Fog-embedded Deep Learning for the Internet of Things
IEEE Transactions on Industrial Informatics(2019)
摘要
In current deep learning models, centralized architecture forces participants to pool their data to the central Cloud to train a global model, while distributed architecture requires a parameter server to mediate the training process. However, privacy issues, response delays, and computation and communication bottlenecks prevent these architectures from working well at the scale of Internet of Things devices. To counter these problems, in this paper we build a Fog-embedded privacy-preserving deep learning framework (FPPDL), which moves computation from the centralized Cloud to Fog nodes near the end devices. The experimental results on benchmark image datasets under different settings demonstrate that FPPDL achieves comparable accuracy to the centralized stochastic gradient descent (SGD) framework, and delivers better accuracy than the standalone SGD framework. Our evaluations also show that both computation and communication cost are greatly reduced by FPPDL, hence achieving the desired tradeoff between privacy and performance.
更多查看译文
关键词
Computational modeling,Deep learning,Servers,Cloud computing,Privacy,Data models,Training
AI 理解论文
溯源树
样例
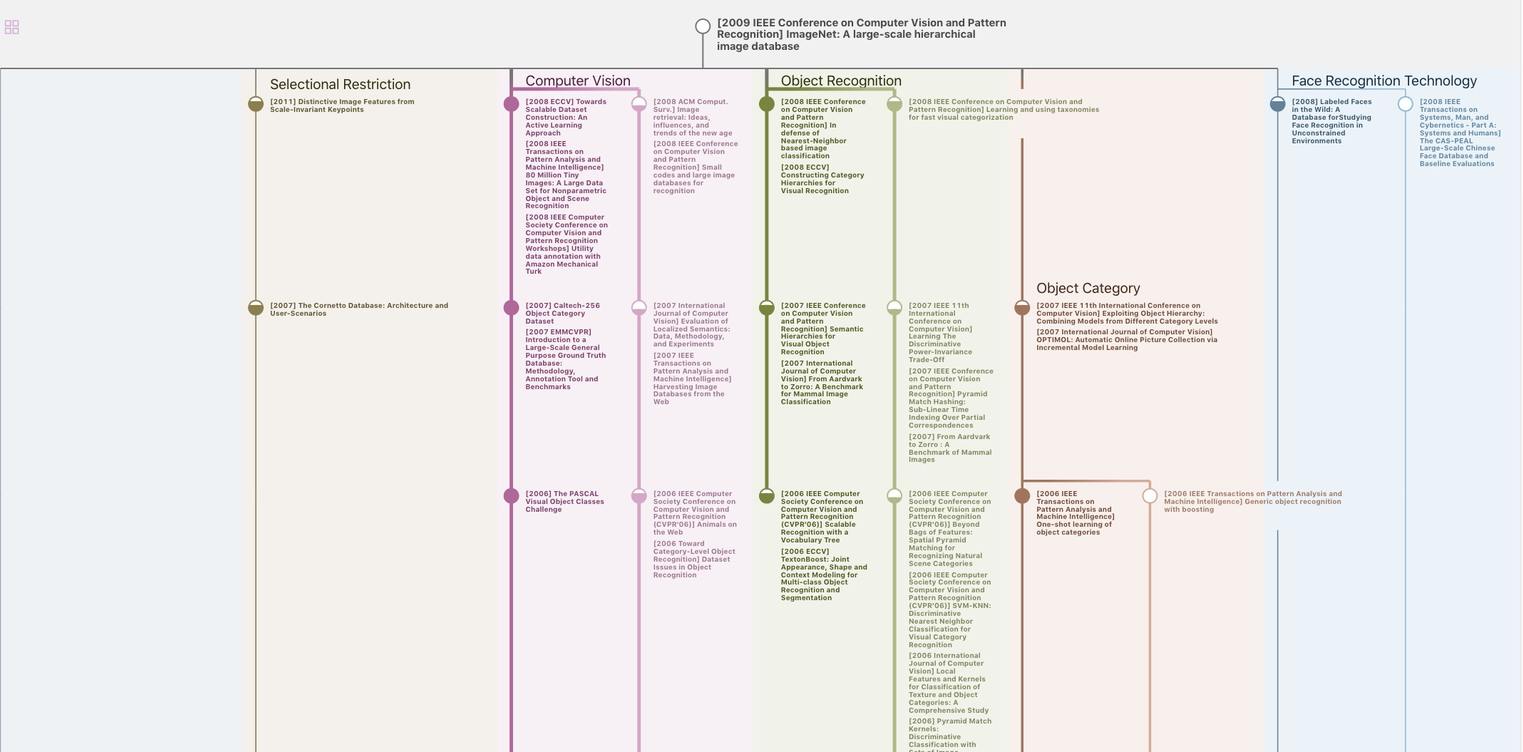
生成溯源树,研究论文发展脉络
Chat Paper
正在生成论文摘要