L 1 -norm loss-based projection twin support vector machine for binary classification
Soft Computing(2019)
摘要
This paper presents a L 1 -norm loss-based projection twin support vector machine (L1LPTSVM) for binary classification. In the pair of optimization problems of L1LPTSVM, L 1 -norm-based losses are considered for two classes, which leads to two different dual problems with projection twin support vector machine (PTSVM). Compared with PTSVM, L1LPTSVM has two main advantages: first, the dual problems of L1LPTSVM avoid the complex calculation of inverse matrices in the training process, indicating that L1LPTSVM can be solved efficiently using some SVM-type training algorithms. Second, similar to the traditional SVM, L1LPTSVM has an unified form in the linear and nonlinear cases. In addition, a density-dependent quantization scheme for sparse representation is used as the data preprocessing unit attached to L1LPTSVM, which makes L1LPTSVM be more suitable for large-scale problems. Extensive experimental results on several artificial and benchmark data sets show the effectiveness of the proposed method.
更多查看译文
关键词
Projection twin support vector,L1-norm loss,Inverse matrix,Density dependent quantization scheme,Large-scale data sets
AI 理解论文
溯源树
样例
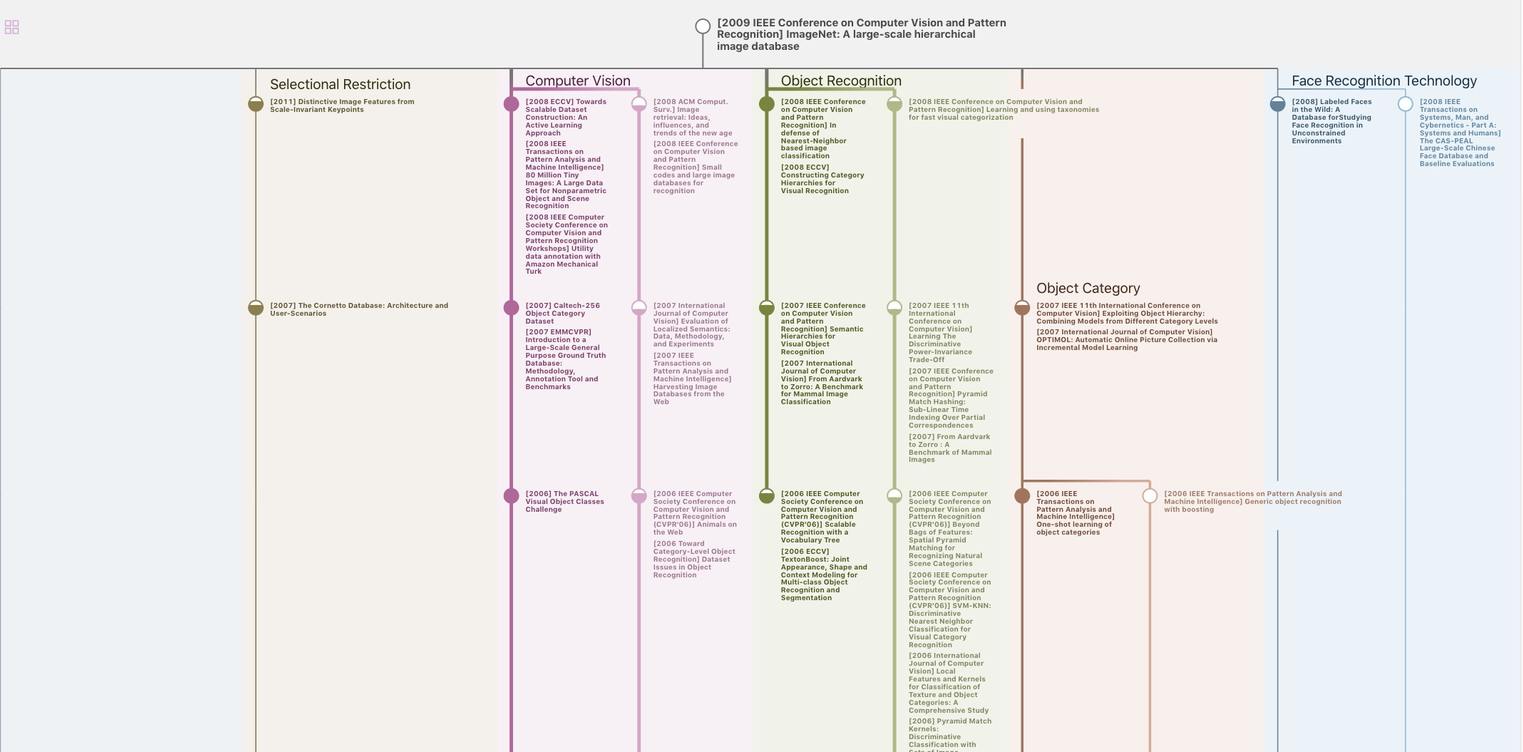
生成溯源树,研究论文发展脉络
Chat Paper
正在生成论文摘要