Uncertain Interval Data EFCM-ID Clustering Algorithm Based on Machine Learning
JOURNAL OF ROBOTICS AND MECHATRONICS(2019)
摘要
In clustering problems based on fuzzy c-means (FCM) for uncertain interval data, points within the interval are usually assumed to have uniform distribution, resulting in the difficulty of accurately describing the interval. Furthermore, the clustering results are considerably affected by the initial clustering centers, and the update speed of the membership degree is slow. To address these problems, a new clustering algorithm called uncertain FCM for interval data (EFCM-ID) is presented. On the basis of a quartile, a median quartile-spacing distance measurement for generally distributed interval data based on machine learning is designed to precisely determine these data. Simultaneously, we sample the whole dataset and consider the density centers as the initial clustering centers to increase accuracy. We call this method sampling based density-center selection (SDCS). To reduce the running time, a new measurement based on competitive-learning theory to update the membership is developed. It accelerates the update speed by different degrees according to value of the membership degree. Experiments conducted on synthetic interval datasets show the feasibility of EFCM-ID.
更多查看译文
关键词
uncertain cluster,interval data,FCM,machine learning,density based
AI 理解论文
溯源树
样例
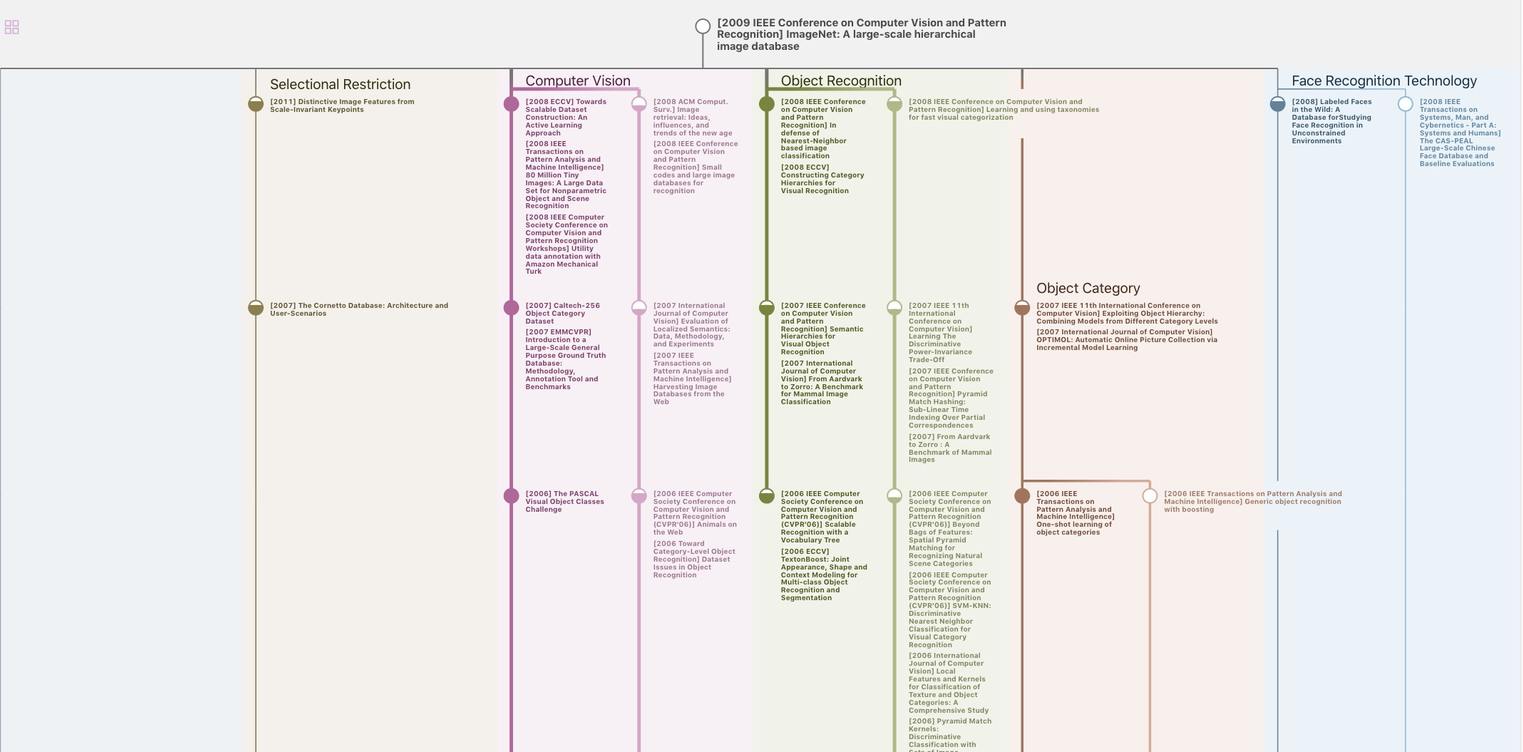
生成溯源树,研究论文发展脉络
Chat Paper
正在生成论文摘要