Density Matrix Based Preference Evolution Networks For E-Commerce Recommendation
DATABASE SYSTEMS FOR ADVANCED APPLICATIONS (DASFAA 2019), PT II(2019)
Abstract
In e-commerce platforms, mining temporal characteristics in user behavior is conducive to recommend the right product for the user at the right time. Recently, recurrent neural networks (RNNs) based methods have achieved profitable performance in exploring temporal features, however, in complex e-commerce scenarios, user preferences changing over time have not been fully exploited. In order to fill the gap, we propose a novel representation for user preferences with the inspiration of a quantum concept, density matrix. It encodes a mixture of item subspaces and represents distribution of user preferences at one time stamp. Further, such a representation and RNNs are combined to form our proposed Density Matrix based Preference Evolution Networks (DMPENs). Experiments on Amazon datasets as well as real-world e-commerce datasets demonstrate the effectiveness of the proposed methods, which achieve rapid convergence and superior performance compared with the state-of-the-art methods in terms of AUC and accuracy.
MoreTranslated text
Key words
E-commerce recommendation, Recurrent neural networks, Density matrix
AI Read Science
Must-Reading Tree
Example
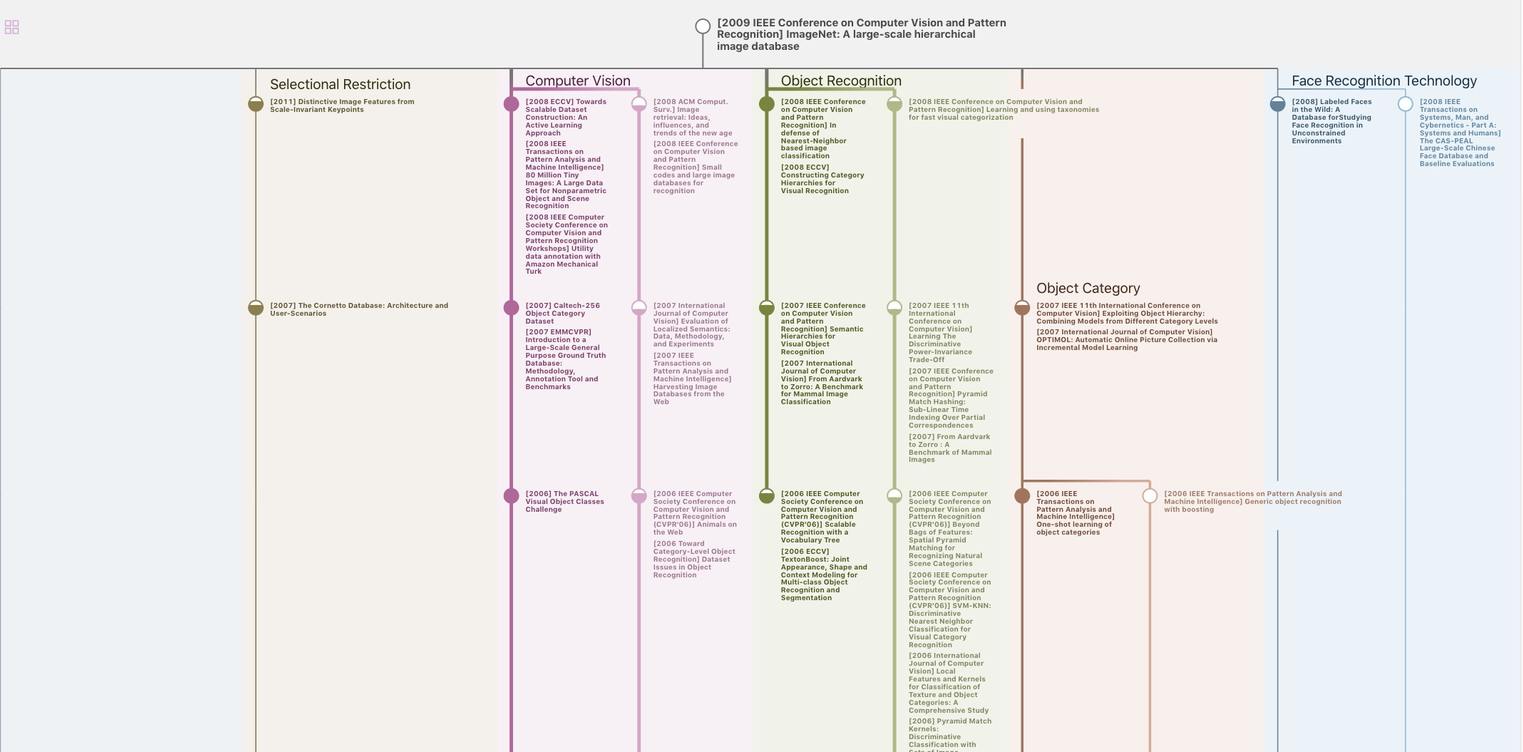
Generate MRT to find the research sequence of this paper
Chat Paper
Summary is being generated by the instructions you defined