Sparse Estimator With $\ell_0$ -Norm Constraint Kernel Maximum-Correntropy-Criterion
IEEE Transactions on Circuits and Systems Ii-express Briefs(2020)
摘要
The kernel maximum-correntropy-criterion (KMCC) algorithm with kernel learning outperforms that with constant kernel, in terms of estimate accuracy and convergence. However, KMCC has limited performance when it is used for sparse system identification since it cannot exploit the sparse structure. This brief proposes
$\ell _{0}$
-norm constraint KMCC (KMCC-L0) algorithm to improve the estimate accuracy and the convergence rate of KMCC method. Specifically, an approximated
$\ell _{0}$
-norm constraint is integrated into KMCC cost function. Then we derive its iterative optimization process via stochastic gradient method. Furthermore, the analysis including parameter choice, computational complexity, and steady-state misalignment are provided in this brief. The proposed KMCC-L0 method is used for identifying and tracking for unknown sparse systems. The simulation results confirm its superior performance.
更多查看译文
关键词
Kernel,Convergence,Steady-state,Circuits and systems,Cost function,Linear programming,Approximation algorithms
AI 理解论文
溯源树
样例
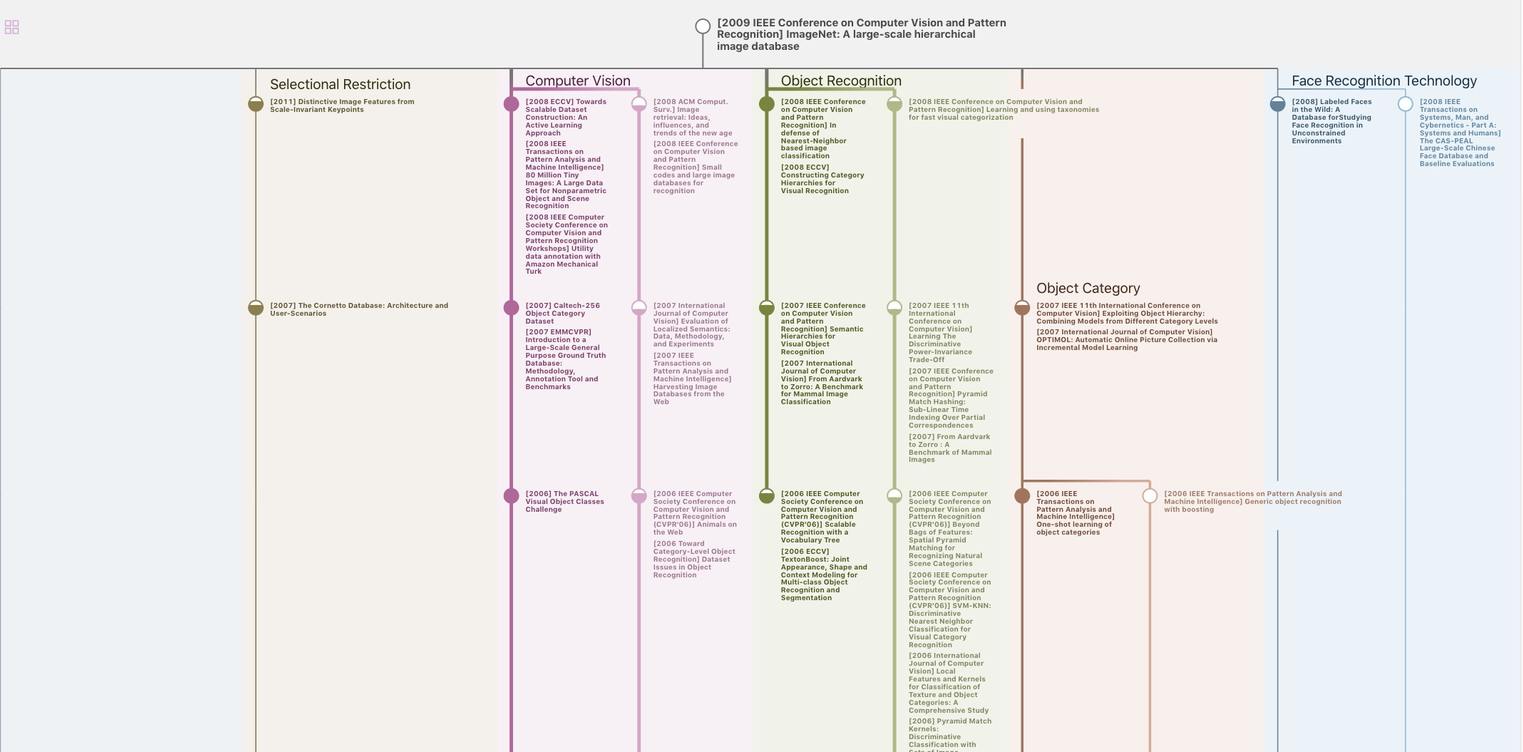
生成溯源树,研究论文发展脉络
Chat Paper
正在生成论文摘要