A comprehensive study in assembling deep convolutional neural networks for image classification
Proceedings of SPIE(2019)
摘要
Ensemble methods have been broadly used in many applications to integrate individual models for achieving better performance like accuracy and robustness. This paper focuses on using deep Convolutional Neural Networks (CNNs) models and ensembling them for image classification. To have a comprehensive and comparative study, five ensemble techniques are explored and their combinations were applied in a two-stage ensemble process. In detail, multiple basic CNN models (structures) are first pre-defined to increase model variety for the ensemble, from which each basic CNN model is trained multiple rounds based on sub-sampling on the training dataset. In the first stage of ensemble, multiple predictions (through sub-sampling) from each basic CNN structure are combined. This is followed by the second stage of ensemble to integrate the outputs from all basic CNN structures. Experiments were conducted using Kaggle's 'Statoil/C-CORE Iceberg Classifier Challenge' image data for iceberg and ship classification. The experimental results showed that the ensembling CNN models could improve classification accuracy in the image classification problem.
更多查看译文
关键词
Ensemble,Convolutional Neural Networks (CNNs),image classification,object recognition
AI 理解论文
溯源树
样例
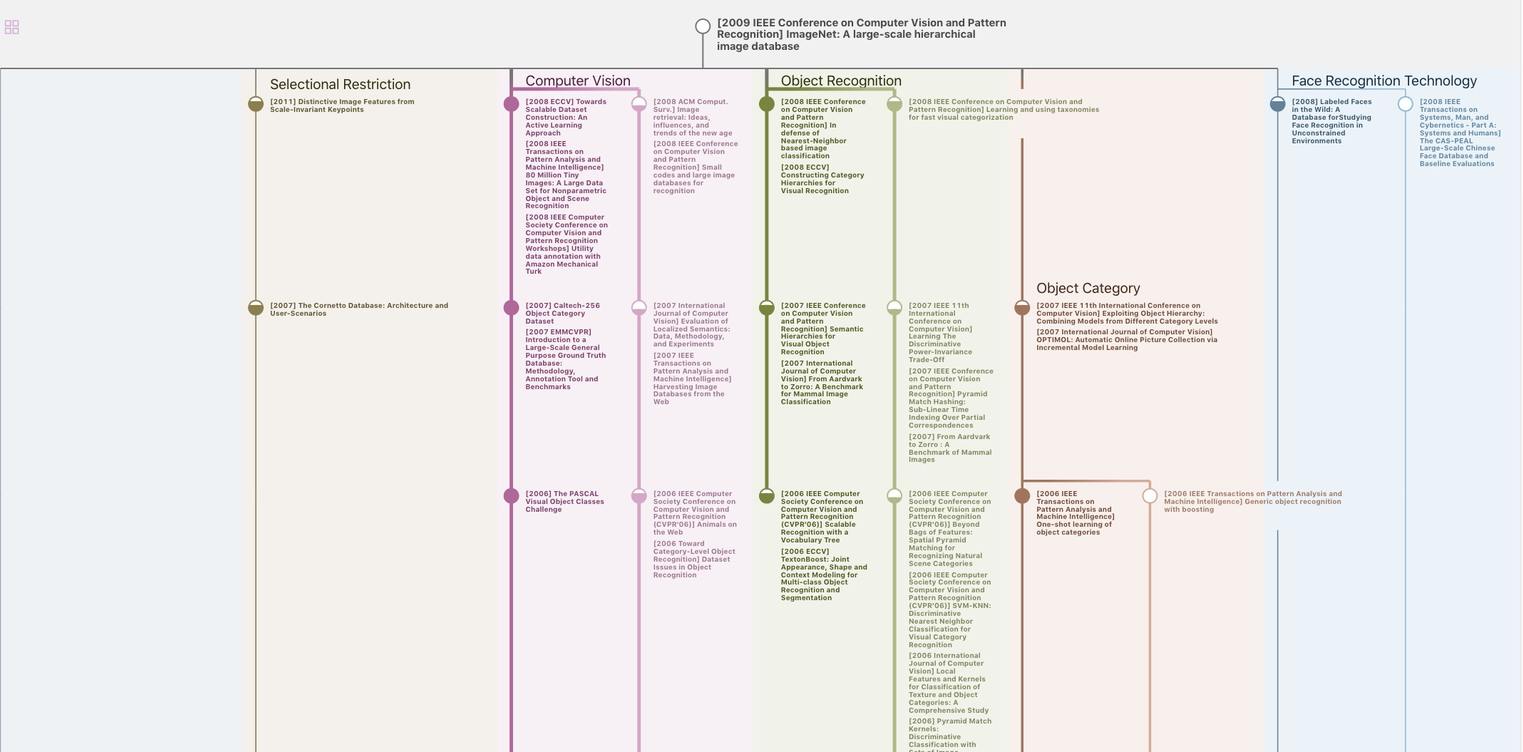
生成溯源树,研究论文发展脉络
Chat Paper
正在生成论文摘要