Deep Counting Model Extensions With Segmentation For Person Detection
2019 IEEE INTERNATIONAL CONFERENCE ON ACOUSTICS, SPEECH AND SIGNAL PROCESSING (ICASSP)(2019)
摘要
Applications like autonomous driving, surveillance, or any application that demands scene analysis requires object detection, semantic segmentation and instance segmentation. In this paper, we focus on the problem of detecting each instance of a specific category of objects, specifically persons. A novel method for object detection is proposed based on a deep counting model. The feature extractor of the deep counting model is extended with additional layers for segmenting specific instances. While the feature extractor of the deep counting model already focuses on the persons in the scene, the segmentation layers help to get a more accurate estimation of the foreground with persons and the instance segmentation is able to estimate separate instances of persons. Our proposed method outperforms other methods on the CUHK08 dataset with an Average Miss Rate (AMR) of 14% and on the PETS09 dataset with an AMR of 41%.
更多查看译文
关键词
Deep convolutional neural networks, deep counting models, detection, segmentation, multi-task network
AI 理解论文
溯源树
样例
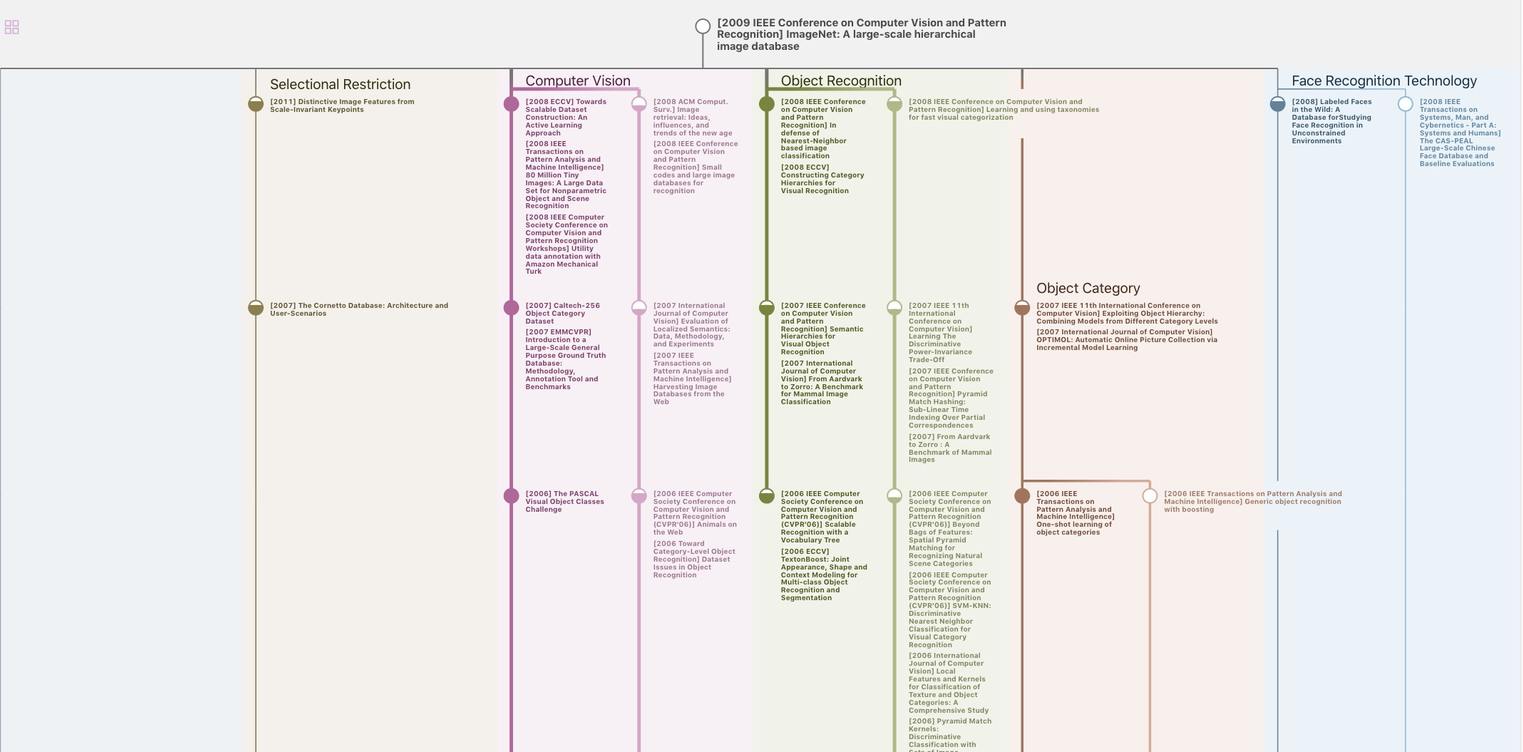
生成溯源树,研究论文发展脉络
Chat Paper
正在生成论文摘要