Evaluation of Deep Learning Neural Networks for Surface Roughness Prediction Using Vibration Signal Analysis
APPLIED SCIENCES-BASEL(2019)
摘要
The use of surface roughness (Ra) to indicate product quality in the milling process in an intelligent monitoring system applied in-process has been developing. From the considerations of convenient installation and cost-effectiveness, accelerator vibration signals combined with deep learning predictive models for predicting surface roughness is a potential tool. In this paper, three models, namely, Fast Fourier Transform-Deep Neural Networks (FFT-DNN), Fast Fourier Transform Long Short Term Memory Network (FFT-LSTM), and one-dimensional convolutional neural network (1-D CNN), are used to explore the training and prediction performances. Feature extraction plays an important role in the training and predicting results. FFT and the one-dimensional convolution filter, known as 1-D CNN, are employed to extract vibration signals' raw data. The results show the following: (1) the LSTM model presents the temporal modeling ability to achieve a good performance at higher Ra value and (2) 1-D CNN, which is better at extracting features, exhibits highly accurate prediction performance at lower Ra ranges. Based on the results, vibration signals combined with a deep learning predictive model could be applied to predict the surface roughness in the milling process. Based on this experimental study, the use of prediction of the surface roughness via vibration signals using FFT-LSTM or 1-D CNN is recommended to develop an intelligent system.
更多查看译文
关键词
surface roughness,vibration signals,convolution neural network,CNN,Fast Fourier Transform,FFT,long short term memory network,LSTM,deep neural networks,DNN,deep learning
AI 理解论文
溯源树
样例
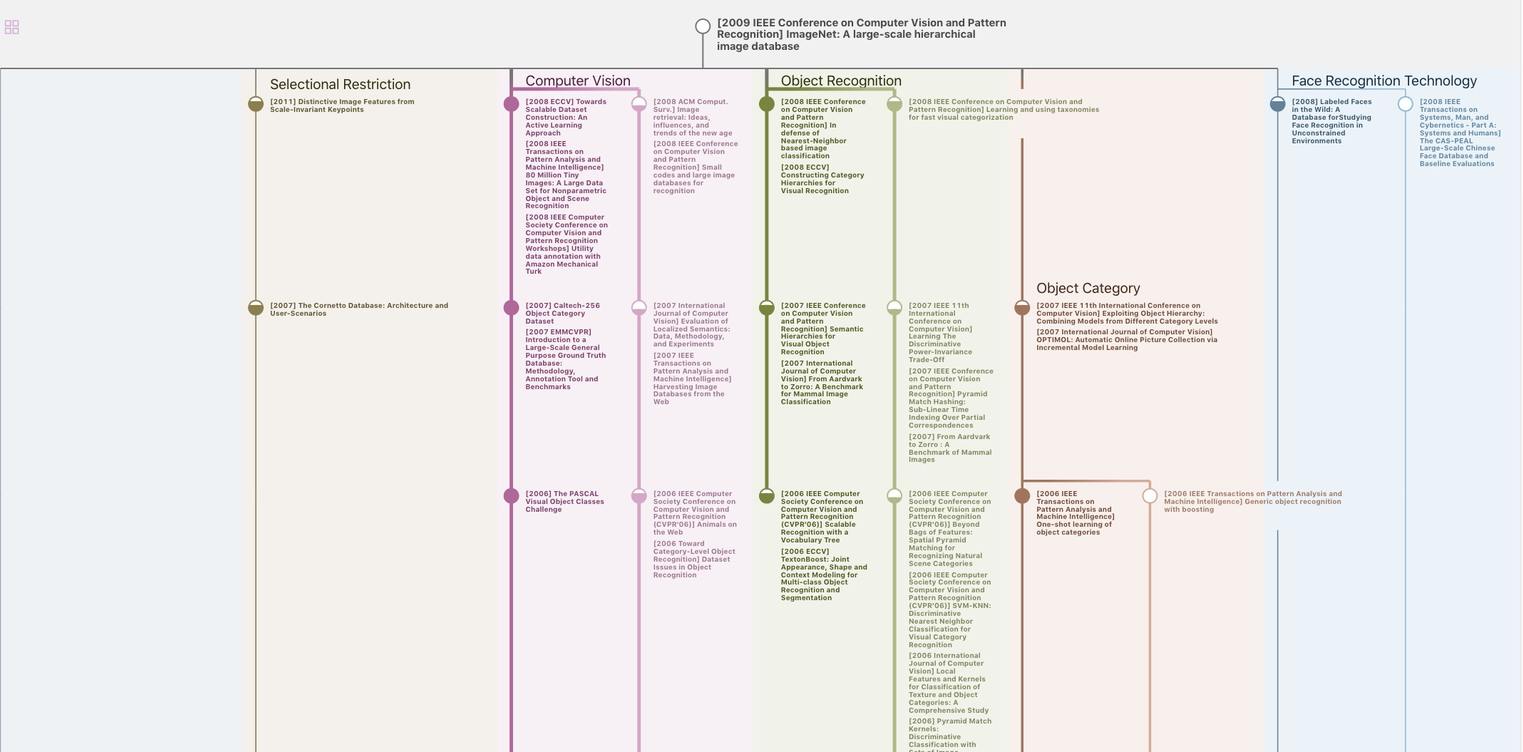
生成溯源树,研究论文发展脉络
Chat Paper
正在生成论文摘要