A Reinforcement Learning Method For Humanoid Robot Walking
2018 IEEE 8TH ANNUAL INTERNATIONAL CONFERENCE ON CYBER TECHNOLOGY IN AUTOMATION, CONTROL, AND INTELLIGENT SYSTEMS (IEEE-CYBER)(2018)
摘要
In this paper, we describe a model-free reinforcement learning method for gait controlling of humanoid robots, which combines Q-learning with Radial Basis Function Network. With the help of RBF Network, this method can solve the approximation problem caused by continuous state space and action space. The approach is applied to the controllers on hip joints of humanoid robots that receives sensory data and constantly adjusts the outputs of steering engines on hip joints, finding an optimal policy that can guide humanoid robots to walk stably on different uneven terrains. We have tested the approach on Webots, a simulation platform, and experiment results have proven the validity of the proposed method.
更多查看译文
关键词
humanoid robot, reinforcement learning, gait planning, radial basis function network
AI 理解论文
溯源树
样例
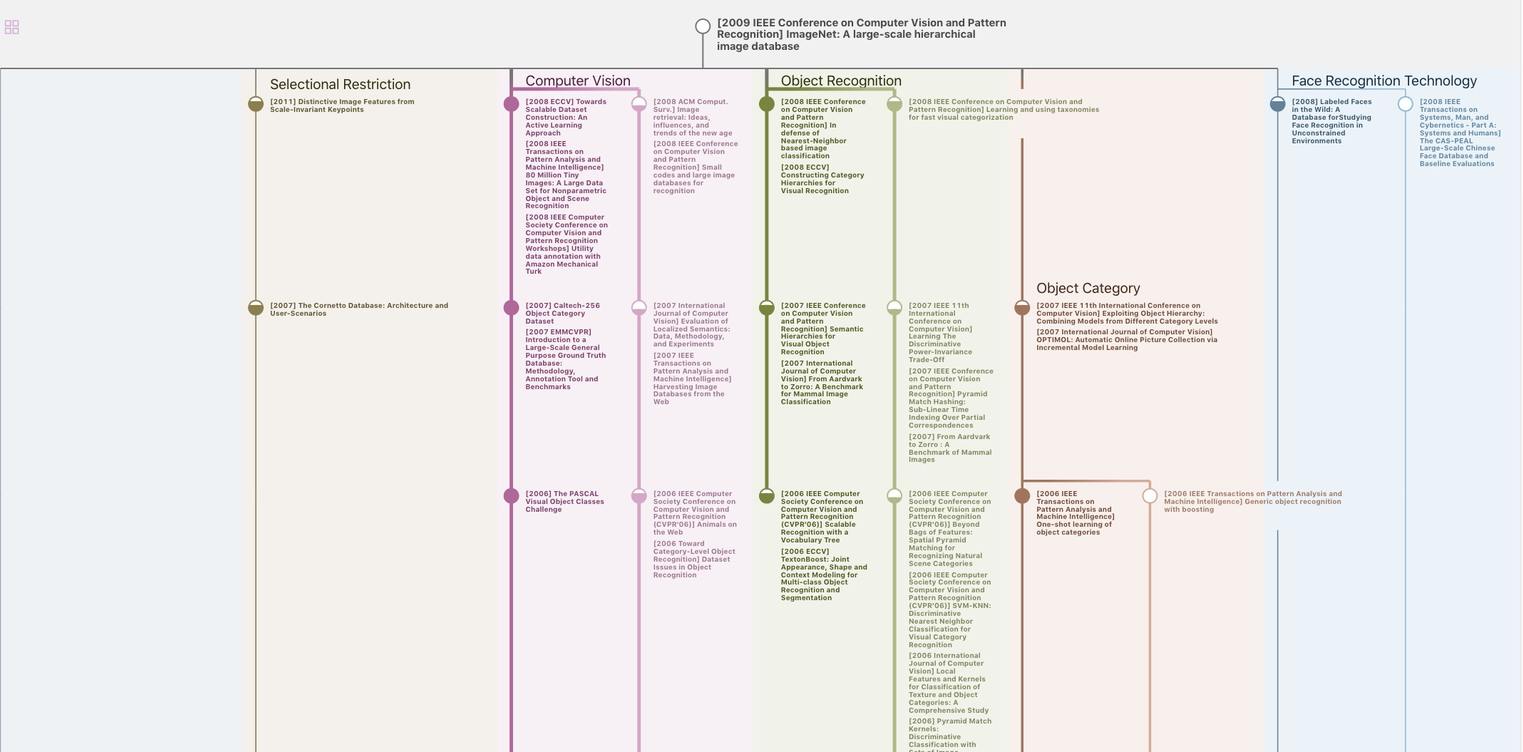
生成溯源树,研究论文发展脉络
Chat Paper
正在生成论文摘要