Minimizing the total weighted tardiness of overlapping jobs on parallel machines with a learning effect
JOURNAL OF THE OPERATIONAL RESEARCH SOCIETY(2020)
摘要
The influence of learning effects on job scheduling has been studied for years. By considering learning effects, an operator can schedule jobs in a more precise way and improve on the original schedules. In fact, jobs with duplicate contents (i.e., overlapping jobs) do not require as much processing time as do disjoint jobs. However, this phenomenon is seldom discussed in traditional scheduling models. In this study, a parallel-machine scheduling problem with a learning effect and an overlap effect is introduced. The objective is to minimise the total weighted tardiness of jobs whose processing times are influenced by both effects. A branch-and-bound algorithm comprising a lower bound algorithm is developed to generate the optimal schedules. Compared with past research, two main contributions are made. First, a model considering simultaneously both the learning effect and the overlap effect is proposed. Second, an efficient lower bound algorithm accelerating the execution speed is developed. At the end, computational experiments are conducted to show the execution efficiency and cost effectiveness.
更多查看译文
关键词
Job scheduling,minimization of total tardiness,learning effect,overlap effect,sensitivity analysis,parallel-machine scheduling
AI 理解论文
溯源树
样例
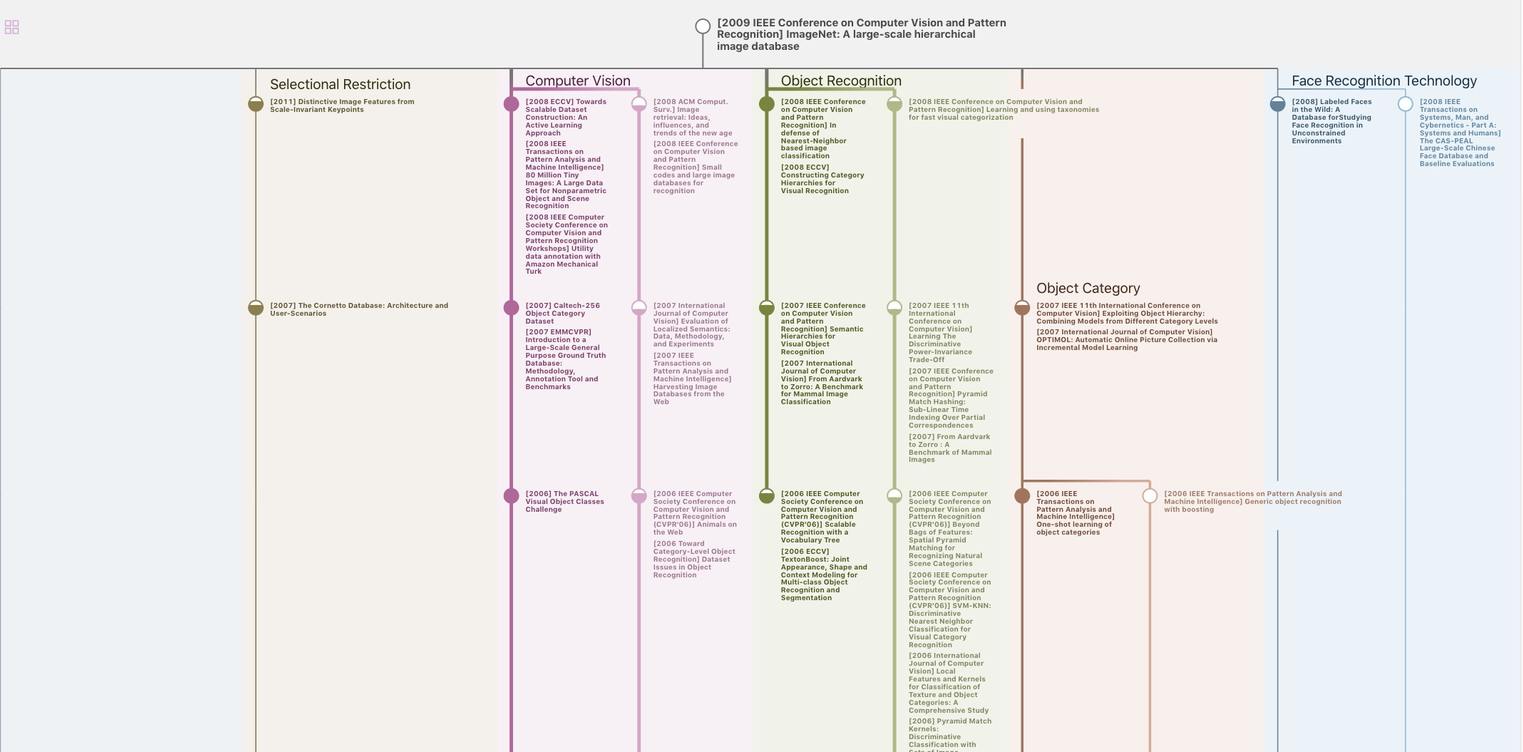
生成溯源树,研究论文发展脉络
Chat Paper
正在生成论文摘要