AdaCML: Adaptive Collaborative Metric Learning for Recommendation
DATABASE SYSTEMS FOR ADVANCED APPLICATIONS (DASFAA 2019), PT II(2019)
摘要
User preferences are dynamic and diverse in real world, while historical preference of a user may not be equally important as current preference when predicting future interests. As a result, learning the evolving user representation effectively becomes a critical problem in personalized recommendation. However, existing recommendation solutions often use a fixed user representation, which is not capable of modeling the complex interests of users. To this end, we propose a novel metric learning approach named Adaptive Collaborative Metric Learning (AdaCML) for recommendation. AdaCML employs a memory component and an attention mechanism to learn an adaptive user representation, which dynamically adapts to locally activated items. In this way, implicit relationships of user-item pairs can be better determined in the metric space and users’ interests can be modeled more accurately. Comprehensive experimental results demonstrate the effectiveness of AdaCML on two datasets, and show that AdaCML outperforms competitive baselines in terms of Precision, Recall, and Normalized Discounted Cumulative Gain (NDCG).
更多查看译文
关键词
Recommender systems,Attention mechanism,Metric learning
AI 理解论文
溯源树
样例
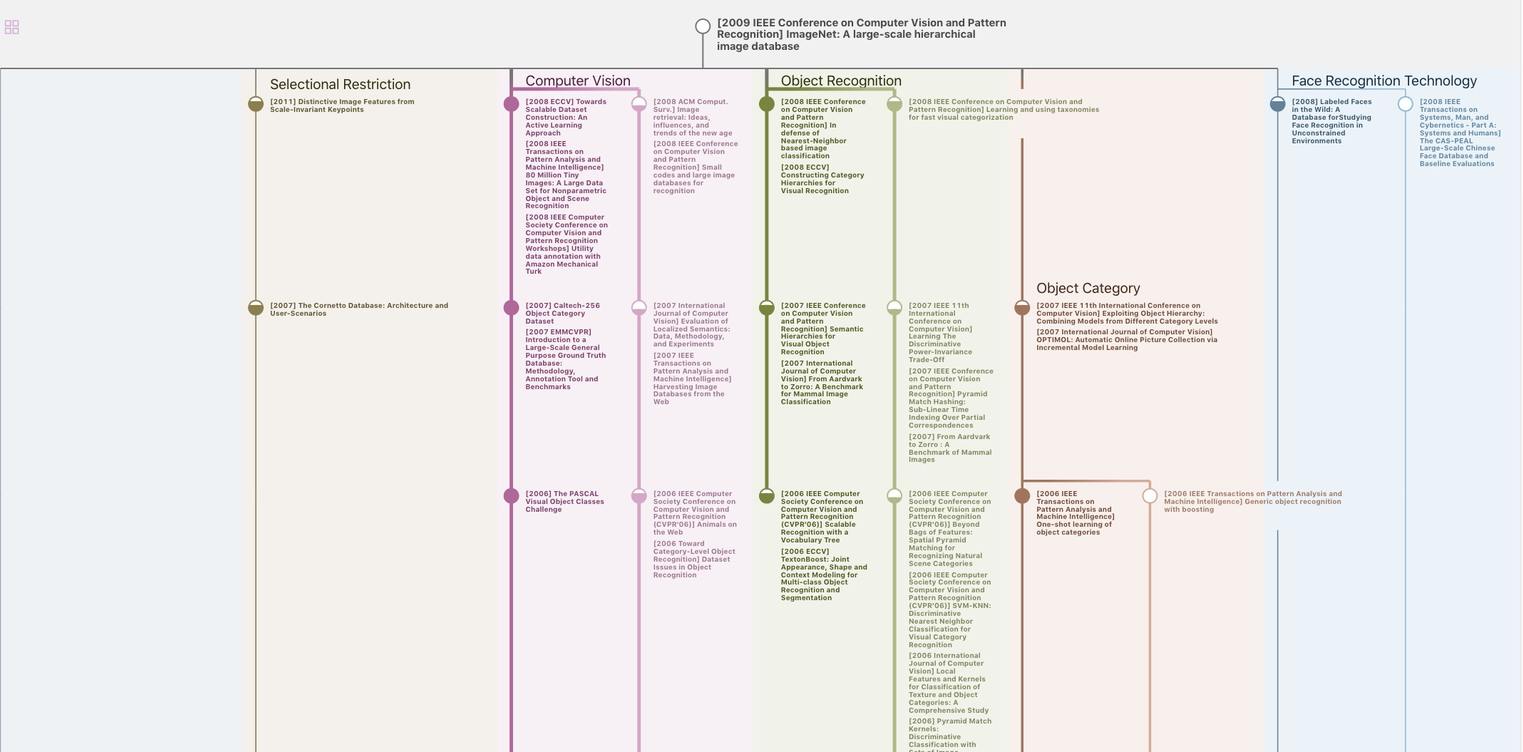
生成溯源树,研究论文发展脉络
Chat Paper
正在生成论文摘要