Graph Regularized Nonnegative Tucker Decomposition For Tensor Data Representation
2019 IEEE INTERNATIONAL CONFERENCE ON ACOUSTICS, SPEECH AND SIGNAL PROCESSING (ICASSP)(2019)
摘要
Nonnegative Tucker Decomposition (NTD) is one of the most popular technique for feature extraction and representation from nonnegative tensor data with preserving internal structure information. From the perspective of geometry, highdimensional data are usually drawn in low-dimensional submanifold of the ambient space. In this paper, we propose a novel Graph reguralized Nonnegative Tucker Decomposition (GNTD) method which is able to extract the low-dimensional parts-based representation and preserve the geometrical information simultaneously from high-dimensional tensor data. We also present an effictive algorithm to solve the proposed GNTD model. Experimental results demonstrate the effectiveness and high efficiency of the proposed GNTD method.
更多查看译文
关键词
Mannifold learning, nonnegative tensor, Tucker decomposition, dimensionality reduction, clustering
AI 理解论文
溯源树
样例
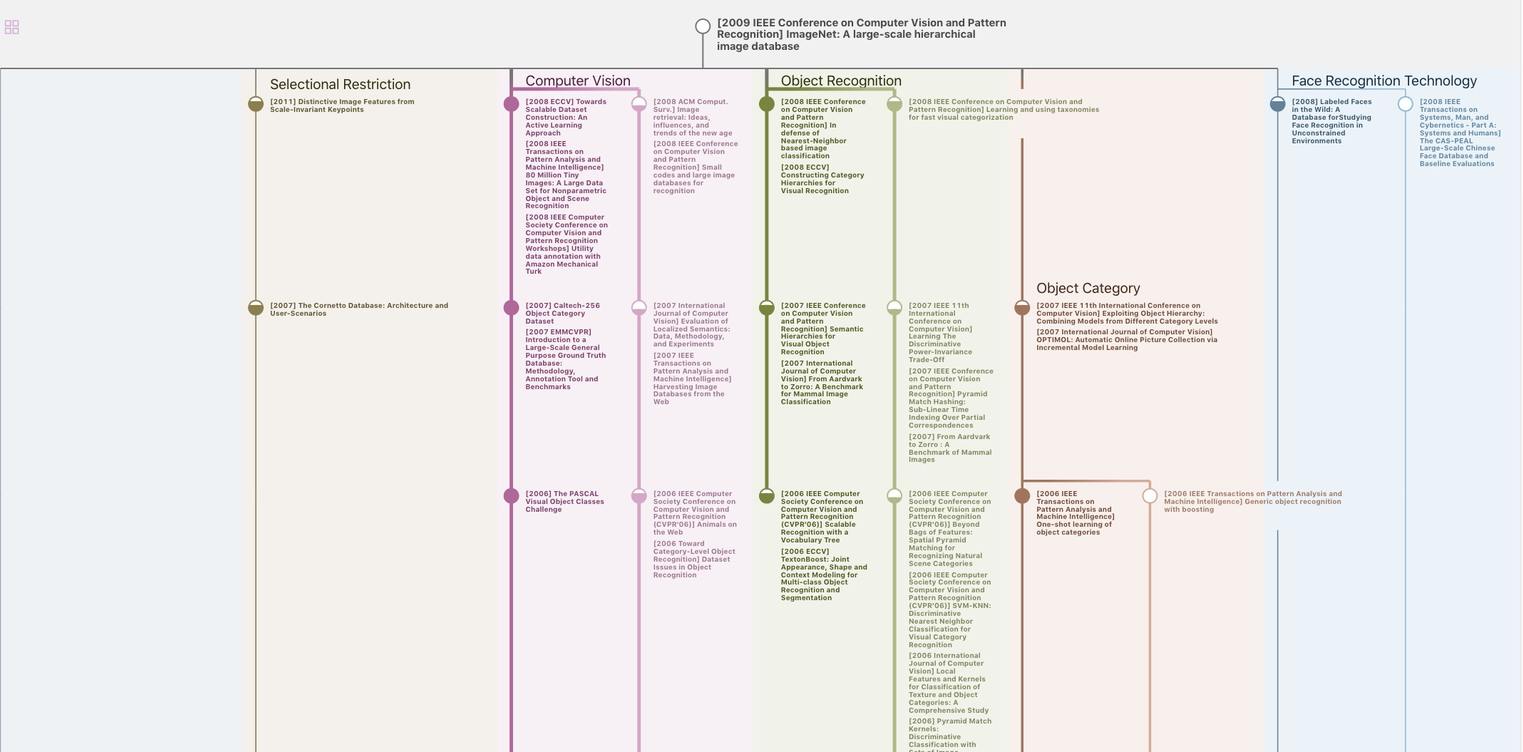
生成溯源树,研究论文发展脉络
Chat Paper
正在生成论文摘要