Boosting Exponential Gradient Strategy for Online Portfolio Selection: An Aggregating Experts’ Advice Method
Computational Economics(2019)
摘要
Online portfolio selection is one of the fundamental problems in the field of computational finance. Although existing online portfolio strategies have been shown to achieve good performance, we always have to set the values for different parameters of online portfolio strategies, where the optimal values can only be known in hindsight. To tackle the limits of existing strategies, we present a new online portfolio strategy based on the online learning character of Weak Aggregating Algorithm (WAA). Firstly, we consider a number of Exponential Gradient (EG (η ) ) strategies of different values of parameter η as experts, and then determine the next portfolio by using the WAA to aggregate the experts’ advice. Furthermore, we theoretically prove that our strategy asymptotically achieves the same increasing rate as the best EG (η ) expert. We prove our strategy, as EG (η ) strategies, is universal. We present numerical analysis by using actual stock data from the American and Chinese markets, and the results show that it has good performance.
更多查看译文
关键词
Online portfolio selection, Universal portfolio, Online expert advice, Weak aggregating algorithm
AI 理解论文
溯源树
样例
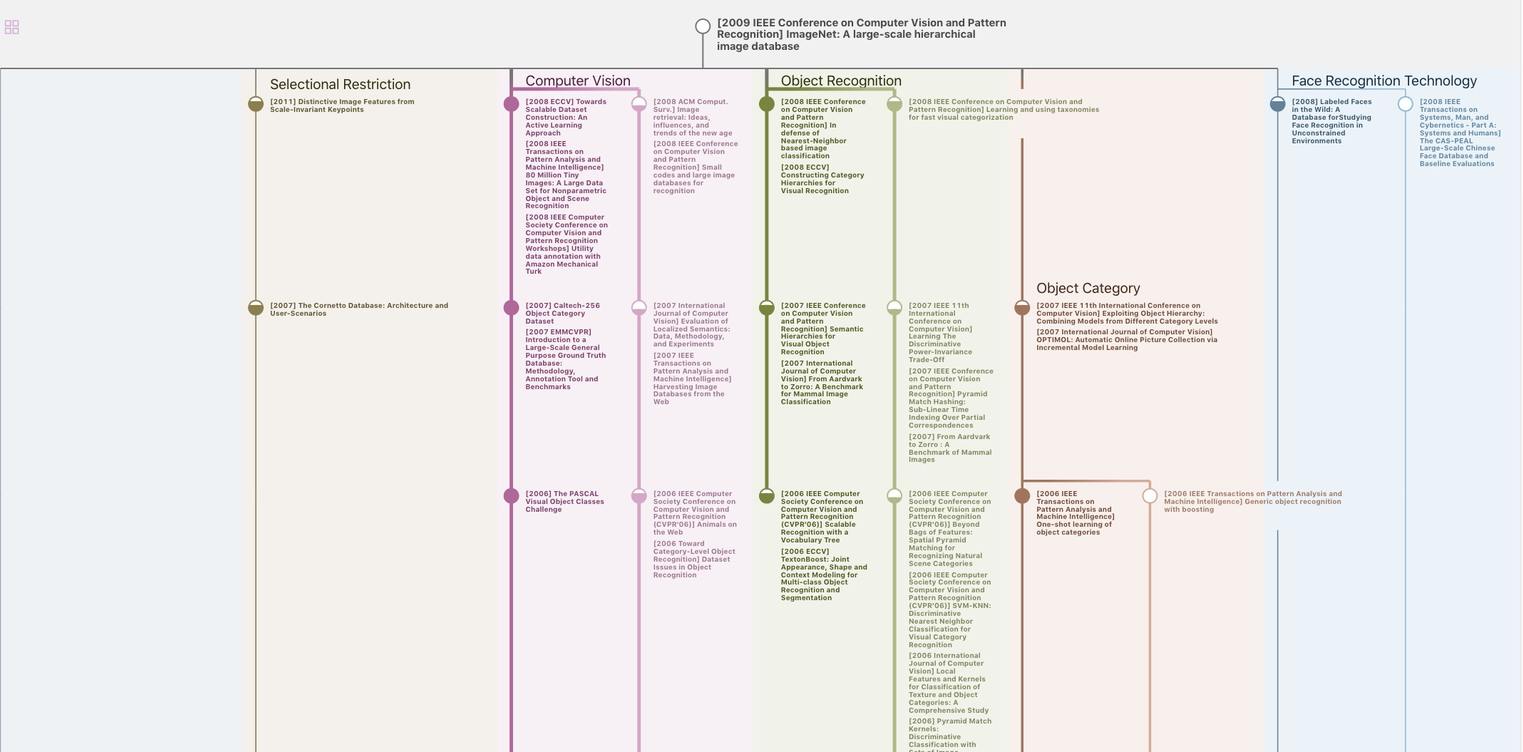
生成溯源树,研究论文发展脉络
Chat Paper
正在生成论文摘要