Cola: Communication-Censored Linearized Admm For Decentralized Consensus Optimization
2019 IEEE INTERNATIONAL CONFERENCE ON ACOUSTICS, SPEECH AND SIGNAL PROCESSING (ICASSP)(2019)
摘要
This paper proposes a communication- and computation-efficient algorithm to solve a convex consensus optimization problem defined over a decentralized network. A remarkable existing algorithm to solve this problem is the alternating direction method of multipliers (ADMM), in which at every iteration every node updates its local variable through combining neighboring variables and solving an optimization subproblem. The proposed algorithm, called as communication-censored linearized ADMM (COLA), leverages a linearization technique to reduce the iteration-wise computation cost of ADMM and uses a communication-censoring strategy to alleviate the communication cost. To be specific, COLA introduces successive linearization approximations to the local cost functions such that the resultant computation is first-order and light-weight. Since the linearization technique slows down the convergence speed, COLA further adopts the communication-censoring strategy to avoid transmissions of less informative messages. A node is allowed to transmit only if the distance between the current local variable and its previously transmitted one is larger than a censoring threshold. We establish convergence as well as sublinear and linear rates of convergence of COLA, and demonstrate its satisfactory communication-computation tradeoff with numerical experiments.
更多查看译文
关键词
Decentralized consensus optimization, alternating direction method of multipliers, communication censoring
AI 理解论文
溯源树
样例
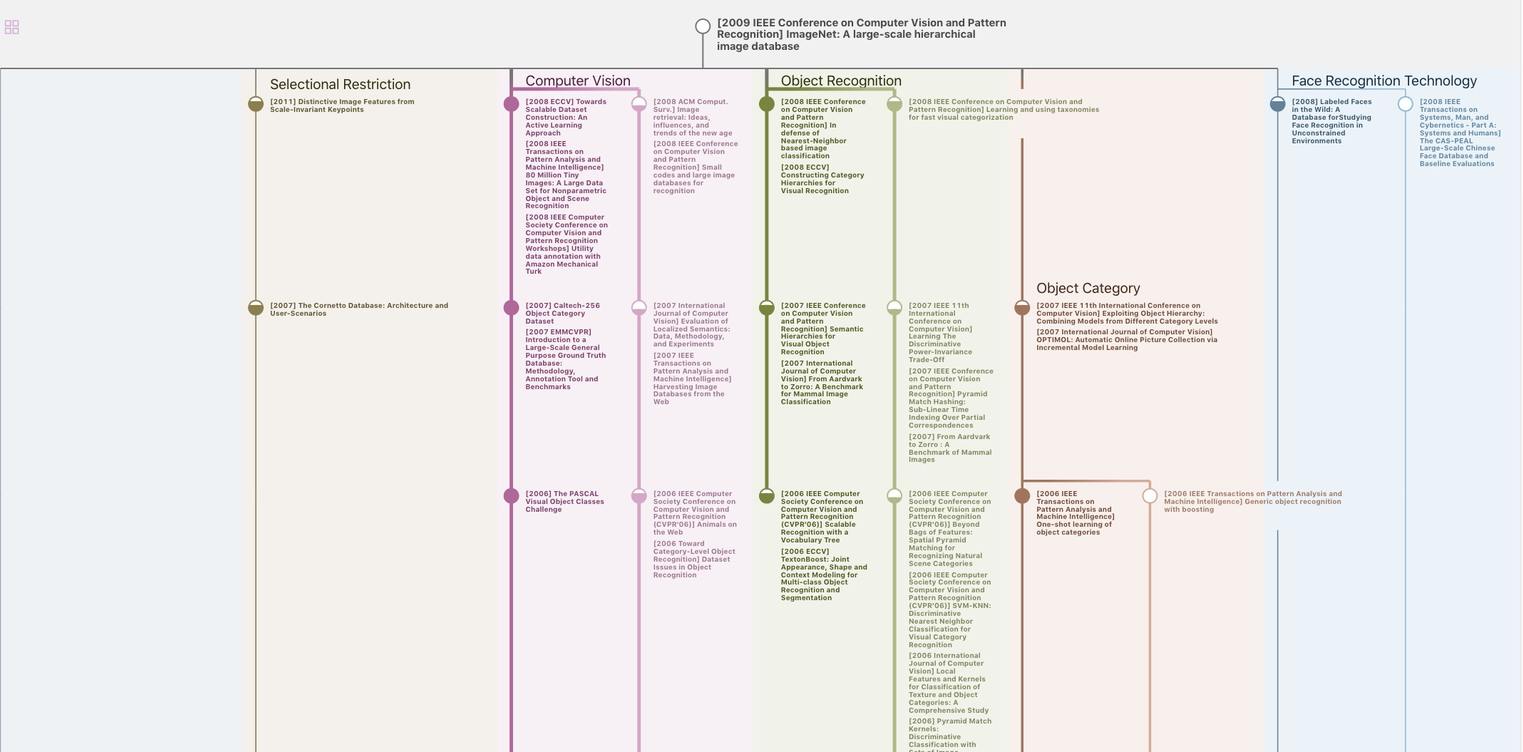
生成溯源树,研究论文发展脉络
Chat Paper
正在生成论文摘要