Fast and Communication-efficient Distributed Pca
2019 IEEE INTERNATIONAL CONFERENCE ON ACOUSTICS, SPEECH AND SIGNAL PROCESSING (ICASSP)(2019)
摘要
This paper focuses on principal components analysis (PCA), which involves estimating the principal subspace of a data covariance matrix, in the age of big data. Massively large datasets often require storage across multiple machines, which precludes the use of cen-tralized PCA solutions. While a number of distributed solutions to the PCA problem have been proposed recently, convergence guarantees and/or communications overhead of these solutions remain a concern. With an eye towards communications efficiency, this paper introduces two variants of a distributed PCA algorithm termed distributed Sanger's algorithm (DSA). Principal subspace estimation using both variants of DSA is communication efficient because of its one time-scale nature. In addition, theoretical guarantees are provided for the asymptotic convergence of basic DSA to the principal subspace, while its "accelerated" variant is numerically shown to have faster convergence than the state-of-the-art.
更多查看译文
关键词
Distributed data, decentralized learning, orthogonal iteration, principal component analysis, Sanger's algorithm
AI 理解论文
溯源树
样例
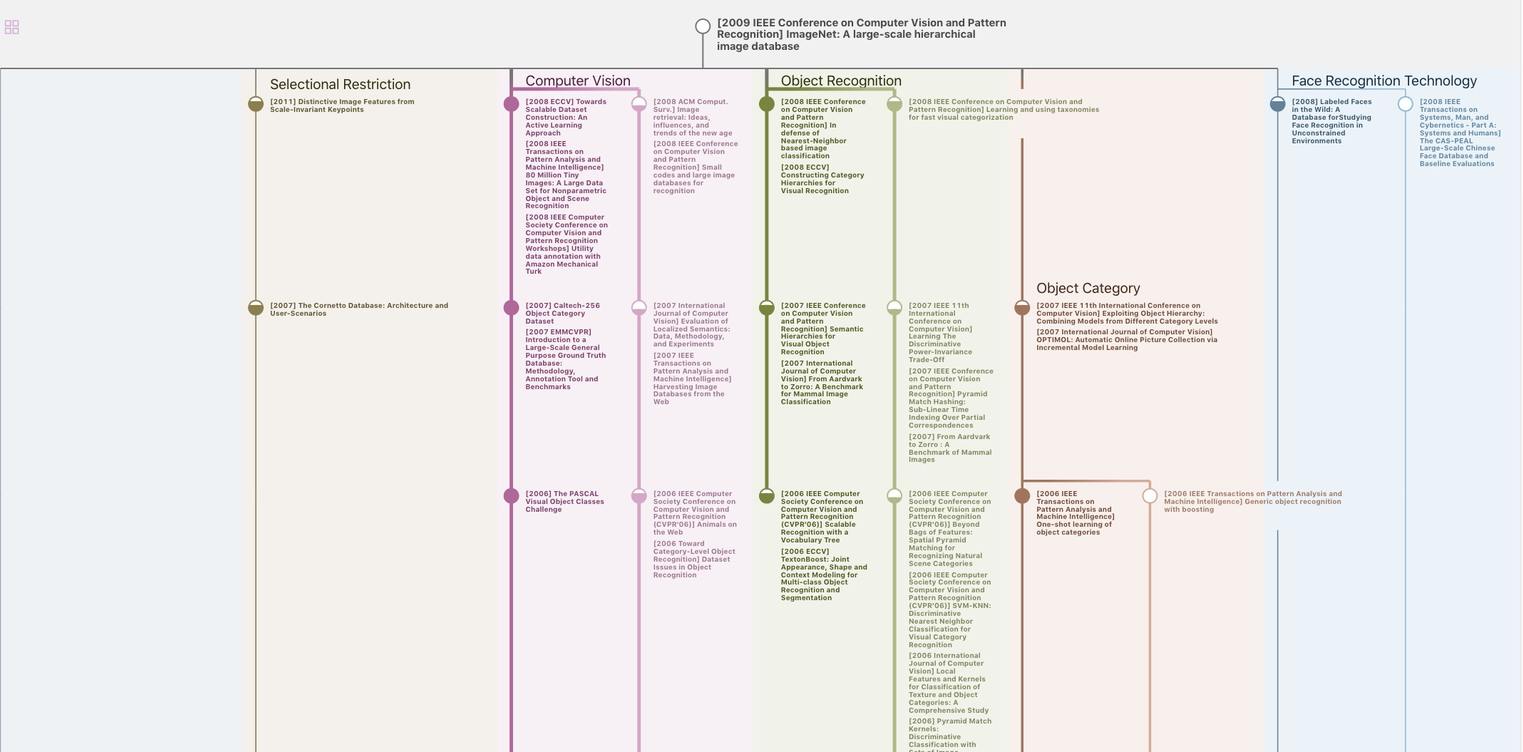
生成溯源树,研究论文发展脉络
Chat Paper
正在生成论文摘要