The number sense is an emergent property of a deep convolutional neural network trained for object recognition
bioRxiv(2019)
摘要
Humans and many non-human animals have the sense, an ability to estimate the number of items in a set without counting. This innate sense of number is hypothesized to provide a foundation for more complex numerical and mathematical concepts. Here I investigated whether we also share the number sense with a deep convolutional neural network (DCNN) trained for object recognition. These in silico networks have revolutionized machine learning over the last seven years, allowing computers to reach human-level performance on object recognition tasks for the first time. Their architecture is based on the structure of mammalian visual cortex, and after they are trained, they provide a highly predictive model of responses in primate visual cortex, suggesting deep homologies. I found that the DCNN demonstrates three key hallmarks of the number sense. First, some of the units comprising the DCNN (analogous to neurons) are numerosity-selective in that their activity varies with the number but not the size or spacing of elements in a visual array. Second, DCNN discrimination of numerosities very closely follows the empirically validated psychometric functions describing human and animal performance on an ordinal comparison task. Third, numerosities are properly ordered in the representational space of the DCNN. Because the DCNN was not trained to enumerate, I conclude that the number sense is an emergent property of the network, the result of some combination of the network architecture and the constraint to develop the complex representational structure necessary for object recognition. By analogy I conclude that the number sense in animals was not necessarily the result of direct selective pressure to enumerate but might have come for free with the evolution of a complex visual system that evolved to identify objects and scenes in the real world.
更多查看译文
AI 理解论文
溯源树
样例
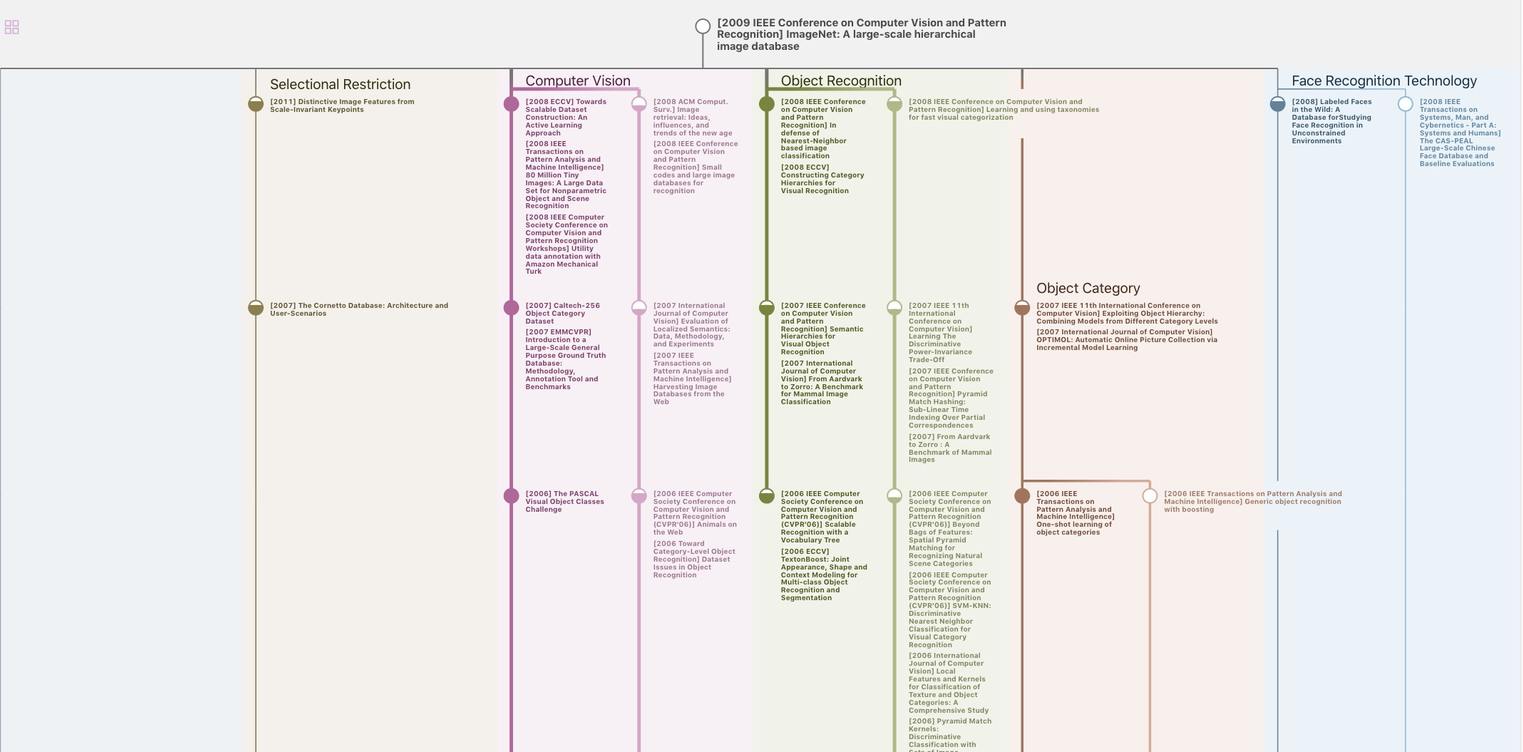
生成溯源树,研究论文发展脉络
Chat Paper
正在生成论文摘要