Synaptic weight decay with selective consolidation enables fast learning without catastrophic forgetting
bioRxiv(2019)
摘要
Learning can interfere with pre-existing memories that in classical neural networks may lead to catastrophic forgetting. Different from these networks, biological synapses show an early decay of long-term potentiation, combined with a tag u0026 capture mechanism for selective consolidation. We present a 2-component synaptic plasticity model that, by an early decay and a tag u0026 capture mechanism, enables context-dependent fast learning without catastrophic forgetting. For reinforcement learning in multiple contexts, the fast learning combined with an early weight decay maximizes the expected reward while minimizing interferences between subsequent contexts. Fast learning, enabled by a highly plastic weight component, improves performance for a given context. Between contexts this plastic component decays to prevent interference, but selective consolidation into a stable component protects old memories. As a downside of these mechanisms, learning is hampered when consolidation is triggered prematurely by interleaving easy and difficult tasks, consistent with human psychophysical experiments.
更多查看译文
AI 理解论文
溯源树
样例
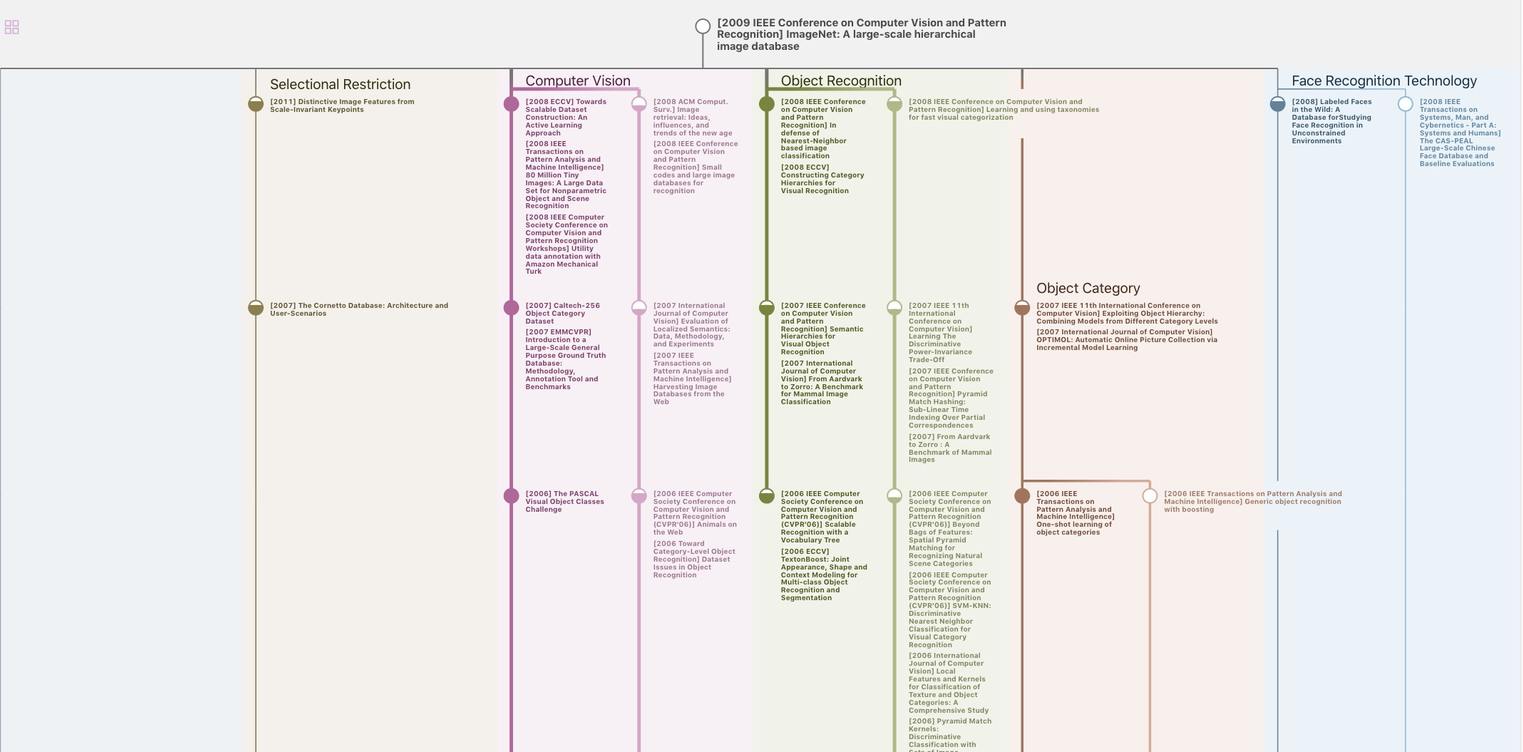
生成溯源树,研究论文发展脉络
Chat Paper
正在生成论文摘要