Improving The Prediction Of Therapist Behaviors In Addiction Counseling By Exploiting Class Confusions
2019 IEEE INTERNATIONAL CONFERENCE ON ACOUSTICS, SPEECH AND SIGNAL PROCESSING (ICASSP)(2019)
摘要
In this work we address the problem of joint prosodic and lexical behavioral annotation for addiction counseling. We expand on past work that employed Recurrent Neural Networks ( RNNs) on multimodal features by grouping and classifying subsets of classes. We propose two implementations: One is hierarchical classification, which uses the behavior confusion matrix to cluster similar classes and makes the prediction based on a tree structure. The second is a graph-based method which uses the result of the original classification just to find a certain subset of the most probable candidate classes, where the candidate sets of different predicted classes are determined by the class confusions. We make a second prediction with simpler classifier to discriminate the candidates. The evaluation shows that the strict hierarchical approach degrades performance, likely due to error propagation, while the graph-based hierarchy provides significant gains.
更多查看译文
关键词
behavioral signal processing, multimodal, class confusions, class hierarchy, graph-based
AI 理解论文
溯源树
样例
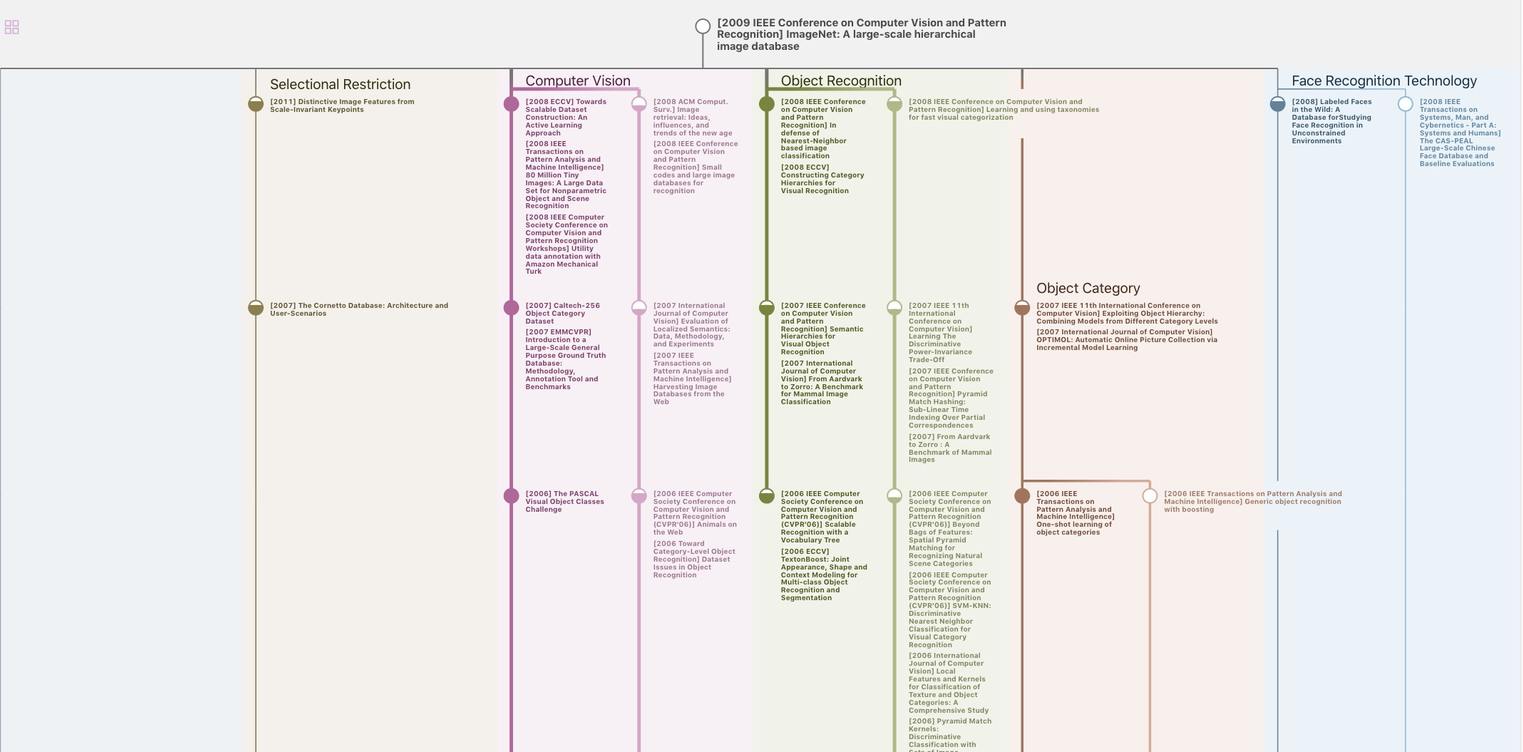
生成溯源树,研究论文发展脉络
Chat Paper
正在生成论文摘要