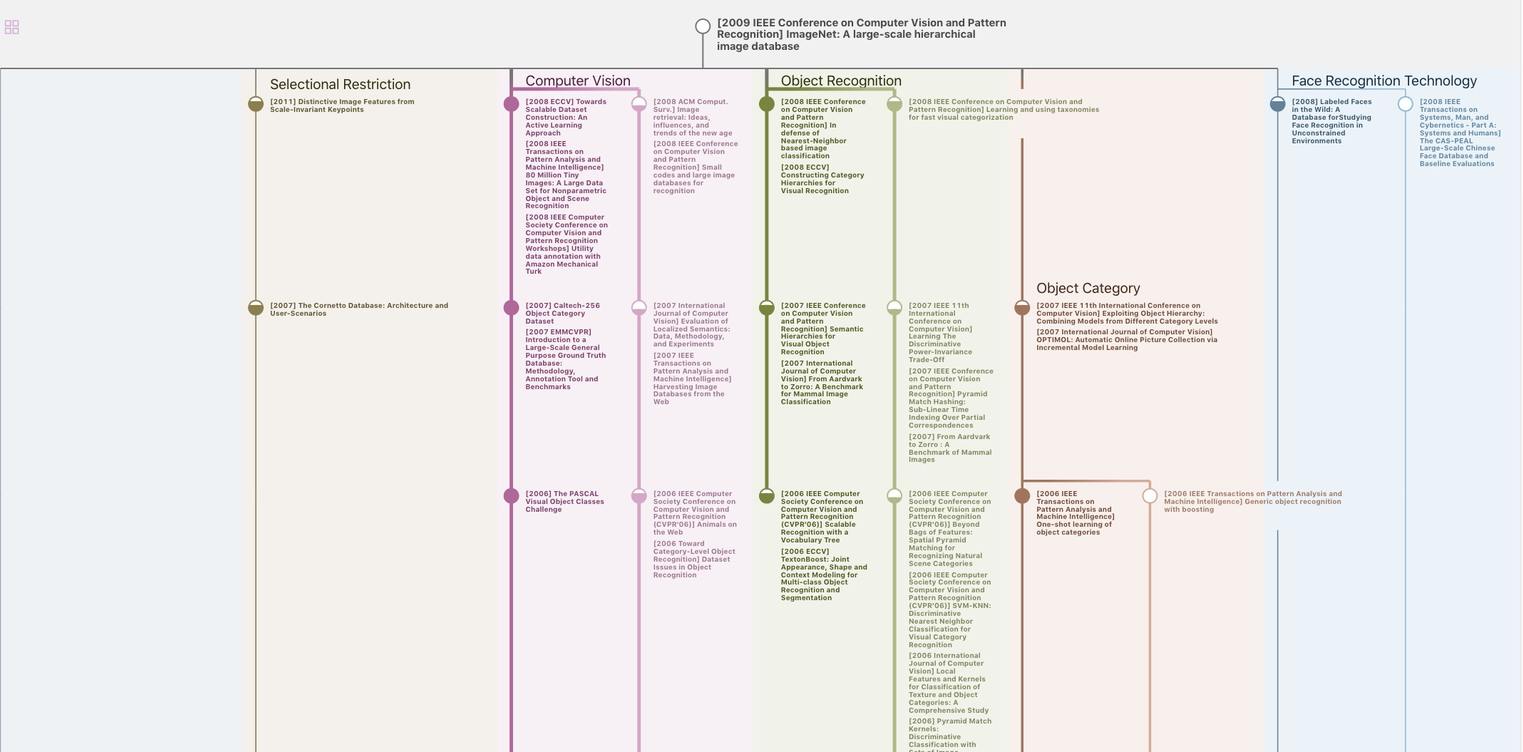
生成溯源树,研究论文发展脉络
Chat Paper
正在生成论文摘要