Joint Optimization Of Neural Network-Based Wpe Dereverberation And Acoustic Model For Robust Online Asr
2019 IEEE INTERNATIONAL CONFERENCE ON ACOUSTICS, SPEECH AND SIGNAL PROCESSING (ICASSP)(2019)
摘要
Signal dereverberation using the Weighted Prediction Error ( WPE) method has been proven to be an effective means to raise the accuracy of far-field speech recognition. First proposed as an iterative algorithm, follow-up works have reformulated it as a recursive least squares algorithm and therefore enabled its use in online applications. For this algorithm, the estimation of the power spectral density ( PSD) of the anechoic signal plays an important role and strongly influences its performance. Recently, we showed that using a neural network PSD estimator leads to improved performance for online automatic speech recognition. This, however, comes at a price. To train the network, we require parallel data, i. e., utterances simultaneously available in clean and reverberated form. Here we propose to overcome this limitation by training the network jointly with the acoustic model of the speech recognizer. To be specific, the gradients computed from the cross-entropy loss between the target senone sequence and the acoustic model network output is backpropagated through the complex-valued dereverberation filter estimation to the neural network for PSD estimation. Evaluation on two databases demonstrates improved performance for online processing scenarios while imposing fewer requirements on the available training data and thus widening the range of applications.
更多查看译文
关键词
dereverberation, speech enhancement, joint optimization, robust ASR
AI 理解论文
溯源树
样例
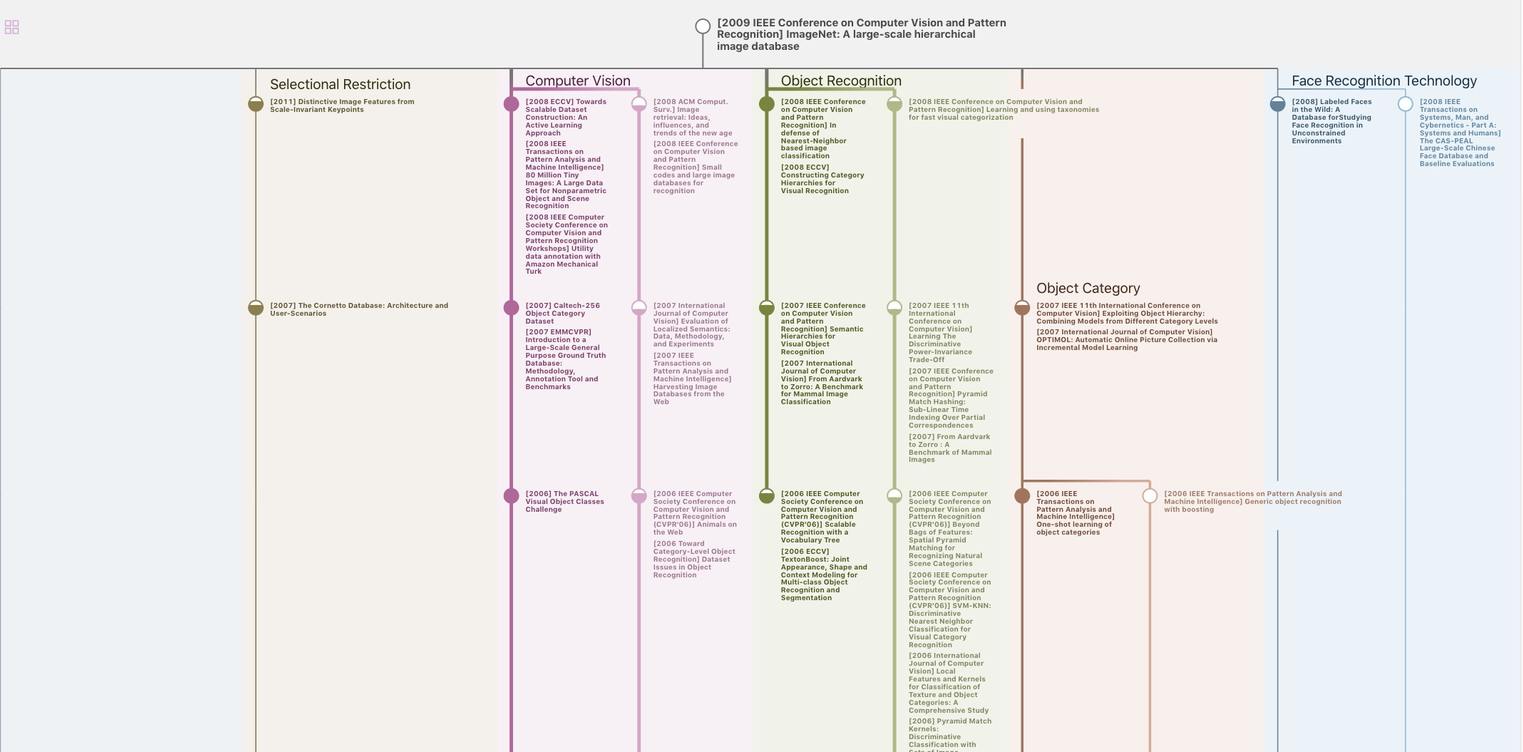
生成溯源树,研究论文发展脉络
Chat Paper
正在生成论文摘要