Neural Approaches To Automated Speech Scoring Of Monologue And Dialogue Responses
2019 IEEE INTERNATIONAL CONFERENCE ON ACOUSTICS, SPEECH AND SIGNAL PROCESSING (ICASSP)(2019)
摘要
We present Neural Network (NN) approaches to the automated assessment of non-native spontaneous speech in a monologic task and a simulated dialogic task. Three attention-based Bidirectional Long Short-Term Memory (BLSTM) Recurrent Neural Networks (RNN) are employed to learn three dimensions (i.e., delivery, language use, and content) of scoring rubrics for the spoken responses. The prompts or turn history information are encoded to low-dimensional vectors by either a BLSTM-RNN or an end-to-end memory network (MemN2N) and used as the conditions of the inputs of the NN for rating the subscore of content. The three subscores are fused together to generate a holistic score. The experimental results show that our approaches significantly outperform the conventional approaches to speech scoring and the correlations of automatically predicted scores with the reference human scores are higher than human-human agreement levels for both tasks.
更多查看译文
关键词
automated speech scoring, LSTM, RNN, attention, end-to-end memory networks
AI 理解论文
溯源树
样例
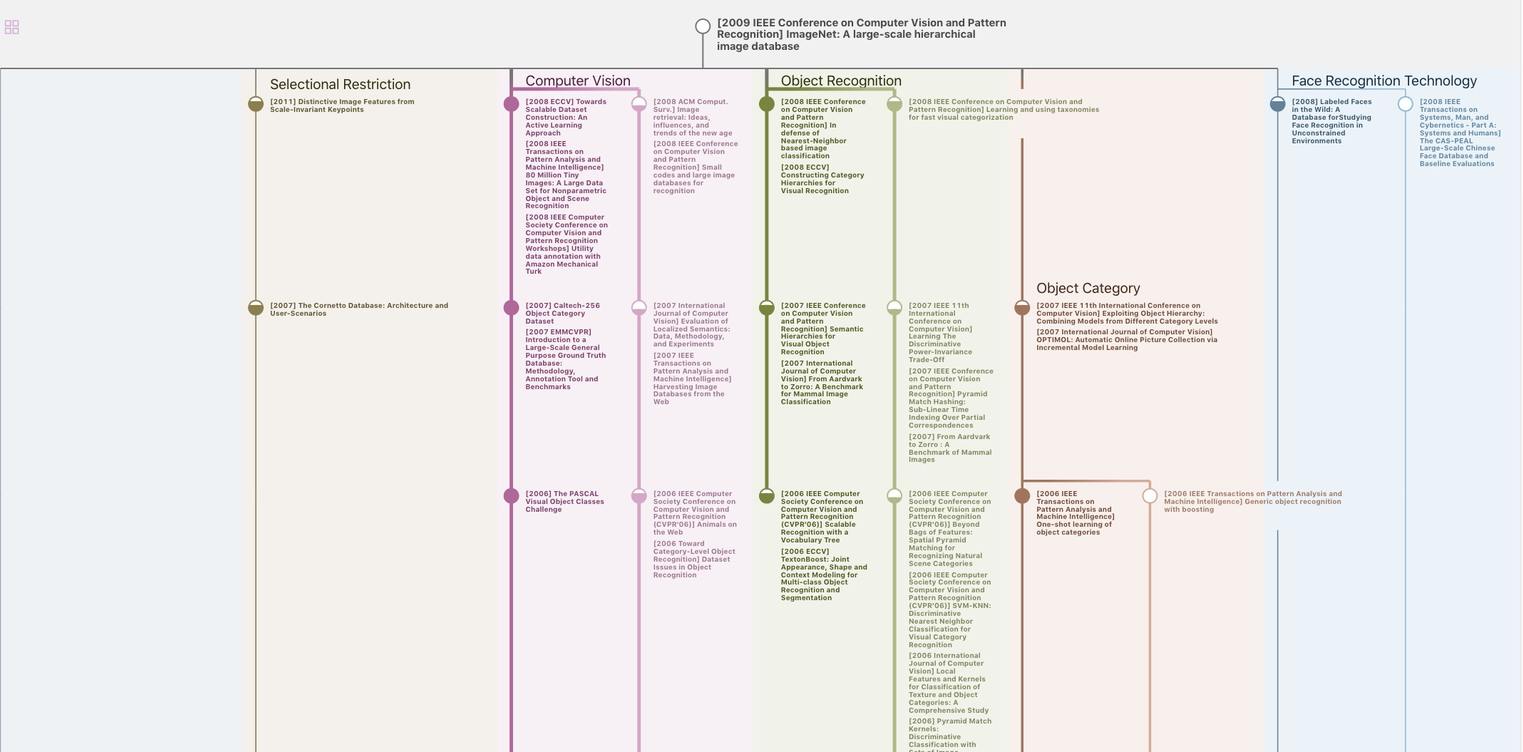
生成溯源树,研究论文发展脉络
Chat Paper
正在生成论文摘要