A Lstm And Cnn Based Assemble Neural Network Framework For Arrhythmias Classification
2019 IEEE INTERNATIONAL CONFERENCE ON ACOUSTICS, SPEECH AND SIGNAL PROCESSING (ICASSP)(2019)
摘要
This paper puts forward a LSTM and CNN based assemble neural network framework to distinguish different types of arrhythmias by integrating stacked bidirectional long shot-term memory (SB-LSTM) network and two-dimensional convolutional neural network (TD-CNN). Particularly, SB-LSTM is used to mine the long-term dependencies contained in electrocardiogram (ECG) from two directions to model the overall variation trends of ECG, while TD-CNN aims at extracting local information of ECG to characterize the local features of ECG. Moreover, we design an ensemble empirical mode decomposition (EEMD) based signal decomposition layer and a support vector machine based intermediate result fusion layer, by which ECG can be analyzed more effectively, and the final classification results can be more accurate and robust. Experimental results on public INCART arrhythmia database show that our model surpasses three state-of-the-art methods, and obtains 99.1% of accuracy, 99.3% of sensitivity and 98.5% of specificity.
更多查看译文
关键词
Arrhythmia Classification, LSTM, CNN, ECG, Ensemble Empirical Mode Decomposition (EEMD)
AI 理解论文
溯源树
样例
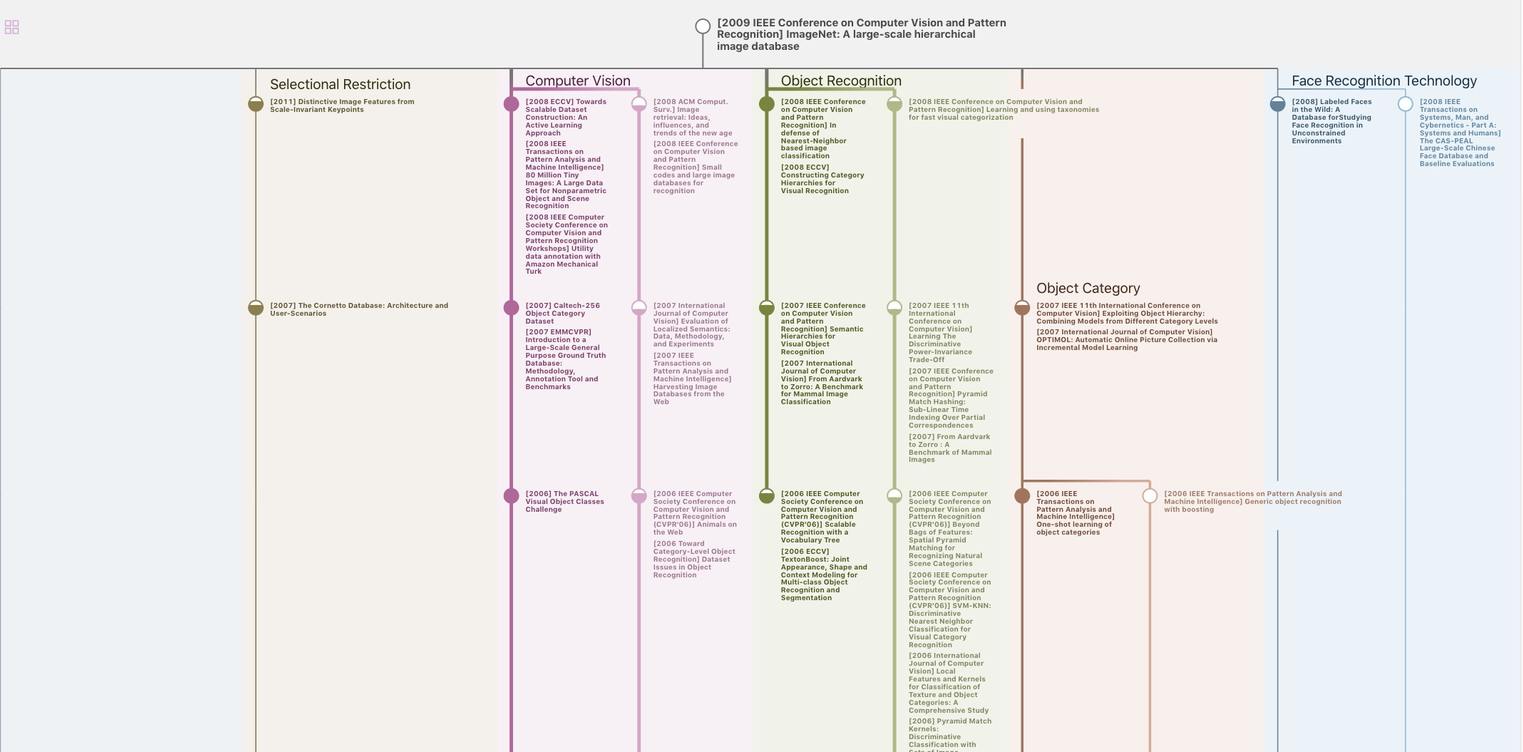
生成溯源树,研究论文发展脉络
Chat Paper
正在生成论文摘要