Reinforcement Learning With Safe Exploration For Network Security
2019 IEEE INTERNATIONAL CONFERENCE ON ACOUSTICS, SPEECH AND SIGNAL PROCESSING (ICASSP)(2019)
摘要
Safe reinforcement learning is important for the safety critical applications especially network security, as the exploration of some dangerous actions can result in huge short-term losses such as network failure or large scale privacy leakage. In this paper, we propose a reinforcement learning algorithm with safe exploration and uses transfer learning to reduce the initial random exploration. A blacklist is maintained to record the most dangerous state-action pairs as a safety constraint. A safe deep reinforcement learning version uses a convolutional neural network to estimate the risk levels and thus further improves the safety of the exploration and accelerates the learning speed for the learning agent. As a case study, the proposed reinforcement learning with safe exploration is applied in the anti-jamming robot communications. Experimental results show that the proposed algorithms can improve the jamming resistance of the robot and reduce the outage rate to enter the most dangerous states compared with the benchmark algorithms.
更多查看译文
关键词
reinforcement learning, safe exploration, deep reinforcement learning, network security
AI 理解论文
溯源树
样例
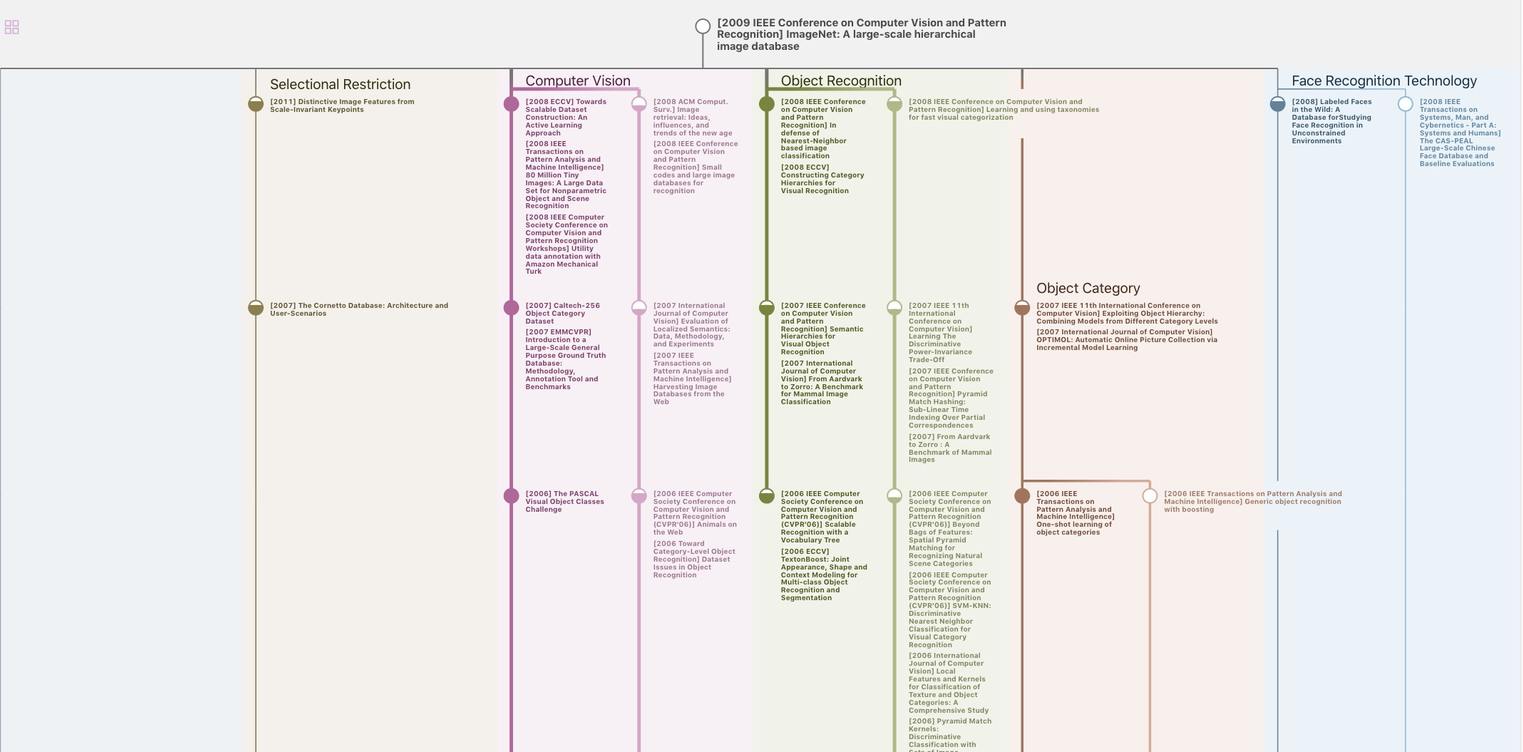
生成溯源树,研究论文发展脉络
Chat Paper
正在生成论文摘要