Data augmentation of random grid-hiding for video object segmentation
Multimedia Tools and Applications(2019)
摘要
Video object segmentation is an important field in computer vision. However, the challenges in video object segmentation such as background clutter, occlusion and edge ambiguity cannot be avoided. In addition, existing labeled video object segmentation datasets are limited in size, which prevents CNN models from reaching their full generalization capabilities. In this paper, we propose a novel approach, called random grid-hiding (RGH), to perform data augmentation. We divide the training image into several rectangular regions and hide some regions randomly during model training. Thus, the convolutional neural network automatically focuses on the discriminative parts of the image. When the most discriminative part of the image is hidden, it compels the network focus on the other related parts of the image. Further, occlusion images are randomly generated in various levels. More features can be obtained by random grid-hiding, which can effectively reduce the risk of overfitting. Our approach is an effective extension of the data augmentation (such as random cropping and random flipping), and leads to improved accuracy in the task of the video object segmentation method on DAVIS dataset. Our experimental results show that the proposed method is a stable and effective method for data augmentation.
更多查看译文
关键词
Video object segmentation, Random grid-hiding, Data augmentation, Convolutional neural network
AI 理解论文
溯源树
样例
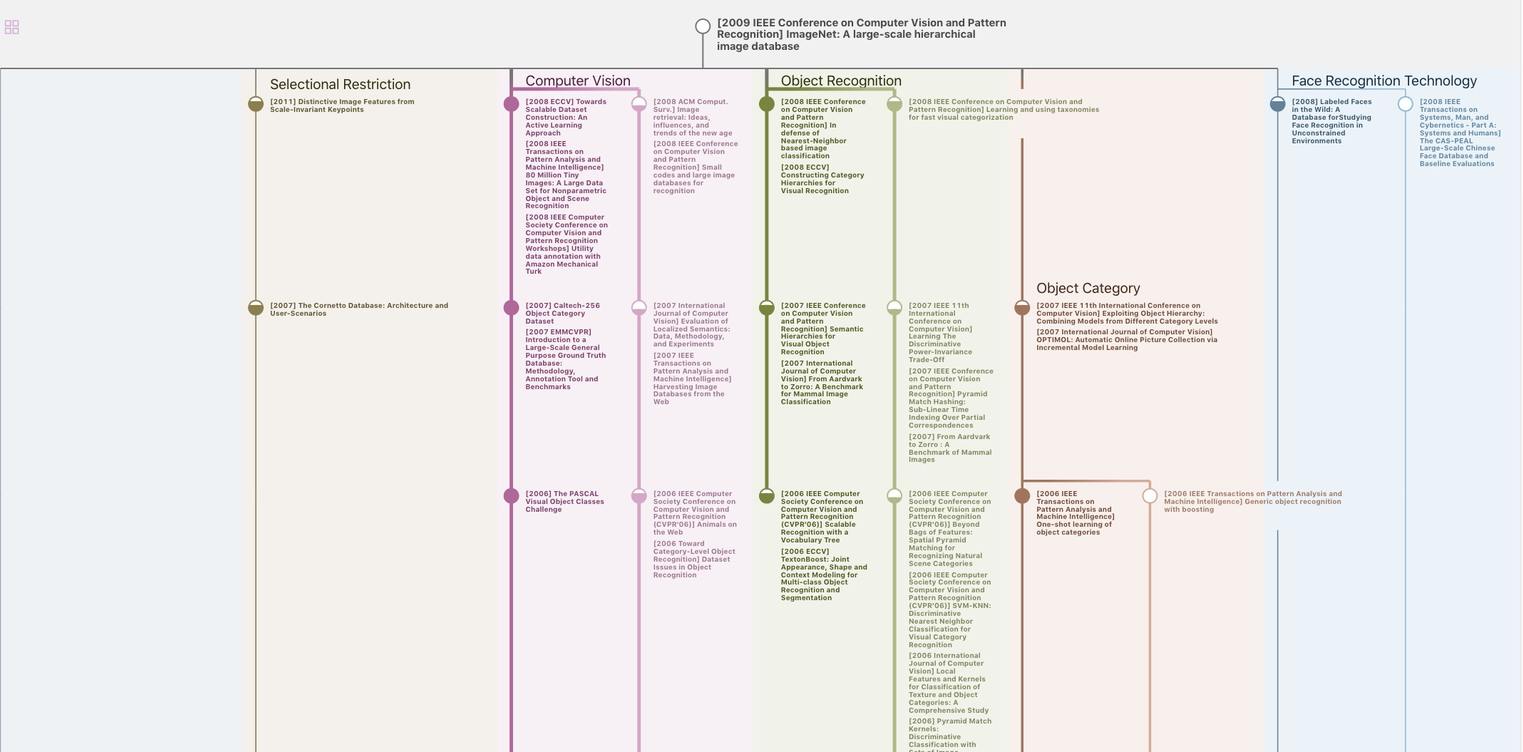
生成溯源树,研究论文发展脉络
Chat Paper
正在生成论文摘要